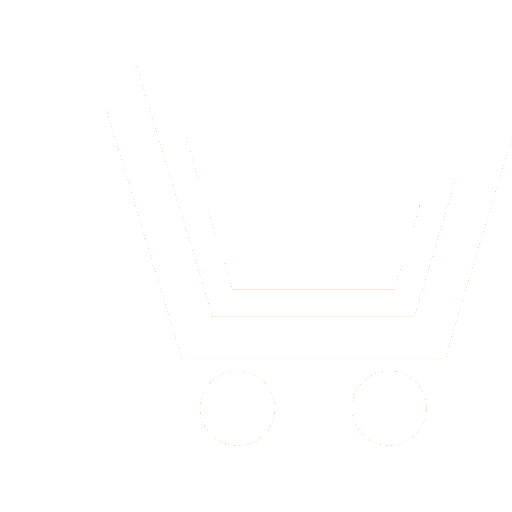
A.S. Zuev1, R.A. Isaev2
1,2 MIREA – Russian Technological University (Moscow, Russia)
The paper sets the task of integrating neural interfaces (brain-computer interfaces) as a new input device with graphical user interfaces (GUIs) as well as augmented, mixed and virtual realities based on a short overview of basic concepts of arranging human-computer interaction. An advanced alternative to pointing devices, touch screens, hand gesture, speech recognition and eye-tracking systems is neural interfaces which do not require physical action but are instead employed nowadays to analyze muscle activity (including the facial muscles) and the user’s concentration on controlling the movements of an object, while in the medium term – to also analyze the operator’s concentration on GUI elements and executable software features.
The paper presents a way of obtaining primary data to implement the tasks of controlling the horizontal and vertical movements of the object (cursor) on a plane as a result of training decision trees and neural networks. These were used by the authors to interpret brain signals registered by the non-invasive neural interface Emotiv Epoc+. The suggested method can also be applied in training neural networks and decision trees to control the object movements on a plane at a variable speed.
The authors have formulated the following hypothesis: the identification of brain activities according to diagonal object movements can be guaranteed without selecting the corresponding dataset classes for training decision trees and neural networks. Furthermore, they introduce a general implementation scheme of combining neural interfaces with devices of augmented, mixed and virtual realities and draw a conclusion about the multipurpose nature of these solutions for extended reality environments as well as for complex graphical user interfaces.
The study describes the unique role of neural interfaces as part of the facilities of human-machine and -computer interaction, which is induced by the brain transferring controls to hardware and software (currently available) and the sensory organs simulating signals to the brain (to become available in the medium term). The study also outlines the main areas of the research concerning neural interfaces carried out in the laboratories of the Institute of Information Technology at RTU MIREA.
Zuev A.S., Isaev R.A. Neural interfaces – a new facility for human-computer interaction. Science Intensive Technologies. 2022. V. 23.
№ 2. P. 70−82. DOI: https://doi.org/10.18127/j19998465-202202-07 (in Russian)
- Sharp H., Preece J., Rogers Y. Interaction Design: Beyond Human-Computer Interaction. 5th ed. New York: John Wiley & Sons Ltd., 2019. 636 p.
- Tidwell J., Brewer S., Valencia A. Designing Interfaces: Patterns for Effective Interaction Design 3rd Edition. Newton: O'Reilly Media, 2020. 302 p.
- Pavlov A.N., Hramov A.E. Nejrointerfejs: kak i zachem. Himiya i zhizn'. 2019. № 12. S. 15–21 (in Russian).
- Barfield W. Fundamentals of Wearable Computers and Augmented Reality, 2nd Edition. CRC Press, Inc.Subs. of Times Mirror 2000 Corporate Blvd. NW Boca Raton, FLUnited States, 2017. 739 p.
- Chuah S.H-W. Wearable XR-technology: literature review, conceptual framework and future research directions. International Journal of Technology Marketing. 2019. № 13(3/4). S. 205–259. https://doi.org/10.1504/IJTMKT.2019.104586.
