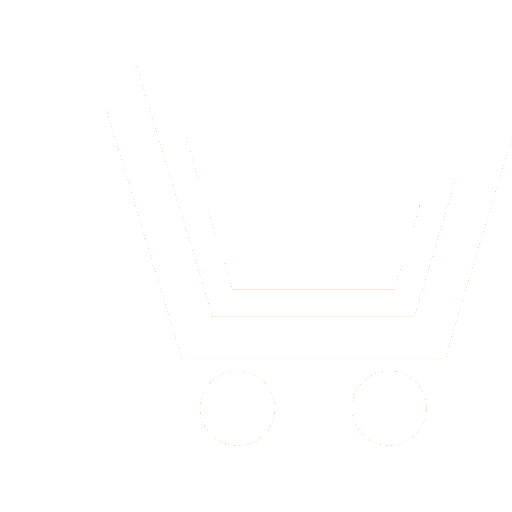
O.V. Nepomnyashchiy, A.V. Tarasov, Yu.V. Krasnobaev, V.N. Khaidukova, D.O. Nepomnyashchiy
Siberian Federal University (Krasnoyarsk Russia)
The problem of increasing the efficiency of power units of autonomous electric transport vehicles is considered. The task of creating a promising power system control device has been singled out. It is determined that in creating such devices, significant results can be obtained by using an intelligent module in the control loop of the electric drive.
Goal. It is necessary to develop a power plant model with intelligent control, allowing to obtain data sets about currents, voltages and engine speeds in different modes of operation.
The architecture of an intelligent control device, a PID controller based on a neural network, has been proposed; it has been proposed to exclude rotor angular velocity sensors from the classical feedback loop. The type and architecture of the neural network is defined. In the software environment MatLab the model of neuroemulator of the engine for formation of a training sample of a neural network by a method of Levenberg – Marquardt is developed. The trained neural network is implemented in the developed model of the electric motor control loop.
The results of simulation of the intelligent control device showed a good convergence of the output influences generated by the neuroemulator with the actual parameters of the electric motor.
Nepomnyashchiy O.V., Tarasov A.V., Krasnobaev Yu.V.,Khaidukova V.N., Nepomnyashchiy D.O. Model of a neural network adaptive system for a digital control loop of an electric drive. Science Intensive Technologies. 2021. V. 22. № 2. P. 34−42. DOI: https://doi.org/10.18127/j19998465-202102-04 (In Russian)
- Bobcov A.A., Pyrkin A.A. Adaptivnoe i robastnoe upravlenie s kompensaciej neopredelennostej: Ucheb. posobie. SPb.: NIU ITMO. 2013. 135 s. (In Russian).
- Aleksandrov A.G., Palenov M.V. Sostoyanie i perspektivy razvitiya adaptivnyh PID-regulyatorov v tekhnicheskih sistemah. Avtomatika i telemekhanika. 2014. № 2. S. 16–30 (In Russian).
- Astrom K.J., Hagglund T. Advanced PID Control. Research Triangle Park: ISA – The Instrumentation, Systems, and Automation Society. 2006. 461 p.
- Sidorova A.A., Malyshenko A.M. Analiz effektivnosti algoritmov avtomaticheskoj nastrojki adaptivnyh promyshlennyh PID-regulyatorov. Izv. Tomskogo politekhnicheskogo universiteta. 2011. T. 318. № 5. S. 6 (In Russian).
- Glushchenko A.I. O metode opredeleniya skorosti obucheniya nejronnoj seti dlya zadachi operativnoj nastrojki linejnyh regulyatorov pri upravlenii nelinejnymi ob"ektami. Staryj Oskol: Starooskol'skij tekhnologicheskij institut im. A.A. Ugarova (filial) FGAOU VO NITU «MISiS». 2018. S. 52–107 (In Russian).
- Terekhov V.M., Osipov O.I. Sistemy upravleniya elektroprivodov: Uchebnik dlya vuzov / Pod red. V.M. Terekhova. Izd. 2-e, ster. M.: Izdatel'skij centr «Akademiya». 2006. 304 s. (In Russian).
- Udut L.S., Mal'ceva O.P., Koyain N.V. Proektirovanie i issledovanie avtomatizirovannyh elektroprivodov. Ch. 8. Asinhronnyj chastotnoreguliruemyj elektroprivod. Tomsk: Izd-vo TPU. 2010. 448 s. (In Russian).
- Langraf S.V., Glazyrin A.S., Afanas'ev K.S. Primenenie nablyudatelya Lyuenbergera dlya sinteza vektornyh bezdatchikovyh asinhronnyh elektroprivodov. Izv. vuzov. Ser. Elektromekhanika. 2011. № 6. S. 57–61 (In Russian).
- Langraf S.V., Glazyrin A.S., Glazyrina T.A., Afanas'ev K.S., Timoshkin V.V., Kozlova L.E. Issledovanie parametricheskoj robastnosti bezdatchikovogo vektornogo asinhronnogo elektroprivoda s identifikatorom Kalmana. Izv. Tomskogo politekhnicheskogo universiteta. 2010. T. 317. № 4. S. 120–123 (In Russian).
- Vinogradov A.B. Vektornoe upravlenie elektroprivodami peremennogo toka. Ivanovo: GOUVPO «Ivanovskij gosudarstvennyj energeticheskij universitet im. V.I. Lenina». 2015. 298 s. (In Russian).
- Kyslan K., Fedák V. DC motor identification based on Recurrent Neural Networks. Computer Science: Conference Paper. January 2015. Faculty of Electrical Engineering and Informatics. Technical University of Košice, Letná 9, 042 00 Košice, Slovak Republic. P. 5.
- Nesterov K.E. Razrabotka i issledovanie sistemy «Tiristornyj preobrazovatel' napryazheniya – asinhronnyj dvigatel'» s vychislitelem skorosti rotora po EDS statora: Dis. … kand. tekhn. nauk. Ekaterinburg. 2009. 140 s. (In Russian).
- Kozlova L.E., Bolovin E.V. Issledovanie statiki i dinamiki zamknutogo bezdatchikogo asinhronnogo elektroprivoda sobstvennyh nuzhd TES po skheme TRN – AD s nejrosetevym nablyudatelem uglovoj skorosti [elektronnyj resurs] Rezhim dostupa: http://www.scienceeducation.ru/117-13581. Sovremennye problemy nauki i obrazovaniya. 2014. № 3. 6 c. (In Russian).
- Costa M.A., Braga A., Menezes B.R. Improving generalization of MLPs with sliding mode control and the Levenberg-Marquardt algorithm. Neurocomp. 2007. V. 70. № 7–9. P. 1342–1347.