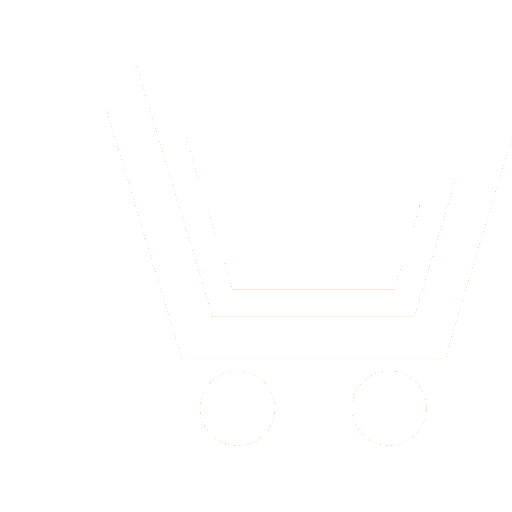
В.И. Терехов1, Д.О. Ишков2
1,2 Московский государственный технический университет им. Н.Э. Баумана (Москва, Россия)
Постановка проблемы. Большинство существующих работ исследует распознавание CAPTCHA фиксированной длины, однако предложено использовать дистилляцию знаний для имитации работы рекуррентно-сверточных моделей, которые хорошо зарекомендовали себя в задаче предсказания динамической длины символов на изображениях. Стремительное развитие систем глубокого обучения, качество распознавания которых достигло уровня зрения человека, делает все более неэффективным способ защиты с помощью CAPTCHA. Кроме того, такая защита предъявляет высокие требования к характеристикам устройств, на которых проводится распознавание. Проведенные в работе исследования позволили предложить эффективный метод обучения CNN на неточных данных для автоматического обхода текстовых CAPTCHA на мобильных устройствах. Цель. Разработать метод обучения легковесных сверточных нейросетей без рекуррентных слоев для распознавания текстовой CAPTCHA различной длины с применением дистилляции знаний. Получить легковесную модель высококачественного распознавания текстовой CAPTCHA, способной работать на мобильных устройствах.
Результаты. Описан метод обучения легковесной модели на неточной разметке, полученной от другой модели. Изучено влияние размера обучающей выборки на качество распознавания, скорость работы модели на различных конечных устройствах на примере популярной социальной сети.
Практическая значимость. Предложенный метод позволяет обучать сверточные модели для обхода защиты веб-сайтов – текстовой CAPTCHA, которые нетребовательны к характеристикам устройств. Произведенный анализ ошибок модели позволяет составить рекомендации по улучшению способов противодействия автоматическому распознаванию.
Терехов В.И., Ишков Д.О. Дистилляция знаний моделей сверточных нейронных сетей на неточных данных для автоматического обхода текстовых CAPTCHA на мобильных устройствах // Динамика сложных систем. 2021. T. 15. № 3. С. 5−19. DOI: 10.18127/j19997493-202103-01
- Klopfenstein L.C. et al. The rise of bots: A survey of conversational interfaces, patterns, and paradigms. Proceedings of the 2017 conference on designing interactive systems. 2017. P. 555–565.
- Von Ahn L. et al. CAPTCHA: Using hard AI problems for security. International conference on the theory and applications of cryptographic techniques. Springer, Berlin, Heidelberg, 2003. P. 294–311.
- Zhao B. et al. Towards evaluating the security of real-world deployed image CAPTCHAs. Proceedings of the 11th ACM Workshop on Artificial Intelligence and Security. 2018. P. 85–96.
- Wang J. et al. CAPTCHA recognition based on deep convolutional neural network. Math. Biosci. Eng. 2019. V. 16. № 5. P. 5851–5861.
- Zi Y. et al. An end-to-end attack on text CAPTCHAs. IEEE Transactions on Information Forensics and Security. 2019. V. 15. P. 753–766.
- Bursztein E., Martin M., Mitchell J. Text-based CAPTCHA strengths and weaknesses. Proceedings of the 18th ACM conference on Computer and communications security. 2011. P. 125–138.
- Golle P. Machine learning attacks against the Asirra CAPTCHA. Proceedings of the 15th ACM conference on Computer and communications security. 2008. P. 535–542.
- Sakkatos P. et al. Analysis of text-based CAPTCHA images using Template Matching Correlation technique. The 4th Joint International Conference on Information and Communication Technology, Electronic and Electrical Engineering (JICTEE). IEEE. 2014. P. 1–5.
