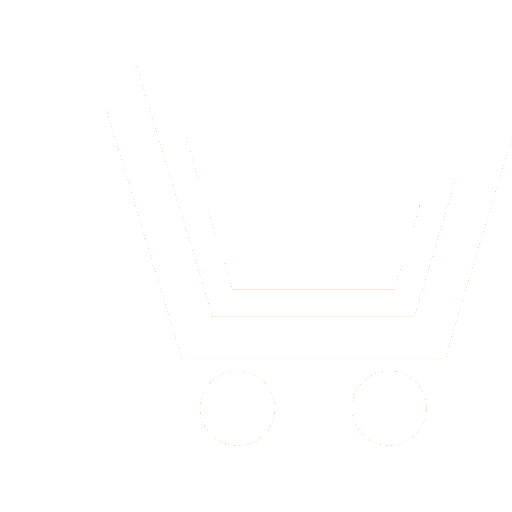
K.M. Paraskevopulo¹, A.N. Narkevich², K.A. Vinogradov³
1–3 Krasnoyarsk State Medical University n.a. prof. V.F. Voino-Yasenetsky Ministry of Health of Russia (Krasnoyarsk, Russia)
ABCDE performance rating system – method for detecting oncological diseases arising from pigmented skin lesions. This system very much depends on the doctor’s experience.
Mathematical modeling methods and computer technology allow the development of decision-making support systems, to help a specialist to determine: is it necessary for person to consult with oncologist and a biopsy to make histologically confirmed diagnosis.
For the application of such systems it is necessary to convert information about the external characteristics of the studied objects into a digital form, since a computer system can perceive only a set of object parameters in digital form, and not its image.
The aim of this research is characteristics representation of pigmented skin lesions in digital form, that have been identified using ABCDE system, and assessment of their applicability for skin cancer recognition.
6594 dermatoscopic images with histologically confirmed conclusions were taken for research from database of The International Skin Image Collaboration. Morphometric and color characteristics were identified for each skin image. These characteristics correspond to description parameters of pigmented skin lesions in ABCDE system. Possibility of applying parameters analysis in automated recognition of skin cancer was performed using Mann–Whitney U-test and ROC-curve. Object parametrization and characteristics analysis allowed to classify objects into benign and malignant neoplasms with 63,1% accuracy.
The practical significance of the research results lies in the fact that the obtained list of pigmented skin lesions parameters in digital form, which corresponds to the characteristics evaluated by objects using the ABCDE diagnostic system, can be used to develop decision-making support systems, as well as to study diagnosis of skin lesions problems in more depth from a position of computer vision.
Paraskevopulo K.M., Narkevich A.N., Vinogradov K.A. Parameterization of objects for recognition in digital skin images. Technologies of living systems. 2021. V. 18. № 1. P. 67–72. DOI: 10.18127/j20700997-202101-07 (In Russian). Tekhnologii zhivyh sistem / Technologies of living systems. V. 18. № 1. 2021. P. 67–72
- Voronina V.R. Melanocitarnye nevusy u detej: izmenyayushchiesya obrazovaniya u rastushchih pacientov. Praktika pediatra. 2019. № 1. S. 36–41 (In Russian).
- Zaripova G.R., Bogdanova Yu.A., Kataev V.A. Aktual'nye modeli sistem podderzhki pri-nyatiya reshenij v praktike vracha-hirurga. Mezhdunarodnyj nauchno-issledovatel'skij zhur-nal. 2016. T. 53. № 11. S. 137–142 (In Russian).
- Kubanov A.A., Sysoeva T.A., Gallyamova Yu.A., Bisharova A.S., Mercalova I.B. Algoritm obsledovaniya pacientov s novoobrazovaniyami kozhi. Lechashchij vrach. 2018. № 3. S. 83–88 (In Russian).
- Romanova O.A., Artem'eva N.G., Solohina M.G. Klinicheskie priznaki ABCDE v diagno-stike displasticheskogo nevusa s priznakami progressirovaniya i nachal'noj melanomy. Le-chashchij vrach. 2016. № 9. S. 92–97 (In Russian).
- Sinel'nikov I.E., Baryshnikov K.A., Demidov L.V. Klinicheskaya diagnostika melanomy kozhi. Vestnik RONC N. N. Blohina RAMN. 2017. T. 28. № 1. S. 68–73 (In Russian).
- Abadir A.P., Ali M.F., Karnes W., Samarasena J.B. Artificial intelligence in Gastrointestinal Endoscopy. Clinical Endoscopy. 2020. V. 53. № 2. P. 132–141.
- Bandic J., Kovacevic S., Karabeg R., Lazarov A., Opric D. Teledermoscopy for Skin Cancer Prevention: a Comparative Study of Clinical and Teledermoscopic Diagnosis. Acta Informatica Medica. 2020. V. 28. № 1. P. 37–41.
- Dhahri H., Rahmany I., Mahmood A., Ai Maghayreh E., Elkilani W. Tabu Search and Ma-chine-Learning Classification of Benign and Malignant Proliferative Breast Lesions. BioMed Re-search International. 2020. V. 2020. P. 1–10.
- Friedman E.B., Scolyer R.A., Thompson J.F. Management of pigmented skin lesions during pregnancy. Australian Journal of General Practice. 2019. V. 48. № 9. P. 621–624.
- Huang S., Cai N., Pacheco P.P., Narrandes S., Wang Y., Xu W. Applications of Support Vec-tor Machine (SVM) Learning in Cancer Genomics. Cancer Genomics Proteomics. 2018. V. 15. № 1. P. 41–51.
- Iftikhar P., Kuijpers M. V., Khayyat A., Iftikhar A., DeGouvia De Sa M. Artificial Intelligence: A New Paradigm in Obstetrics and Gynecology Research andы Clinical Practice. Cureus. 2020. V. 12. № 2. P. 1–9.
- Islam M.M., Poly T.N., Walther B.A., Yang H.C., Li Y.J. Artificial Intelligence in Ophtalmology: A Meta-Analysis of Deep Learning Models for Retinal Vessels Segmentation. Journal of Clinical Medicine. 2020. V. 9. № 4. P. 1–19.
- Vishwanath M., Jafarlou S., Shin I., Lim M. M., Dutt N., Rahmani A. M., Cao H. Investigation of Machine Learning Approaches for Traumatic Brain Injury Classification via EEG Assessment in Mice. Sensors. 2020. V. 20. № 7. P. 1–10.