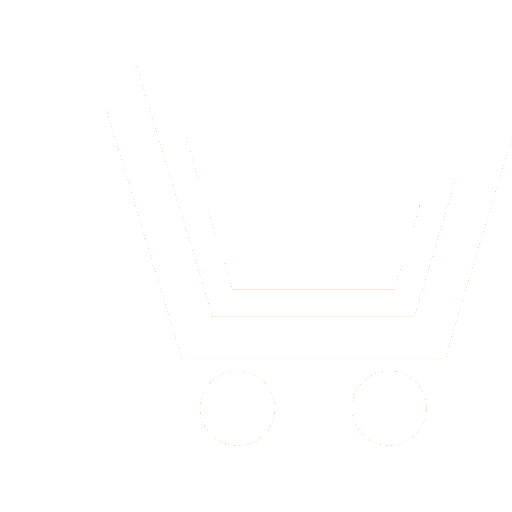
A.E. Krasnov1, T.N. Krasnova2
1 Russian State Social University (Moscow, Russia)
2 Moscow State University named after M.V. Lomonosov (Moscow, Russia)
Dementia is a disease in which the cognitive abilities (the ability to think) of the patient are impaired. Dementia causes gradual memory loss. The neurobiological cause of the disease is associated with the destruction of the synaptic connections of neurons in some parts of the cerebral cortex. Often there is also dementia due to arterial hypertension, leading to discirculatory arteriosclerotic encephalopathy.
Together with medical and neurobiological research to study the mechanisms of the brain (folding / unfolding of protein molecules, the functioning of the new cerebral cortex - the neocortex), a number of global computer projects (Folding@home, Blue Brain), focused on the use of cloud computing and supercomputers, have been launched. One of these projects (3D Atlas), designed to model the cortical columns of the brain on supercomputers, was carried out in Russia.
At the same time, along with global computer projects focused on numerous neurobiological and molecular models of computer simulation of the brain, it is of considerable interest to consider the simplest functional model of dementia using local neuron-like networks that can be implemented on personal computers.
This paper presents the results of modeling dementia based on a computer simulation of the disruption of synaptic connections in a neural three-layer feed-forward network. The stability of the associative response of the network was studied both in the presence of interference in the synaptic connections of its input peripheral layer, and in the event of a violation of the synaptic connections of neurons in the inner and output neuronal layers. It is shown that a network with three neural layers is resistant to the destruction of its synaptic connections. Thus, the network accurately (at the level of 5% significance) recognizes reference signals that differ in average variation by 13%, in the presence of 10% -15% discord in the setting of synaptic connections of the input peripheral neuron layer and of 10% -30% discord in the setting of synaptic connections of the neural layer. The results of the study demonstrate the mechanism of dementia development through the destruction of the functional connections of the collective interaction of peripheral and internal neuronal layers, which necessitates their constant training.
Krasnov A.E., Krasnova T.N. Dementia simulation based on the disruption imitation of neural network synaptic connections. Technologies of Living Systems. 2022. V. 19. № 3. Р. 22-36. DOI: https://doi.org/10.18127/j20700997-202203-03 (In Russian)
- Teljan V.S. Features of the manifestation of dementia: a review of the literature. Scientific Collection «InterConf», (46): with the Proceedings of the 1st International Scientific and Practical Conference «Current Issues and Prospects for the Development of Scientific Research» (March 19-20, 2021) at Orléans, France. № 46. P. 306–315. https://doi.org/10.51582/interconf.19-20.03.2021
- Tyler R. Ulland, Wilbur M. Song, Stanley Ching-Cheng Huang, et al. Trem2 Maintains Microglial Metabolic Fitness in Alzheimer’s Disease. Open Archive. 2017. V. 170. Iss. 4. P. 649–663. E3. https://doi.org/10.1016/j.cell.2017.07.023
- Bouras C., Hof P.R., Giannakopoulos P., Michel J.P., Morrison J.H. Regional distribution of neurofibrillary tangles and senile plaques in the cerebral cortex of elderly patients: a quantitative evaluation of a one-year autopsy population from a geriatric hospital. Cerebral Cortex journal. 1994. V. 4. № 2. P. 138–150. https://doi.org/10.1093/cercor/4.2.138
- Parfenov V.A. Vascular cognitive impairment and chronic cerebral ischemia (dyscirculatory encephalopathy). Neurology, Neuropsychiatry, Psychosomatics. 2019. V. 11(3S). P. 61–67. https://doi.org/10.14412/2074-2711-2019-3S-61-67
- Mikadze Yu.V., Skvortsov A.A. Soderzhaniye ponyatiy "neyropsikhologicheskiy faktor" i "neyropsikhologicheskiy sindrom" v kontekste metoda sindromnogo analiza A.R. Lurii. Voprosy psikhologii. 2014. № 4. S. 60–71. (in Russian).