- Ye G. et al. Yet another text CAPTCHA solver: A generative adversarial network based approach. Proceedings of the 2018 ACM SIGSAC Conference on Computer and Communications Security. 2018. P. 332–348.
- Парфенов А.С., Сычев П.П. Распознавание CAPTCHA на основе генеративно-состязательной сети // Системный анализ в науке и образовании: сетевое научное издание. 2020. № 2. С. 40–48. URL: http://sanse.ru/download/389.
- Li C. et al. End-to-end attack on text-based CAPTCHAs based on cycle-consistent generative adversarial network // Neurocomputing. 2021. V. 433. P. 223–236.
- Кущук Д.О. и др. Использование доменно-состязательного обучения для распознавания текстовых капч // Труды Института системного программирования РАН. 2020. Т. 32. № 4.
- Stark F. et al. CAPTCHA recognition with active deep learning. Workshop new challenges in neural computation. 2015. V. 2015. P. 94.
- Shi B., Bai X., Yao C. An end-to-end trainable neural network for image-based sequence recognition and its application to scene text recognition. IEEE transactions on pattern analysis and machine intelligence. 2016. V. 39. № 11. P. 2298–2304.
- Hussain R., Gao H., Shaikh R.A. Segmentation of connected characters in text-based CAPTCHAs for intelligent character recognition. Multimedia Tools and Applications. 2017. V. 76. № 24. P. 25547–25561.
- Thobhani A. et al. CAPTCHA Recognition Using Deep Learning with Attached Binary Images. Electronics. 2020. V. 9. № 9. P. 1522.
- Han S., Mao H., Dally W.J. Deep compression: Compressing deep neural networks with pruning, trained quantization and huffman coding. arXiv preprint arXiv:1510.00149. 2015.
- Frankle J., Carbin M. The lottery ticket hypothesis: Finding sparse, trainable neural networks. arXiv preprint arXiv:1803.03635. 2018.
- Hinton G., Vinyals O., Dean J. Distilling the knowledge in a neural network. arXiv preprint arXiv:1503.02531. 2015.
- Tang R. et al. Distilling task-specific knowledge from bert into simple neural networks. arXiv preprint arXiv:1903.12136. 2019.
- Graves A. et al. Connectionist temporal classification: labelling unsegmented sequence data with recurrent neural networks. Proceedings of the 23rd international conference on Machine learning. 2006. P. 369–376.
- Sanh V. et al. DistilBERT, a distilled version of BERT: smaller, faster, cheaper and lighter. arXiv preprint arXiv:1910.01108. 2019.
- Srivastava N. et al. Dropout: a simple way to prevent neural networks from overfitting. The journal of machine learning research. 2014. V. 15. № 1. P. 1929–1958.
- Goyal P. et al. Accurate, large minibatch sgd: Training imagenet in 1 hour. arXiv preprint arXiv:1706.02677. 2017.
- Geoffrey Hinton, Nitish Srivastava, Kevin Swersky. Neural networks for machine learning lecture 6a overview of mini-batch gradient descent. 2012. P. 14.
- Zhong Z., Jin L., Xie Z. High performance offline handwritten chinese character recognition using googlenet and directional feature maps. 2015 13th International Conference on Document Analysis and Recognition (ICDAR). IEEE. 2015. P. 846–850.
- Glorot X., Bordes A., Bengio Y. Deep sparse rectifier neural networks. Proceedings of the fourteenth international conference on artificial intelligence and statistics. JMLR Workshop and Conference Proceedings. 2011. P. 315–323.
- Graves A., Schmidhuber J. Framewise phoneme classification with bidirectional LSTM and other neural network architectures. Neural networks. 2005. V. 18. №. 5–6. P. 602–610.
- Sandler M. et al. Mobilenetv2: Inverted residuals and linear bottlenecks. Proceedings of the IEEE conference on computer vision and pattern recognition. 2018. P. 4510–4520.
- Ma N. et al. Shufflenet v2: Practical guidelines for efficient cnn architecture design. Proceedings of the European conference on computer vision (ECCV). 2018. P. 116–131.
- Ba L.J., Caruana R. Do deep nets really need to be deep? arXiv preprint arXiv:1312.6184. 2013.
- Watkins C.J.C.H., Dayan P. Q-learning. Machine learning. 1992. V. 8. № 3–4. P. 279–292.
- Van Hasselt H., Guez A., Silver D. Deep reinforcement learning with double q-learning. Proceedings of the AAAI Conference on Artificial Intelligence. 2016. V. 30. № 1.