350 rub
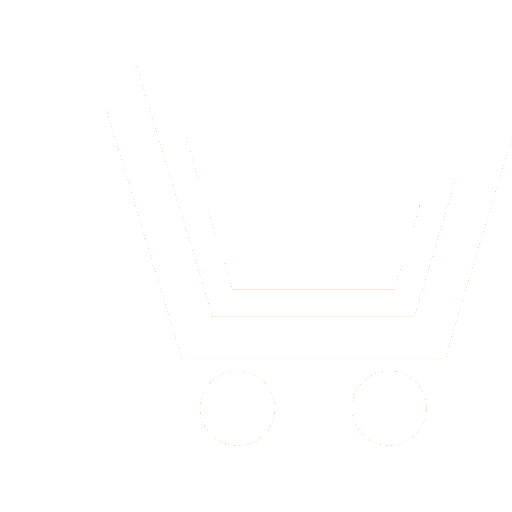
Journal Biomedical Radioelectronics №6 for 2009 г.
Article in number:
Automatic Classification Electroencephalogram on the Basis of their Wavelet-Batch Operation
Keywords:
wavelet-packages
electroencephalographically signals
automatic classification
the coding of signs
Authors:
N.T. Abdullayev, O.A. Dishin, Kh.Z. Samedova
Abstract:
By means of wavelet-batch operation EEG of signals of its organization in time and space which find the reflection in frequency of the basic rhythms to their regularity, amplitude and zone distinction on areas of a brain are defined. On frequencies it is convenient to represent distribution of rhythms in the form of substrip coding with reduction of a strip of frequencies at each level twice. According to E.A. Jurmunskaya EEG syndromic classification - signals break into 17 groups to six signs. In the received code the place occupied in figure, designates sign number, and value of figure designates number of gradation of this sign.
Wavelet-batch factors in each frequency substrip allow to establish an accessory of a pattern of data EEG to this or that group EEG on classification E.A. Jirmunskaya, and also to define moment of occurrence of pathology and dynamics of its development.
These factors are calculated by means of package wavelet - the algorithms which have been built in in system of computer mathematics Wavelet Toolbox MATLAB-6.
Pages: 63-68
References
- Жирмунская Е.А., Лосев В.С. Системы описания и классификация электроэнцефалограмм человека. М.: Наука. 1984. 79 с.
- Жирмунская Е.А. Клиническая электроэнцефалография (Обзор литературы и перспектива использования метода). М.: Изд-во «Мейби». 77 с.
- Сахаров В.Л. Андреенко А.С. Методы математической обработки электроэнцефалограмм. Таганрог: Изд-во «Антон». 2000. 44 с.
- Чуи К. Введение в вейвлеты / пер. с англ. М.: Мир. 2001. 412 с.
- Добеши И. Десять лекций по вейвлетам / пер. с англ. М.-Ижевск: НИЦ «Регулярная и хаотическая динамика». 2004. 463 с.
- Meyer Y., Coifman R .R., Wickerhauser M.V. Size properties of wavelet packets. In Ruskai etal, editor. Wavelets and their Applications. Jones and Bartlett. 1992.
- Яковлев А.Н. Основы вейвлет-преобразования сигналов. Учебное пособие. М.: САЙНC-ПРЕСС. 2003. 80 с.
- Бендат Дж., Пирсол А. Применение корреляционного и электронного анализа / пер с англ. М.: Мир. 1983. 312 с.
- Жуковский В.Д. Автоматизированная обработка данных клинических функциональных исследований. М.: Медицина. 1981. 352 с.
- Жирмунская Е.А., Дубнер П.П., Гутман С.Р. Перспективы применения моделей типа авторегрессии - скользящего среднего для анализа ЭЭГ // Успехи физиологических наук. 1984. Т. 69. № 4.
- Жариков И.О. Статистический анализ информационных сигналов от датчиков медицинских приборов (электроэнцефалография) // Датчики и Системы. 2003. № 7. С. 23 - 29.
- Ширяев А.Н. Статистический последовательный анализ. Оптимальные правила остановки. М.: Наука. 1976. 231 с.
- Никифоров И.В. Последовательное обнаружение изменения свойств временных рядов. М.: Наука. 1983. 199 с.
- Mallat S. A theory for multiscale signal decomposition. The wavelet representation // Trans. Pattern Analysis and Machine Intelligence. 1989. July. V.11. P. 674 - 693.