- Kotsyubinskiy A., Sheynina N., Burkovskiy G. i dr. Funktsionalnyy diagnoz v psikhiatrii. SPb.: SpetsLit. 2013. 232 s. (in Russian).
- Krasnova T.N., Kryukova I.P., Krasnov A.E., et al. Principles of formalization of the syndrome diagnosis used in an automated system of management of patients. Biomed Eng. 1998. V. 32. P. 140–147. https://doi.org/10.1007/BF02369144
- Arnaldo Parra-Damas et al. CRTC1 Function During Memory Encoding Is Disrupted in Neurodegeneration, Biological Psychiatry. A Journal of Psychiatric Neuroscience and Therapeutics. 2017. V. 81(2). P. 11-123 (2017). https://doi.org/10.1016/j.biopsych.2016.06.025
- Juny Yokos, Ereiko Okubo-Suzuki, Masanori Nomoto, et. al. Overlapping memory trace indispensable for linking, but not recalling, individual memories. Science. 2017. V. 355. Iss. 6323. P. 398–403. DOI: 10.1126/science.aal2690
- Bennet B. Murdock, William E. Hockley. Short-Term Memory for Associations. Psychology of Learning and Motivation. 1989. V. 24. P. 71–108. https://doi.org/10.1016/S0079-7421(08)60535-6
- Samoylova T.A., Griber Yu.A. Intellektualnyy analiz tsvetovykh predpochteniy: poisk assotsiativnykh pravil vs. klasternyy analiz. Mir nauki. Pedagogika i psikhologiya. 2020. № 6. URL: https://mir-nauki.com/PDF/107PSMN620.pdf, https://doi.org/10.15862/ 107PSMN620 (in Russian).
- Munter J., Babaevskaya D., Wolters E.Ch. et al. Molecular and behavioural abnormalities in the FUS-tg mice mimic frontotemporal lobar degeneration: Effects of old and new anti-inflammatory therapies. Journal of Cellular and Molecular Medicine. 2020. V. 24. P. 10251–10257. https://doi.org/10.1111/jcmm.15628
- Khavinson V., Ilina A., Kraskovskaya N., et al. Neuroprotective Effects of Tripeptides–Epigenetic Regulators in Mouse Model of Alzheimer’s Disease. Pharmaceuticals. 2021. V. 14(6). P. 515. https://doi.org/10.3390/ph14060515
- Dorofeykova M.V., Petrova N.N., Egorov A.Yu. Eksperimentalnyye modeli kognitivnykh narusheniy pri neyrodegenerativnykh i organicheskikh rasstroystvakh. Rossiyskiy fiziologicheskiy zhurnal im. I.M. Sechenova. 2020. T. 24(2). S. 157–175. https://doi.org/10.31857/S086981392002003X (in Russian).
- Krasnov A.E., Nadezhdin E.N., Nikolskiy D.N., i dr. Nauchno-metodicheskoye obespecheniye sozdaniya programmnogo kompleksa dlya issledovaniya aktivnosti neyroglii v kortikalnykh kolonkakh golovnogo mozga. Informatizatsiya obrazovaniya i nauki. 2019. № 3(43). S. 3–14. URL: https://elibrary.ru/item.asp?id=38326978 (in Russian).
- Kokhonen T. Assotsiativnyye zapominayushchiye ustroystva. M.: Mir. 1982. 384 s. (in Russian).
