350 rub
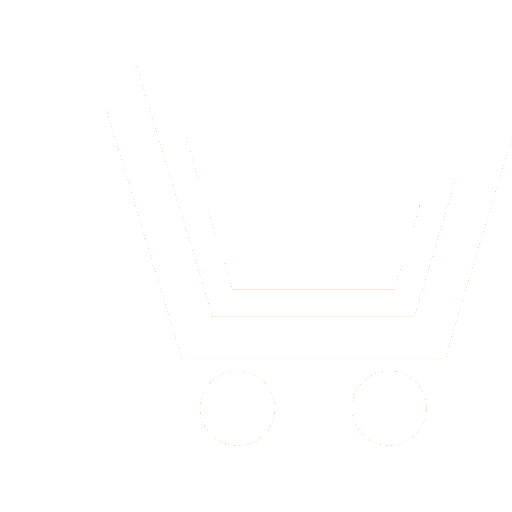
Journal Technologies of Living Systems №2 for 2011 г.
Article in number:
ANALYSIS THE LONG- AND SHORT SINGULARITIES OF HUMAN PHYSIOLOGICAL SIGNALS
Keywords:
physiological and biomedical signals
R/S analysis
persistent and antipersistent correlations
the local Hurst exponent
Authors:
S.A. Demin, B.N. Galimzyanov
Abstract:
In this is work studying the dynamics of long - and short-range correlations in human physiological signals is carried out on the base of R/S analysis. To calculate the Hurst exponent we use the "fast algorithm" as well as the window-time averaging algorithm with the following linear and piecewise-linear approximation. Analyzing the Hurst exponent local behavior is carried out after the choice of optimal window length.
Within the R/S analysis was carried out differentiation of biomedical signals, depending on the level of stochastic and/or determinism physiological processes. Register an increase in the persistent character of correlations in the case of providing a therapeutic effect on patients with Parkinsonian disease. Discovered reversible (slump - raise) the character of the dynamics of correlations during the tonic seizure at epilepsy. Revealed a similar character in the dynamics of the spread of ARI and influenza in social networks. Analysis of temporal behavior of the Hurst exponent H(t) revealed a persistent and antipersistent correlations in cer-tain areas of EEG signal man in the tonic seizure as well as the dynamics of the spread of ARI in Vakhitov region of Kazan city.
The results which demonstrate the principal possibility of fixation of even small individual differences in the responses of living systems confirm the need creating the "individual medicine" as the medicine of the future.
Pages: 3-17
References
- Altmann E.D., Kantz H. Recurrence time analysis, long-term correlations, and extreme events // Physical Review E. 2005. V. 71. P. 056106?1?9.
- Badii R., Politi A. Complexity: Hierarchical Structures and Scaling in Physics. New York: Cambridge University Press. 1997. 318 p.
- Rigozo N.R., Echer E., Nordemann D.J.R. et al.Comparative study between four classical spectral analysis methods // Appl. Math. Comput. 2005. V. 168. № 1. P. 411?430.
- Bickel D.R., Verklan M.T., Moon J. Detection of anomalous diffusion using confidence intervals of the scaling exponent with application to preterm neonatal heart rate variability // Physical Review E. 1998. V. 58. № 5. P. 6440?6448.
- Javors-kyj I., Isayev I., Zakrzewski Z.,Brooks S.P. Coherent covariance analysis of periodically correlated random processes // Signal Process. 2007. V. 87. № 1. P. 13?32.
- Chen Z., Ivanov P.Ch., Hu K. et al. Effects of nonlinearities on detrended fluctuation analysis // Physical Review E. 2002. V. 65. P. 041107?1?15.
- Hu K., Ivanov P.Ch., Chen Z. et al.Effect of trends on detrended fluctuation analysis // Physical Review E. 2001. V. 64. P. 011114?1?19.
- Peng C.-K., Buldyrev S.V., Havlin S. et al.On the mosaic organization of DNA sequences // Physical Review E. 1994. V. 49. P. 1685 ? 1689.
- Peng C.-K., Havlin S., Stanley H.E.,Goldberger A.L. Quantification of scaling exponents and crossover phenomena in nonstationary heartbeat time series // Chaos. 1995. V. 5. P. 82 - 87.
- Kantz H., Schreiber T. Nonlinear Time Series Analysis. Cambridge: Cambridge University Press. 1997. 304 p.
- Schreiber T., Schmitz A. Classification of time series data with nonlinear similarity measures // Physical Review Letters. 1997. V. 79. № 8. P. 1475?1478.
- Wolf A., Swift J.B., Swinney H.L,Vastano J.A. Determining Lyapunov exponents from a time series // Physica D. 1985. V. 16. P. 285 ? 317.
- Addison P.S. Wavelet analysis of the ECG: A review // Physiol. Meas. 2005. V. 26. P. 155 ? 199.
