350 rub
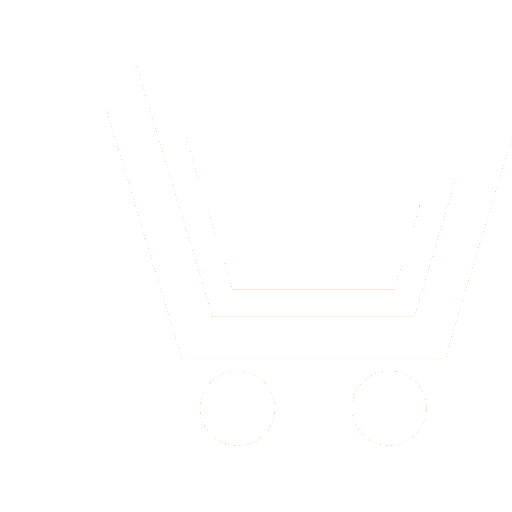
Journal Technologies of Living Systems №5 for 2012 г.
Article in number:
Statistical test diagnostics of parkinson-s disease EEG
Keywords:
statistical test
Parkinson's disease
electroencephalogram (EEG)
a convex set
the hyperplane
Authors:
F.N. Grigoriev, N.A. Kuznetsov
Abstract:
The problem of testing statistical hypotheses: the main hypothesis H0 the patient is suffering from Parkinson's disease and the alternative hypothesis for it H1 - the patient has no this disease. It is believed that all the information for the testing of hypotheses containes in the EEG recording the patient. It was chosen as the criterion for testing is statistical hypotheses H0 and H1.
It is assumed that at some time interval EEG potentials findings with sufficient accuracy for a given problem can be described as stochastic processes fourth-order autoregression with unknown parameters.
Estimates of these parameters for each patient were determined by solving the sistem of equations of the Yule-Walker. Test statistic was given by an affine function of the parameters of an autoregressive process.
Coefficients of affinity function (statistics) were determined by the «training set» containing n - 5 EEG clearly ill patients, and m 5 EEG of normal ones. Autoregressive process parameters were determined for training set of each patient.
Then, in a four-parameter space have been constructed set, ie, convex hulls for the parameters of patients and healthy subjects. It was followed constructed a hyperplane separating these sets. The equation of the hyperplane obtained was taken as test statistic.
Parameter estimates an autoregressive process the corresponding EEG were determined for the diagnosis of the patient. And these estimates were substituted into the expression for the statistic (the equation of the hyperplane).
If this gives a value greater than zero, there is a statistically well-founded suspicion that the patient is sick, and vice versa, if the resulting value is less than or equal to zero, then the patient is healthy.
The problem was completely solved for a particular training sample.
Pages: 58-62
References
- Беритов И.С. Структура и функции коры большого мозга. М.: Наука. 1969.
- Осовец С.М., Гинзбург Д.А., Гурфинкель В.С., Зенков Л.Р., Латаш Л.П., Малкин В.Б., Мельничук П.В., Пастернак Е.Б. Электрическая активность мозга: механизмы и интерпретация // Успехи физических наук. 1983. T. 141. № 1. С. 103 - 150.
- Щекутьев Г.А. Методика электроэнцефалографии. В кн.: Нейрофизиологические исследования в клинике. М.: Антидор. 2001. С. 16-24.
- Болдырева Г.Н. Стабильность спектрально-когерентных характеристик ЭЭГ человека // Успехи физиологических наук. 1994. Т. 25. № 1. С. 68-104.
- Воронов В.Г. Выявление статистически значимых особенностей в частотных спектрах электроэнцефалограмм // Труды YIII Междунар. конф. «Новые информационные технологии в медицине и технологии». Украина. Гурзуф. 2000. С. 244-245.
- Электрофизиологическое исследование стационарной активности в головном мозге. М.: Наука. 1983.
- Нидеккер И.Г. К вопросу о некорректном применении математики (на примере спектрального анализа электроэнцефалограмм) // Технологии живых систем. 2008. Т. 5. № 1. С. 42-56.
- Габова А.В., Гнездицкий В.В., Боснякова Д.Ю., Жарикова А.В., Самотаева И.С., Обухов Ю.В., Кузнецова Г.Д.Частотно-временная динамика разрядов «пик-волна» у пациентов с абсансной эпилепсией // Технологии живых систем. 2008. Т. 5. № 5-6. С. 72-81.
- Яглом А.М. Юла-Уокера оценки. Вероятность и математическая статистика. Энциклопедия. М.: Изд-во Большая Российская энциклопедия. 1999. С. 832.
- Болтянский В.Г. Оптимальное управление дискретными системами. М.: Наука.