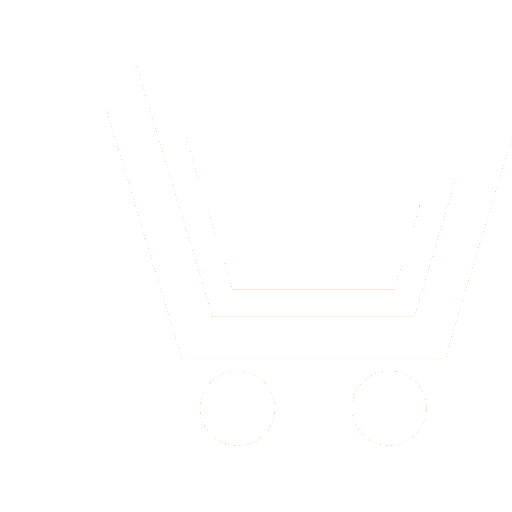
N.D. Badanina1, V.V. Dudihin2, I. Yu. Mezhuev3, N.S. Novikova4, I.Yu. Yakupov5
1–5 JSC «Distant Radiocommunication Scientific Research Institute» (Moscow, Russia)
A number of common classical methods and approaches are used to solve the problem of verifying objects detected on radar images based on data from an over-the-horizon radar station. However, these algorithms do not always provide the necessary level of accuracy, they may have a number of limitations on the functionality used, such as processing time, which plays an important role in real-time data analysis. The Goal is to supplement the list of methods based on neural network models. It is also essential to improve the quality of verification of objects in radar images. In this paper, an experimental study was carried out, which made it possible to select the architecture of an ensemble of neural networks that has the greatest generalizing ability, as well as to determine the optimal input data format for it. The projection of the three-dimensional observation space onto the plane made it possible to use models of convolutional neural networks trained on two-dimensional data. This approach has significantly improved the performance and accuracy of the model in comparison with training on data presented in three-dimensional space. The paper shows the effectiveness of including metadata about an object in the model. The developed approach can be used to solve various types of verification tasks of objects in the field of radiolocation, and can also be applied to improve existing software. The methods of training neural networks described in the article, their integration into an ensemble, as well as validation of the generalizing ability of models, can be transferred to similar tasks of verifying objects in three-dimensional data arrays obtained from other sources, or to time-sequential images.
Badanina N.D., Dudihin V.V., Mezhuev I.Yu., Novikova N.S., Yakupov I.Yu. Method of objects’ verification on radar images using ensembles of neural networks. Science Intensive Technologies. 2023. V. 24. № 2. P. 79−85. DOI: https://doi.org/10.18127/j19998465-202302-10 (in Russian)
- Farina A., Studer F. Cifrovaya obrabotka radiolokacionnoj informacii. Soprovozhdenie celej M.: Radio i svyaz'. 1993 (in Russian).
- Farber V.E. Osnovy traektornoj obrabotki radiolokacionnoj informacii v mnogokanal'nyh RLS. M.: MFTI. 2005 (in Russian).
- Berdyshev V.P., Garin E.N., Fomin A.N. i dr. Radiolokacionnye sistemy. Sib. feder. un-t., Krasnoyarsk. 2011 (in Russian).
- Tatuzov A.L. Nejronnye seti v zadachah radiolokacii. M.: Radiotekhnika. 2009 (in Russian).
- Dudihin V.V., Ivanov A.S., Mezhuev I.Yu., SHokov A.V., Yakupov I.Yu. Raspoznavanie radiolokacionnyh izobrazhenij, poluchennyh v millimetrovom diapazone, s ispol'zovaniem nejronnyh setej. Vestnik Koncerna VKO «Almaz – Antej». 2022. № 3. S. 48–58 (in Russian).
- Basov O.O., Tolstoj I.M., Le An' Tu. Klassifikaciya dvizhushchihsya nazemnyh ob"ektov po ih doplerovskim portretam s pomoshch'yu metodov mashinnogo obucheniya. Sovremennye naukoemkie tekhnologii. 2021. № 10 (in Russian).
- Kvasnov A.V. Intellektual'naya obrabotka radiolokacionnoj informacii. SPb.: Sankt-Peterburgskij politekhnicheskij universitet Petra Velikogo. 2020 (in Russian).
- Shchiryj A.O. Perspektivy primeneniya mashinnogo obucheniya dlya adaptacii zagorizontnyh radiolokacionnyh stancij dekametrovogo diapazona k geofizicheskim usloviyam. Postanovka zadach i vozmozhnye podhody. Sbornik nauchnyh statej po materialam VIII Mezhdunar. nauch.-prakt. konf. «Dal'nyaya radiolokaciya na sluzhbe Otechestvu», MGTU. M. 2022. C. 133–144 (in Russian).
- Kazachkov E.A., Matyugin S.N., Popov I.V., Sharonov V.V. Obnaruzhenie i klassifikaciya malorazmernyh ob"ektov na izobrazheniyah, poluchennyh radiolokacionnymi stanciyami s sintezirovannoj aperturoj. Vestnik Koncerna VKO «Almaz – Antej». 2018. № 1. S. 93–99 (in Russian).
- Zhang M. et al. Neural networks for radar waveform recognition. Symmetry. 2017. V. 9. № 5 (in Russian).
- Merkulov V.I., Verba V.S. (red.). Ocenivanie dal'nosti i skorosti v radiolokacionnyh sistemah. Ch. 3. M.: Radiotekhnika. 2010. Gl. 15.3 (in Russian).
- Pavlov V.A. Primenenie svertochnyh nejronnyh setej dlya obnaruzheniya i raspoznavaniya iskusstvennyh kosmicheskih i nazemnyh ob"ektov / Radiotekhnika. 2019. T. 83. № 12(20). S. 58–67. DOI: 10.18127/j00338486-201912(20)-08 (in Russian).
- Viryasova A. Yu., Klimov D.I., Hromov O.E., Gubajdullin I.R., Oreshko V.V. Analiz vozmozhnosti primeneniya svertochnyh nejronnyh setej i ih apparatnoj realizacii dlya zadachi termo-videotelemetrii / Radiotekhnika. 2021.