- Ivanov P.Ch., Rosenblum M.G., Peng C.-K. et al.Scaling behaviour of heartbeat intervals obtained by wavelet-based time-series analysis // Nature. 1996. V. 383. P. 323 ? 327.
- Percival D.B., Walden A.T. Wavelet Methods for Time Series Analysis. Cambridge: Cambridge University Press. 2000. 620 p.
- Permann D., Hamilton I. Wavelet analysis of time series for the Duffing oscillator: The detection of order within chaos // Physical Review Lettes. 1992. V. 69. № 18. P. 2607?2610.
- Timmer J., Lauk M., Deuschl G. Quantitative analysis of tremor time series // Electroencephalogr. Clin. Neuro. 1996. V. 101. № 5. P. 461?468.
- Timashev S.F.Generalization of Fluctuation-Dissipation relationships // Russ. J. Phys. Chem. A. 2005. V. 79. № 11. P. 1720?1727.
- Timashev S.F., Vstovskii G.V. Flicker-Noise Spectroscopy for Analyzing Chaotic Time Series of Dynamic Variables: Problem of Signal-to-Noise Relation // Russ. J. Electrochem. 2003. V. 39. № 2. P. 141?153.
- Yulmetyev R.M., Demin S.A., Panischev O.Yu. et al. Regular and stochastic behavior of Parkinsonian pathological tremor signals // Physica A. 2006. V. 369. № 2. P. 655?678.
- Farmer J.D., Sidorowich J.J. Predicting Chaotic Time Series // Physical Review Letters. 1987. V. 59. № 8. P. 845?848.
- Genςay R.A statistical framework for testing chaotic dynamics via Lyapunov exponents // Physica D. 1996. V. 89. P. 261 ? 266.
- Ivanov P.Ch., Amaral L.A.N., Goldberger A.L. et al.Multifractality in human heartbeat dynamics // Nature. 1999. V. 399. P. 461 ? 465.
- Liebovitch L.S., Yang W. Transition from persistent to antipersistent correlation in biological systems // Physical Review E. 1997. V.56. № 4. P. 4557?4566.
- Mandelbrot B.B. The Fractal Geometry of Nature. New York: W.H. Freeman and Comp. 1983. 460 p.
- Muzy J.-F., Bacry E., Kozhemyak A. Extreme values and fat tails of multifractal fluctuations // Physical Review E. 2006. V. 73. № 6. P. 066114?1?14.
- Sapir N., Karasik R., Havlin S. et al.Detecting scaling in the period dynamics of multimode signals: Application to parkinsonian tremor // Physical Review E. 2003. V. 67. P. 031903?1?8.
- Stanley H.E., Meakin P. Multifractal phenomena in physics and chemistry // Nature. 1988. V. 335. P. 405 ? 409.
- HurstH.E. Long term Storage Capacity of Reservoirs //Transactions of the American Society of Civil Engineers. 1951. V. 116. P. 770 ? 808.
- Hurst H.E., BlackR.P., SimaikaY.M. Long-Term Storage: An Experimental Study. London: Constable. 1965. 145 p.
- Бутаков В., Граковский А. Оценка уровня
стохастичности временных рядов произвольного происхождения при помощи показателя Хёрста // Comput. Model. New Tech. 2005.V. 9.
№ 2.P. 27?32. - Yulmetyev R.M., Gafarov F., Hänggi P. et al.Possibility between earthquake and explosion seismogram differentiation by discrete stochastic non-Markov processes and local Hurst exponent analysis // Physical Review E. 2001. V. 64. P. 066132?1?14.
- Юльметьев Р.М., Дёмин С.А., Панищев О.Ю., Хусаенова Э.В. Локальные особенности стохастической динамики живых систем //Технологии живых систем. 2007. Т. 4. № 2.С. 32?44.
- Yulmetyev R.M., Mokshin A.V., Hänggi P. Universal approach to overcoming nonstationarity, unsteadiness and non-Markovity of stochastic processes in complex systems // Physica A. 2005. V. 345. P. 303 ? 325.
- Beuter A., Titcombe M.S., Richer F. et al. Effects of deep brain stimulation on amplitude and frequency characteristics of rest tremor in Parkinson-s disease // Thalamus Relat. Syst. 2001. V.1. № 3.P. 203?211.
- Quiroga
R.Q., Blanco S., Rosso O.et al.Searching for
Hidden Information with Gabor Transform in Generalized Tonic-Clonic Seizures. ?
Electroenceph. and Clin. Neurophysiol. 1997.
V. 103. P. 434?439. - Yulmetyev R.M., Emelyanova N.A., Demin S.A. et al. Non-Markov stochastic dynamics of real epidemic process of respiratory infections // Physica A. 2004. V. 331. P. 300 ? 318.
- Timashev S.F., Polyakov Yu.S., Yulmetyev R.M. et al. Analysis of Biomedical Signals by Flicker-Noise Spectroscopy: Identification of Photosensitive Epilepsy Using Magnetoencephalograms // Las. Phys. 2009. V. 19. № 4. P. 836?854.