- Chuah S.H-W. Why and Who Will Adopt Extended Reality Technology? Literature Review, Synthesis, and Future Research Agenda. Working paper, Universiti Sains Malaysia, School of Management, Department of Operation Management. 2019. № 56. https://doi.org/10.2139/ssrn.3300469.
- Mulla F., Eya E., Ibrahim E., Alhaddad A., Qahwaji R., Abd-Alhameed R. Neurological assessment of music therapy on the brain using Emotiv Epoc. Internet Technologies and Applications (ITA), 2017. https://doi.org/10.1109/ITEChA.2017.8101950.
- Babini M.H., Vladimir V Kulish, Namazi H. Physiological state and learning ability of students in normal and virtual reality conditions: complexity-based analysis. Journal of Medical Internet Research. 2020. № 22(6). https://doi.org/10.2196/17945.
- Rajanen D., Mikko R. A Review of Using EEG and EMG Psychophysiological Measurements in User Experience Research. University of Oulu, Information Processing Science, Master’s Thesis, Tewodros Belete Taffese. 2017.
- Murugappan M., Murugappan S., Gerard C. Wireless EEG signals based neuromarketing system using Fast Fourier Transform (FFT). IEEE 10th International Colloquium on Signal Processing and its Applications; 2014; Kuala Lumpur, Malaysia: IEEE. DOI: 10.1109/CSPA.2014.6805714.
- Blanco J.A., Vanleer A.C., Calibo T.K., Firebaugh S.L. Single-Trial Cognitive Stress Classification Using Portable Wireless Electroencephalography. Sensors 2019. № 19. P. 499; Sensors 2019. № 19(3). P. 499; https://doi.org/10.3390/s19030499.
- Zhang S., Yuan S., Huang L., Zheng X., Wu Z., Xu K., Pan G. Human Mind Control of Rat Cyborg’s Continuous Locomotion with Wireless Brain-to-Brain Interface. Scientific Reports 2019. № 9(1). P. 1321. https://doi.org/10.1038/s41598-018-36885-0.
- Suhov P.A., Chunihin A.O., Shabanov V.V., Shul'man V.D. Nejrointerfejs: tekhnologiya «chteniya mozga» v povsednevnoj. Voprosy ustojchivogo razvitiya obshchestva. 2021. № 7. S. 402–408 (in Russian).
- Morais P., Quintão C., Vieira P. Brain-Computer Interfaces by Electrical Cortex Activity: Challenges in Creating a Cognitive System for Mobile Devices Using Steady-State Visually Evoked Potentials. 7th Doctoral Conference on Computing, Electrical and Industrial Systems (DoCEIS). Costa de Caparica, Portugal. 2016. P. 135–141. https://doi.org/10.1007/978-3-319-31165-4_14.
- Kamesheva S.B. Tendencii razvitiya interfejsov: social'naya robototekhnika i cheloveko-mashinnoe vzaimodejstvie. Doklady Adygskoj (Cherkesskoj) Mezhdunarodnoj akademii nauk. 2020. № 4. S. 25–30. https://doi.org/10.47928/1726-9946-2020-20-4-25-30 (in Russian).
- Popov A.A. Ergonomika pol'zovatel'skih interfejsov v informacionnyh sistemah: uchebnoe posobie. M.: RUSAJNS. 2016. 312 s. (in Russian).
- Smirnova A.V. Ustrojstva otobrazheniya. Vidy monitorov. Uchebnye materialy. Elektronnoe izdanie Kotovskogo promyshlenno-ekonomicheskogo tekhnikuma. Kotovo. 2019 (in Russian).
- Thang N.T. Algoritmy raspoznavaniya zhestov na videoposledovatel'nostyah: Avtoref. diss. … kand. tekhn. nauk: 05.13.11. [b. i.], 2014. URL: http://vital.lib.tsu.ru/vital/access/manager/Repository/vtls:000469053. (data obrashcheniya 08.01.2022). – Tekst: elektronnyj (in Russian).
- Markovnikov N.M., Kipyatkova I.S. Analiticheskij obzor integral'nyh sistem raspoznavaniya rechi. Informatika i avtomatizaciya (Trudy SPIIRAN). 2018. vypusk 3 (58). https://doi.org/10.15622/sp.58 (in Russian).
