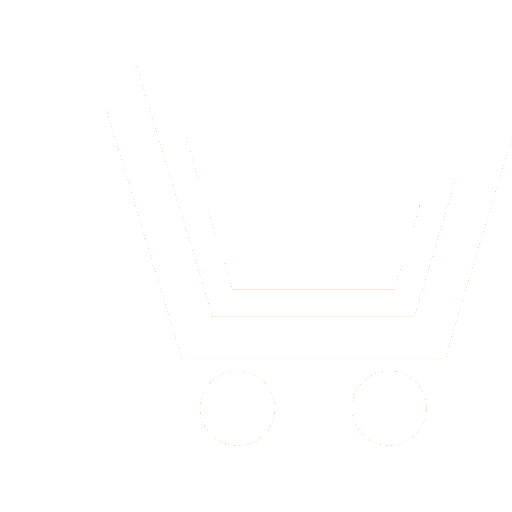
I.Yu. Bratukhin1, G.M. Revunov2
1,2 St. Petersburg State University of Aerospace Instrumentation (St. Petersburg, Russia)
1 bratukhinIY@signal-spb.ru, 2 revunpank@gmail.com
When processing measurement results in scientific or industrial studies of the properties of objects, the averaged characteristics of these properties are most often found, in particular their average values and scatter parameters. However, when monitoring product parameters, the central characteristic of observations, the average value, is not the best assessment of the result of the control measurement. That is, there is a difference between processing results when studying object parameters and when monitoring parameters for compliance with acceptable values. Therefore, it is necessary to consider a method for assessing the uncertainty of an extreme (minimum or maximum) observation, which is based on the properties of order statistics and allows one to calculate the standard and expanded uncertainty of extreme observations.
Even with a normal distribution of observations, the dependence of the most extreme (minimum or maximum) observations depends on the sampling parameters. Since such control measurements are mainly associated with the destruction of products, the number of available observations is significantly limited, therefore, when assessing the uncertainty of extreme values, it is impossible to apply their limiting distributions. To assess the uncertainty of measurement results, the Monte–Carlo method is considered.
The emergence of statistical modeling methods (Monte–Carlo) in various areas of applied mathematics is associated with the need to solve qualitatively new problems arising from the needs of practice. The direct statistical modeling method is the most common among numerical methods for solving applied problems when conducting experimental studies that depend on the sample size. Analysis of the results obtained by Monte–Carlo simulation showed that for various types of distributions, the parameters describing the normalized deviation of the minimum observation are quite close to each other. This can be used to estimate the expanded uncertainty of a minimum (maximum) observation in the absence of information about the distribution of the observations themselves.
The proposed methodology can be used to assess the uncertainty of results when testing tensile, tensile or perpendicularity, flatness of other mechanical or geometric properties of functional materials parameters in the production process.
Bratukhin I.Yu., Revunov G.M. Processing results of experimental research and simulation using the Monte–Carlo method. Achievements of modern radioelectronics. 2024. V. 78. № 1. P. 95–104. DOI: https://doi.org/10.18127/j20700784-202401-09 [in Russian]
- Petrushin V.N, Ul'yanov M.V. Informatsionnaya chuvstvitel'nost' komp'yuternykh algoritmov. M.: Fizmatlit. 2010. [in Russian]
- Kosobutskiy P. Statisticheskie i Monte-Karlo algoritmy modelirovaniya sluchaynykh protsessov v makro- i mikrosistemakh v MathCAD. L'vov: Izd-vo Natsional'nogo universiteta «L'vovskaya politekhnika». 2014. [in Russian]
- Evaluation of measurement data – Supplement 1 to the «Guide to the Expression of Uncertainty in Measurement» – Propagation of distributions using a Monte-Carlo method. BIPM, IEC, IFCC, ILAC, ISO, IUPAC, IUPAP and OIML.JCGM 101:2008.p.82.
- Khofman D. Tekhnika izmereniy i obespecheniya kachestva: Spravochnaya kniga. Per. s nem. M.: Energoatomizdat. 1983. [in Russian]
- Novitskiy P.V., Zograf I.A. Otsenka pogreshnostey rezul'tatov izmereniy. Monografiya. L.: Energoatomizdat. 1991. [in Russian]
- Dorozhovets M., Bubela I. Computing uncertainty of the extreme values in random samples. International Journal of Computing. 2016. V. 15 (2). P. 127–135.
- Linnik Yu.V. Razlozheniya veroyatnostnykh zakonov. L.: Izd-vo LGU. 1960. [in Russian]
- Gmurman V. Teoriya veroyatnostey i matematicheskaya statistika. M.: Vyssh. shkola. 2003. [in Russian]
- Bratukhin I.Yu., Kryachko A.F. Metodika priblizhennogo resheniya zadach modelirovaniya fizicheskikh kharakteristik radiopogloshchayushchikh materialov. Uspekhi sovremennoy radioelektroniki. 2022. T. 76. № 12. S. 29–36. DOI: https://doi.org/10.18127/j20700784-202212-05. [in Russian]
- Bratukhin I.Yu., Kryachko A.F., Shakin O.V. Metod sinteza mikrourovnevykh struktur radiopogloshchayushchikh materialov. Uspekhi sovremennoy radioelektroniki. 2022. T. 76. № 12. S. 37–45. DOI: https://doi.org/10.18127/j20700784-202212-06. [in Russian]