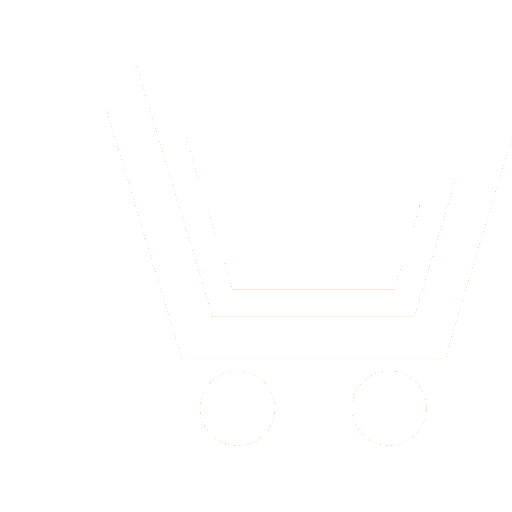
V.Yu. Leshchinskiy – Leading Engineer,
JSC NPO «NIIIP-NziK» (Novosibirsk)
E-mail: wdm@ngs.ru
I.F. Lozovskiy – Ph.D. (Eng.), Senior Research Scientist, Head of Sector,
JSC NPO «NIIIP-NziK» (Novosibirsk) E-mail: lozov igor@yandex.ru
The rapid development of unmanned systems has led to the fact that traditional radar systems were unable to effectively detect them and measure their flight parameters. This led to the need to increase the resolution of the radar survey. One way to achieve this is to use broadband sounding signals. In addition, the use of broadband signals, in the long run, allows us to solve the problem of target recognition by the characteristic distribution of brilliant points. The broadband signal reflected from the target is a set of reflections randomly distributed on the basis of the signal from the «shiny points» of the target, the number of which depends on the size of the target, angle and band of the signal. In this paper, it is assumed that the number of such points is not less than three and does not exceed 41 (the maximum is determined by the signal band).
In order for the radar to be able to efficiently detect such signals reflected from an arbitrary target, while maintaining a constant level of false alarms, the existing CMS systems are not suitable and it is necessary to develop new algorithms for solving this problem. As one of the approaches to solving this problem, we can propose a multi-channel system, each channel of which is «tuned» to a specific signal length. Each channel has an interference power evaluator and an incoherent drive. The accumulation results are normalized to power and compared with an individual threshold selected based on the desired level of false alarms. Then all the channels are combined by «OR».
Such a system provides stabilization of false alarms over the entire range of interference power.
In this paper, we propose an algorithm for detecting broadband signals with a constant level of false alarms based on artificial neural networks. The algorithm is constructed using convolutional neural networks. The volume of the training sample was 10,000 elements, each of which could be either just noise with a Rayleigh distribution or a mixture of a signal of a certain duration and noise. The signal-to-noise ratio was selected from a certain range. The training was carried out by the method of back propagation of error. After training, the obtained algorithm was tested using a newly generated sample. The decision threshold was selected using the ROC (receiver operating characteristic) curve based on the required probability of false alarm. This threshold was common to all possible signal lengths. After that, the algorithm was validated by constructing the detection characteristics, determining threshold signals for various signal lengths, and building the dependence of the level of false alarms depending on the interference power. Comparison of the results with a multichannel algorithm based on incoherent accumulation showed that the difference in threshold signals does not exceed 0,5 dB, while algorithms based on neural networks do not require an individual calculation of thresholds for each channel. The algorithm proposed in this paper is designed to work in noise conditions with a Rayleigh distribution of the envelope, but in the future it is supposed to generalize it to work in conditions of interference with another distribution.
- Sekine M., Mao Y. Weibull Radar Clutter. London. U.K. IEEPress. 1990.
- Hansen V.G. Generalised constant false alarm rate processing and an application to the Weibull distribution. 1972 International Symposium on Information Theory, Asilomar, CaL. USA.
- Schleher D.C. Radar detection in Weibull clutter. IEEE Trans. On AES. 1976. № 12 (6). P. 736–743.
- Schleher D.C. Radar detection in Log-normal clutter. Proc.of the IEEE International Radar Coference. Washintong. 1975. P. 262–267.
- Glubokoe obuchenie. Pogruzhenie v mig glubokih setej. [in Russian]
- Finn H.M., Johnson K.S. Adaptive Detection Mode with Threshold Control as a Function of Spatially Sampled Clutter - Level Estimates. RCA Review. Sept. 1968. V. 29. № 3. P. 414–464.