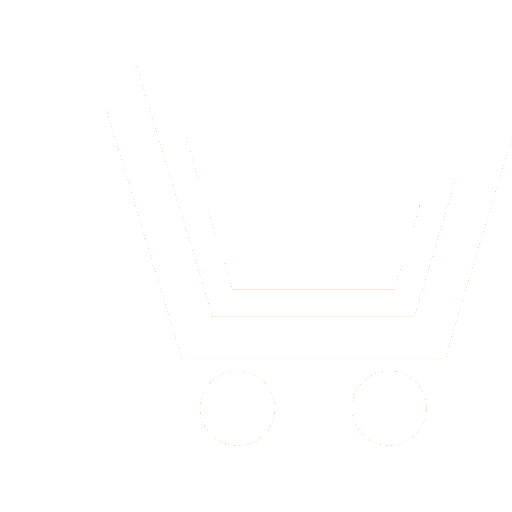
I.A. Prokopenkov1, A.S. Garkovenko2, V.V. Sukhov3, M.A. Puchkova4
1,2 Military Academy of Military Air Defense of the Armed Forces of the Russian Federation (Smolensk, Russia)
3,4 MIREA – Russian University of Technology (Moscow, Russia)
The development of information technology has led to an increase in the amount of data exchanged by complex systems. Timely assessment of the efficiency of complex systems allows you to quickly make management decisions. Classical approaches to collecting, storing and analyzing data are effectively used to solve problems of evaluating systems in which data sets are represented by a limited list of parameters. The functioning of complex systems leads to the fact that classical technologies for storing and processing databases do not allow for timely and high-quality analysis to form an assessment of the system, since it is often necessary to create a new metric to identify new dependencies in the data. To increase the efficiency of assessing complex systems based on the use of this approach, information about the functioning of the system is processed by data analysis specialists, which affects the economic component of the assessment process. The speed with which data analysts can evaluate information about the functioning of a system directly depends on their level of qualifications and knowledge of the subject area. This article presents the results of a study of an approach to the assessment of complex systems based on the representation of knowledge of a problem area in the form of an ontological model, the formation of fuzzy precedent bases regarding its classes, the construction and training of an assessment model in the form of a neuro-fuzzy classifier.
Prokopenkov I.A., Garkovenko A.S., Sukhov V.V., Puchkova M.A. An approach to evaluating complex systems based on ontology and neuro-fuzzy classifier. Science Intensive Technologies. 2023. V. 24. № 6. P. 61−71. DOI: https://doi.org/10.18127/ j19998465-202306-07 (in Russian)
- Tindova M. G., Ledneva O. V. Primenenie innovacionnyh instrumentov prodvizheniya novogo tovara v konkurentnoj srede. Sovremennaya konkurenciya. 2023. T.17. №1(91). S.87–95. DOI: 10.37791/2687-0649-2023-17-1-87-95 (in Russian).
- Halin V.G., Kalajda S.A., Chernova G.V., Yurkov A.V. Algoritmy formirovaniya modelej effektivnogo biznesa. Prikladnaya informatika. 2023. T. 18 №3(105). S. 5–21. DOI: 10.37791/2687-0649-2023-18-3-5-21 (in Russian).
- Romanov A.A., Basenko V.P., Zhukov B.M. Marketing: Ucheb. posobie. M.: Izdatel'sko-torgovaya korporaciya «Dashkov i K°», 2012. 440 s. (in Russian).
- Solov'eva Yu.N. Upravlenie marketingovoj kompetentnost'yu: uchebnik dlya vuzov. Pod red. G.L. Bagieva. SPb.: Asterion. 2015. 285 s. (in Russian).
- Godin A.M. Marketing: Uchebnik. M.: Izdatel'sko-torgovaya korporaciya «Dashkov i K°», 2013. 671 s. (in Russian).
- Taranov V.V. Metod ocenki privlekatel'nosti klientov. Markteing v Rossii i zarubezhom. 2013. № 1. S. 47–51 (in Russian).
- Romanenkova O.N. i dr. Marketingovye kommunikacii. Uchebnik i praktikum dlya prikladnogo bakalavriata: Uchebnik dlya vuzov po ekonomicheskim napravleniyam i special'nostyam. Pod red. O.N. Romanenkovoj. M.: Yurajt. 2014. 455 s. (in Russian).
- Oficial'nyj sajt Federal'noj sluzhby gosudarstvennoj statistiki [Elektronnyj resurs]: Rezhim dostupa: http://chelstat.gks.ru/html, svobodnyj (in Russian).
- Yarushkina N.G. Analiz slabostrukturirovannyh tekstov na osnove nechetkih ontologij. Gibridnye i sinergeticheskie intellektual'nye sistemy: GISIS 2018: materialy IV Vserossijskoj Pospelovskoj konferencii s mezhdunarodnym uchastiem. Svetlogorsk. 14–19 maya. Pod red. A.V. Kolesnikova. Kaliningrad: Izd-vo BFU im. I. Kanta. 2018. S. 133–139 (in Russian).
- Gribova V.V., Timchenko V.A. Koncepciya podderzhki lazernogo additivnogo proizvodstva na osnove ontologicheskogo podhoda. Ontologiya proektirovaniya. 2020. T. 10. № 2(36). S. 176–189 (in Russian).
- Muromcev D.I. Ontologicheskij inzhiniring znanij v sisteme Protégé. SPb.: ITMO. 2007. 62 s. (in Russian).
- Zagorul'ko Yu.A., Sidorov O.I., Borovikova O.I. Ontologicheskij podhod k postroeniyu sistem informacionnoj podderzhki nauchnoj i proizvodstvennoj deyatel'nosti. Vseross. konf. s mezhdunarodnym uchastiem «ZNANIYa – ONTOLOGII – TEORII» (ZONT-09): Sbornik trudov konferencii. Novosibirsk: Institut matematiki im. S.L. Soboleva SO RAN. 2009. T.2. S. 93–102 (in Russian).
- Gavrilova T.A., Horoshevskij V.F. Bazy znanij intellektual'nyh sistem. SPb.: Piter. 2001. 384 s. (in Russian).
- Prokopenkov I.A., Kotov D.V., Molyavko A.A. Sposob formirovaniya obobshchennyh precedentnyh reshenij na osnove kompozicionnogo ontologicheskogo podhoda. Sbornik nauchnyh trudov VIII Mezhdunarodnoj NPK «Nechetkie sistemy, myagkie vychisleniya i intellektual'nye tekhnologii». Smolensk: Universum, 2020. Ch. II. S. 128–135 (in Russian).
- Borisov V.V., Fedulov A.S., Zernov M.M. Osnovy nechetkoj matematiki. Chast' 4. Osnovy nechetkogo logicheskogo vyvoda. Uchebno-teoreticheskoe posobie po discipline «Nechetkie modeli i seti»: kurs lekcij. Smolensk: RIO filiala MEI v g. Smolenske. 2014. 102 s. (in Russian).
- Borisov V.V., Fedulov A.S., Zernov M.M. Osnovy nechetkoj matematiki. Chast' 5. Osnovy gibridizacii nechetkih modelej: Ucheb. posobie. M.: Goryachaya liniya – Telekom. 2016. 105 s. (in Russian).