- Polyakov E.B., Hahina A.M. Tendencii razvitiya pol'zovatel'skogo interfejsa. Nauka, obrazovanie, innovacii: gumanitarnye, estestvenno-nauchnye i tekhnicheskie resheniya sovremennosti: Materialy XXIII Vseros. nauch.-prakt. konf. Yuzhnyj universitet (IUBiP). 2020.
S. 58–61 (in Russian). - Shneiderman B. The future of interactive systems and the emergence of direct manipulation. Behaviour and Information Technology. 1982. № 1(3). P. 237–256, https://doi.org/10.1109/MC.1983.1654471.
- Smilkov D., Carter S., Sculley D., Viégas F.B., Wattenberg M. Direct-Manipulation Visualization of Deep Networks. Cornell University. Submitted on 12 Aug 2017.
- Shneiderman B. Direct manipulation: A step beyond programming languages. Computer. 1983. № 16(8). P. 57–69.
- Kwon B.C., Javed W., Elmgvist N., Yi J.S Direct manipulation through surrogate objects. Proceedings of the SIGChI Conference on Human Factors in Computing Systems. 2011. P. 627–636. https://doi.org/10.1145/1978942.1979033.
- Papagiannis H. Dopolnennaya real'nost'. Vse, chto vy hoteli uznat' o tekhnologii budushchego. M.: Eksmo. 2019. 288 s. (in Russian).
- Sosnilo A.V., Kreer M.Y., Petrova V.V. AR/VR technologies in management and education. Upravlenie. 2021. № 2(9). S. 114–124. https://doi.org/10.26425/2309-3633-2021-9-2-114-124.
- Ivanova A.V. Tekhnologii virtual'noj i dopolnennoj real'nosti: vozmozhnosti i prepyatstviya primeneniya. Strategicheskie resheniya i risk-menedzhment. 2018. № 3(108). S. 88–107. https://doi.org/10.17747/2078-8886-2018-3-88-107 (in Russian).
- Speicher M., Hall B.D., Nebeling M. What is Mixed Reality? In: Proc. of the 2019 ChI Conference on Human Factors in Computing Systems. Glasgow, Scotland, UK. 2019. № 537. P. 1–15. https://doi.org/10.1145/3290605.3300767.
- Tang Y. M., Au K. M., Lau H. C. W., Ho, G. T. S., Wu C. H. Evaluating the effectiveness of learning design with mixed reality (MR) in higher education. Virtual Reality. 2020. № 24(4). P. 797–807. https://doi.org/10.1007/s10055-020-00427-9.
- Zuev A.S., Makushchenko M.A., Ivanov M.E., Merkulov E.S. Tekhnologii rasshirennoj real'nosti – novyj komponent promyshlennoj inzhenerii i proizvodstvennyh sistem. Rossijskij tekhnologicheskij zhurnal. 2020. № 8(4). S. 46–65. https://doi.org/10.32362/2500-316X-2020-8-4-46-65 (in Russian).
- Milgram P., Kishino F. A Taxonomy of Mixed Reality Visual Displays. IEICE Transactions on Information Systems. 1994. E77-D(12).
P. 1321–1329. - Azevich A.I. Dopolnennaya real'nost' i dopolnennaya virtual'nost' kak vidy immersionnyh tekhnologij obucheniya. Continuum. Matematika. Informatika. Obrazovanie. 2020. № 2(18). S. 79–84. https://doi.org/10.24888/2500-1957-2020-18-2-79-84 (in Russian).
- Dezhina I.G., Kotelevcev Yu.V. Tekhnologii vosstanovleniya i rasshireniya resursov mozga cheloveka: publichnyj analiticheskij doklad. M.: OOO «Lajm». 2020. 256 s. (in Russian).
- Pavlova L.P. Dominanty deyatel'nogo mozga. Sistemnyj psihofiziologicheskij podhod k analizu EEG. SPb.: Inform-navigator. 2017. 442 s. (in Russian).
- Kalacheva N.Yu. Magnitoencefalografiya. Fizika i medicina: sozdavaya budushchee: sbornik materialov. 2018. S. 56–58 (in Russian).
