350 rub
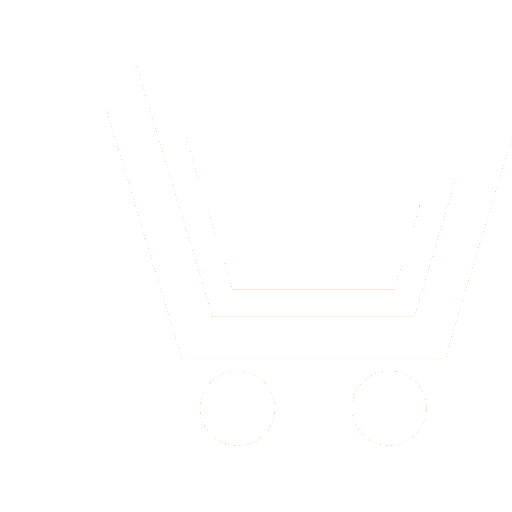
Journal Biomedical Radioelectronics №5 for 2009 г.
Article in number:
Hybrid Method for Classification of Biosignals Based on Fuzzy Logic Decisions and Neural Networks Technology
Authors:
V.V. Zhilin, S.A Filist, Al?-Muaalemi Vail Abdulkarim
Abstract:
In this work we analyzed the possibility of applying fuzzy logic technology decision-making, and neural networks for solving classification biosignalov. A comparative analysis of characteristics of the use of different methods for classifying data sets with non-standard topology of the distribution of informative features. The following methods of separation of classes:
use of a neural network trained on the basis of baseline data;
construction of a fuzzy logical conclusion based on expert evaluation of the space characteristics.
For consideration in this article are also presented developed a hybrid method of classifying biosignalov combining the advantages of the above methods. The hybrid method of division into classes is to use neural network as a system of fuzzy defuzzifikatora output. Comparative analysis is performed using the corresponding software module, developed in the Matlab 7.1. In this paper demonstrate the main features of the module for modeling a hybrid system of decision-making, to apply the specified method in determining the classification of biomedical problems. This module allows you to build and train a neural network, the choice of the type and parameters of membership functions for each feature class, the synthesis of fuzzy aggregator system, ie a set of fuzzy operations to convert the values of functions belonging signs of the coefficients of confidence classes. Experimental studies on the classification of the test data set showed that using four-levels neural network classification accuracy was 82%. The distribution of class-based fuzzy system allowed for the accuracy of 88%. When using the hybrid classification algorithm achieved accuracy was 96%, which confirmed the validity of applying the algorithm to solve the problems of classification.
Pages: 77-82
References
- Ярушкина Н.Г. Нечеткие гибридные системы. Теория и практика. М.: Физматлит. 2007. 208 с.
- Ярушкина Н.Г. Нечеткие нейронные сети с генетической настройкой. Лекция научной школы «Нейроинформатика-2005». М.: МИФИ. 2005. 214 с.
- Леоненков А. В. Нечеткое моделирование в среде Matlab и fuzzyTech. СПб.: БХВ-Петербург. 2005. 736 с.
- Дьяконов В.П., Круглов В.В. MATLAB 6.5 SP1/7/7 SP1/7 SP2 + Simulink 5/6. Инструменты искусственного интеллекта и биоинформатики. Сер. «Библиотека профессионала». М.: СОЛОН-ПРЕСС. 2006. 456 с.
- Грахов А.А., Жилин В.В., Филист С.А. Модель модуля нечеткого вывода для ранней диагностики ишемической болезни сердца. VIII междунар. научно-техн. конф. «Физика и радиоэлектроника в медицине и экологии». Владимир. 2008. 4 с.