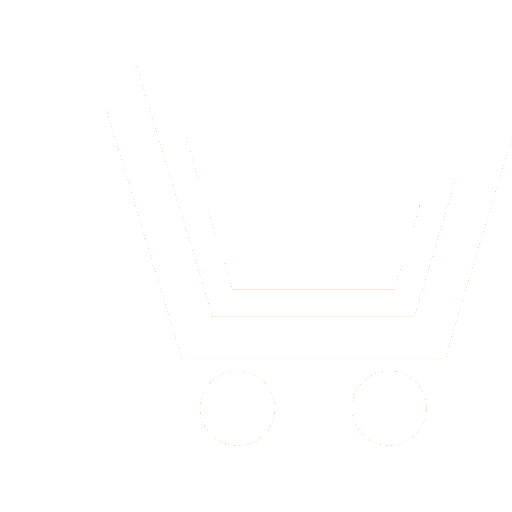
A.N. Narkevich1, T.Н. Mamedov2
1,2 FSBEI HE Prof. V.F. Voino-Yasenetsky KrasSMU MOH Russia (Krasnoyarsk, Russia)
Diabetic retinopathy is one of the most frequent complications in patients with diabetes mellitus. Accurate and timely diagnosis of this complication can save a person's vision. Medical professionals are increasingly becoming available to various intellectual and mathematical technologies in the form of decision support systems and diagnostics in general. Various intellectual and mathematical technologies are increasingly becoming available to doctors for decision support systems and diagnostics in general. Despite the modern methods proposed in medicine, the question of fast and accurate diagnosis of diabetic retinopathy remains open.
One of these methods for diagnosing diabetic retinopathy is the use of mathematical classification models to classify digital images of the fundus. These models have many customizable configurations, and combining several models into an ensemble can improve the accuracy of the classification of diabetic retinopathy. In this case, the results obtained at the output of several initial mathematical models are input for one resulting model.
The purpose of this study was to develop ensembles of classification mathematical models to improve the accuracy of automated diagnostics of diabetic retinopathy. The study used images from the open repository DIARETDB1 – Standard Diabetic Retinopathy Database. The construction of classification mathematical models and ensembles was carried out in the statistical package IBM SPSS Statistics v.19. The ensembles included the following classification mathematical models: discriminant equation, classification tree, logistic regression equation, and artificial multilayer neural network. As a result of the work, four ensembles of classification mathematical models were built. The resulting model in the ensembles was the classification tree model. Thanks to the creation of ensembles of classification mathematical models, an improvement was achieved in the accuracy of pixel classification for individual signs of diabetic retinopathy: hard exudates – 84.6 [84.4; 84.8]%; hemorrhages – 82.6 [81.7; 83.4]%; microaneurysms – 86.2 [85.1; 87.3]%; soft exudates – 92.3 [91.5; 93.0]%. The use of ensembles of models is a justified approach to improving the quality of the classification.
The constructed ensembles of classification mathematical models are a promising way to improve the classification accuracy of diabetic retinopathy and can be further used in decision support systems.
Narkevich A.N., Mamedov T.H. Application of ensembles of classification mathematical models to improve the accuracy of automated diagnostics of diabetic retinopathy. Technologies of Living Systems. 2021. V. 18. № 4. Р. 50−55. DOI: https://doi.org/10.18127/j20700997-202104-05 (In Russian)
- Dedov I.I., Shestakova M.V., Vikulova O.K. Epidemiologiya sakharnogo diabeta v Rossiyskoy Federatsii: kliniko-statisticheskiy analiz po dannym Federalnogo registra sakharnogo diabeta. Sakharnyy diabet. 2017. T. 20(1). S. 13–41. (in Russian).
- Dedov I.I., Shestakova M.V., Vikulova O.K., Zheleznyakova A.V., Isakov M.A. Epidemiologiya sakharnogo diabeta v Rossiyskoy Federatsii: Chto izmenilos za posledneye desyatiletiye? Terapevticheskiy arkhiv. 2019. T. 91(10). S. 4–13. (in Russian).
- Dedov I.I., Shestakova M.V., Vikulova O.K., Zheleznyakova A.V., Isakov M.A. Sakharnyy diabet v Rossiyskoy Federatsii: rasprostranennost. zabolevayemost. smertnost. parametry uglevodnogo obmena i struktura sakharosnizhayushchey terapii po dannym Federalnogo registra sakharnogo diabeta. status 2017 g. Sakharnyy diabet. 2018. T. 21(3). S. 144–159. (in Russian).
- Tsykunov D.V., Moiseyeva V.A. Primeneniye glubokogo obucheniya v zadache diagnostirovaniya zabolevaniy po izobrazheniyam opticheskoy kogerentnoy tomografii. Politekhnicheskiy molodezhnyy zhurnal. 2018. T. 12. S. 1–8. (in Russian).
- Abramoff M.D., Lou Y., Erginay A., Clarida W., Amelon R., Folk J.C., Niemejer M. Improved Automated Detection of Diabetic Retinopathy on a Publicly Available Dataset Through Integration of Deep Learning. Investigative Ophthalmology & Visual Science. 2016. V. 57(13). P. 5200–5206.
- Kapoor R., Walters S.P., Ai-Aswad L.A. The current state of artificial intelligence in ophthalmology. Survey of Ophthalmology. 2019. V. 64(2). P. 233–240.
- Kaur J., Mittal D. A generalized method for the segmentation of exudates from pathological retinal fundus images. Biocybernetics and Biomedical Engineering. 2018. V. 38(1). P. 27–53.
- Khojasteh P., Aliahmad B., Kumar D.K. Fundus images analysis using deep features for detection of exudates, hemorrhages and microaneurysms. BMC Ophthalmology. 2018. V. 18(288). P. 1–13.
- Ting D.S., Cheung G.C., Wong T.Y. Diabetic retinopathy: global prevalence, major risk factors, screening practices and public health challenges: a review. Clinical & Experimental Ophthalmology. 2016. V. 44(4). P. 260–277.
- Wong T.Y., Bressler N.M. Artificial intelligence with deep learning technology looks into diabetic retinopathy screening. JAMA. 2016. V. 316(22). P. 2366–2367.