- Pagiamtis K., Sheikholeslami A. Content-Addressable Memory (CAM) Circuits and Architectures: A Tutorial and Survey. IEEE J. of Solid-State Circuits. 2006. V. 41(3). P. 712–727. URL: https://www.pagiamtzis.com/pubs/pagiamtzis-jssc2006.pdf
- Bradbury J., Merity S., Xiong C., Socher R. Quasi-Recurrent Neural Networks. Under review as a conference paper at ICLR 2017. arXiv:1611.01576v2 [cs.NE] 21 Nov 2016. URL: arXiv:1611.01576 (cs)
- Tri M. Nguyen1, Logan A. Thomas, Jeff L. Rhoades et al. Structured connectivity in the cerebellum enables noise-resilient pattern separation. bioRxiv preprint. 2021. https://doi.org/10.1101/2021.11.29.470455
- Krasnov A.E., Kalachev A.A., Nadezhdin E.N., Nikol’skii D.N., et al. The Model of the Cybernetic Network and its Realization on the Cluster of Universal and Graphic Processors. Proceedings of the Scientific-Practical Conference “Research and Development - 2016”. 2016. P. 117–128. https://doi.org/10.1007/978-3-319-62870-7_13
- Karbasi A., Salavati A.H., Shokrollahi A., Varshney l.R. Noise Facilitation in Associative Memories of Exponential Capacity. Neural Computation. 2014. V. 26(11). P. 2493–2526. https://doi.org/10.1162/NECO_a_00655
- Krasnov A.E., Nadezhdin E.N., Nikol'skii D.N., Shmakova E.G. Multilayered neural-like network of direct propagation with the adjustment according to similarity measures of vectors of the learning sample. CEUR Workshop Proceedings. 2017. V. 2064. P. 209–218. https://www.scopus.com/inward/record.uri?eid=2-s2.0-85044520319&partnerID=40&md5 =e02ab3aa36ba811329b393721db8eef3
- Tarkov V.S. Assotsiativnaya pamyat na osnove kletochnoy neyronnoy seti s bipolyarnoy stupenchatoy funktsiyey aktivatsii. Prikladnaya diskretnaya matematika. 2020. № 48. S. 100–108. https://doi.org/10.17223/20710410/48/9 (in Russian).
- Poznyak A.S., Sanchez E.N., Yu W. Differential Neural Networks for Robust Nonlinear Control. Identification, State Estimation and Trajectory Tracking. Singapore: World Scientific Publishing. 2001. 422 p.
- Krasnov A.E., Nadezhdin E.N., Nikolskiy D.N., Kalachev A.A. Neyrosetevoy podkhod k probleme otsenivaniya effektivnosti funktsionirovaniya organizatsii na osnove agregirovaniya pokazateley eye deyatelnosti. Informatizatsiya obrazovaniya i nauki. 2017. № 1(33). S. 141–154. URL: https://informika.ru/pechatnye-izdaniya/zhurnal-informatizaciya-obrazovaniya-i-nauki/arhiv-vypuskov/2017/vypusk-n33/ (in Russian).
- Krasnov A.E., Pivneva S.V. Hierarchical quasi-neural network data aggregation to build a university research and innovation management system. In: Murgul V., Pukhkal V. (eds) International Scientific Conference Energy Management of Municipal Facilities and Sustainable Energy Technologies EMMFT 2019. EMMFT 2019. Advances in Intelligent Systems and Computing, V. 1259. Springer, Cham. https://doi.org/10.1007/978-3-030-57453-6_2
- Krasnov A.E., Saginov Yu.L., Feoktistova N.A. Kolichestvennoye otsenivaniye kachestva mnogoparametricheskikh obyektov i protsessov na osnove neyrosetevoy tekhnologii. Sbornik nauchnykh trudov Vserossiyskoy konferentsii «Informatsionnyye tekhnologii. menedzhment kachestva. informatsionnaya bezopasnost» (IT&MQ&IS-2015) – (20–25 maya 2015 g.). Uchebno-nauchnaya baza KBGU v Prielbrusye (p. Elbrus). Prilozheniye k zhurnalu «Kachestvo. Innovatsii. Obrazovaniye». 2015. T. 2. № 5. S. 97–108. URL: http://quality-journal.ru/wp-content/uploads/2016/07/ITMQIS-2015.pdf (in Russian).
- Krasnov A.E., Vagin V.A., Nikol’skii D.N. Fast Methods for Reducing Dimensionality of Spectral Data for their Visualization. Journal of Applied Spectroscopy. 2019. V. 86. Iss. 2. P. 369–369 (Russian Original 2019. V. 86. № 1. P. 101–105). https://doi.org/10.1007/s10812-019-00827-z
- Sb. dokladov II-y Mezhdunarodnoy konferentsii po neyronnym setyam i neyrotekhnologiyam (NeuroNT'2021). SPb. 2021. 66 s. https://doi.org/10.1109/NeuroNT53022.2021 (in Russian).
- Neural Networks. V. 144. Pages 1–790 (December 2021). URL: https://www.sciencedirect.com/journal/neural-networks/vol/144/suppl/C