- Harlamov N.D., Astrelin A.A., Zhinzhikov K.N. Zubkov A.V., Shurlaeva E.A., Rozaliev V.L. Analiz podhodov dlya realizacii programm, rabotaYushchih s ispol'zovaniem nejrointerfejsa. Programmnaya inzheneriya: sovremennye tendencii razvitiya i primeneniya: sbornik materialov IV Vserossijskoj nauchno-prakticheskoj konferencii, posvyashchennoj 30-letiYu sozdaniya kafedry programmnoj inzhenerii. 2020. S. 87–90 (in Russian).
- Zubkov A.V., Kamennov Ya.E., Orlova Yu.A., Rozaliev V.L. Izuchenie modelej mozgovoj aktivnosti, ispol'zuya nejrointerfejs. Innovacionnye, informacionnye i kommunikacionnye tekhnologii. 2019. № 1. S. 187–191 (in Russian).
- Speshilova N.V., Andrienko D.A., Rahmatullin R.R., Speshilov E.A. Analiz i ocenka gorizontov primeneniya tekhnologii nejrointerfejsa pri realizacii koncepcii «Industriya 4.0» v konkuriruYushchem mirovom ekonomicheskom prostranstve. Vestnik Evrazijskoj nauki. 2019.
№ 2. S. 1–12 (in Russian). - Samieva F.F., Chuficheva E.A. Nejrointerfejs: ot teorii k poslednim razrabotkam. Fizika i medicina: sozdavaya budushchee: sbornik materialov II nauch.-prakt. konf. studentov i molodyh uchyonyh nauchno-obrazovatel'nogo medicinskogo klastera «Nizhnevolzhskij» «Fizika i medicina». 2018. S. 287–291 (in Russian).
- Bodin O.N., Solodimova G.A., Spirkin A.N. Nejrointerfejs dlya upravleniya robotizirovannymi ustrojstvami. Izmerenie. Monitoring. Upravlenie. Kontrol'. 2019. № 4(30). S. 70–76. https://doi.org/10.21685/2307-5538-2019-4-8 (in Russian).
- Koshevaya D.O., Ruch R.O., Kadykov N.Yu., Kamcev V.A., Filipovich O.V. Zadacha ispol'zovaniya nejrointerfejsa dlya upravleniya manipulyatorom didakticheskogo naznacheniya. Mir komp'yuternyh tekhnologij: sbornik statej Vserossijskoj nauchno-tekhnicheskoj konferencii studentov, aspirantov i molodyh uchenyh. 2019. S. 67–71 (in Russian).
- Loboda Yu.O., Kataev S.G., Gasymov Z.A., Nemchaninov A.A. Upravlenie mekhatronnoj rukoj s pomoshch'Yu nejrointerfejsa. Elektronnye sredstva i sistemy upravleniya. Materialy dokladov Mezhdunarodnoj nauchno-prakticheskoj konferencii. 2018. № 1–2. S. 191–192 (in Russian).
- Zhang G., Davoodnia V., Sepas-Moghaddam A., Zhang Y., Etemad A. Classification of Hand Movements From EEG Using a Deep Attention-Based LSTM Network. IEEE Sensors Journal. 2020. 6 p. https://doi.org/10.1109/JSEN.2019.2956998.
- Md. A. Mannan Joadder, Myszewski J.J., Rahman M.H., Wang I. A performance-based feature selection technique for subject independent MI based BCI. Health Inf Sci Syst. 2019. № 7(1). 7 p. https://doi.org/10.1007/s13755-019-0076-2.
- Ji N., Ma. L., Dong H., Zhang X. EEG Signals Feature Extraction Based on DWT and EMD Combined with Approximate Entropy. Brain Sci. 2019. № 9(8). https://doi.org/10.3390/brainsci9080201.
- Edla D.R., Mangalorekar K., Dhavalikar G., Dodia S. Classification of EEG data for human mental state analysis using Random Forest Classifier. Procedia Computer Science. 2018. № 132. P. 1523–1532. https://doi.org/10.1016/j.procs.2018.05.116.