350 rub
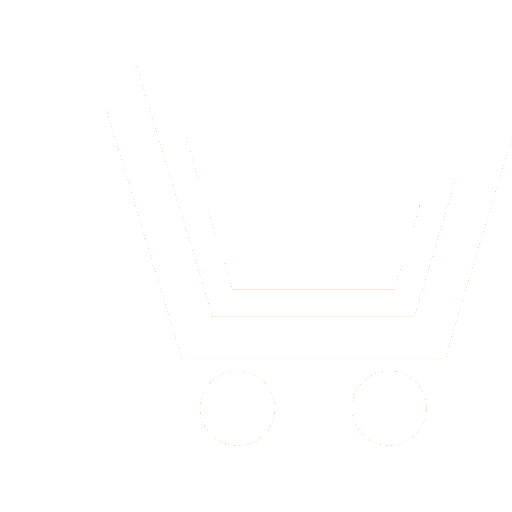
Journal Science Intensive Technologies №11 for 2015 г.
Article in number:
A comparative study of the denoising algorithms-s effectiveness for mri brain images
Keywords:
MRI images
spatial and frequency filtering
mean square error
peak signal / noise ratio
performance
Authors:
A.R.A. Abdulraqeb - Post-graduate Student, Department Biomedical and Electronic systems and technology, Vladimir State University named after A.&N. Stoletovs. E-mail: atef_alsanawy@mail.ru
L.T. Sushkova - Dr. Sc. (Eng.), Professor, Head of Department Biomedical and Electronic systems and technology, Vladimir State University named after A.&N. Stoletovs. E-mail: ludm@vlsu.ru
K.V. Chirkov - Ph. D. (Eng.), Associate Professor, к Biomedical and Electronic systems and technology, Vladimir State University named after A.&N. Stoletovs. E-mail: chrkv@mail.ru
Abstract:
Magnetic resonance imaging (MRI), as a method of medical imaging is widely used for the diagnosis and treatment of brain tumors. Often the MR image have a noise component, which not only reduces the distinguishability of objects with low contrast, but, in general, reduces the visual quality and have a negative influence on the result of segmentation and recognition of pathological lesions.
MRI images as the result of a complex multi-component technical system, display different physical effects in the internal tissues of the body and contain multi-noise (Gaussian noise, impulse noise) [11, 12]. To determine the optimal filter enhances the effectiveness of noise removing in the MRI images of the brain, in this the task is to make a research and comparative analysis of the most frequently used in MRI images processing filters (averaging filter, Gaussian filter, Wiener filter, median filter, Butterworth and Haar wavelet).
The study showed that among the considered filter higher quality filtering results of MRI brain images provide a Gaussian filter and Wiener, as evidenced by the values obtained criterion assessments. Thus, for the mean square error of the Gaussian filter MSE = 0,0044, peak signal / noise ratio PSNR = 47,1954 and speed (estimated time spent on filtering process) is 0,3974 s. For Wiener filter respectively: MSE = 0,0068; PSNR = 43,3859 and performance is 0,9023 s. From the point of view of computational efficiency and simplicity of implementation, more fast is the averaging filter (computation time is 0,3398 s).
Pages: 62-68
References
- Marusina M.JA., Kaznacheeva A.O. Sovremennye vidy tomografii. Ucheb. posobie. SPb.: SPbGU ITMO. 2006. 152 s.
- McRobbie D.W., Moore E.A., Graves M.J., Graves M.R. MRI From Picture to Proton Cambridge University Press, New York Cambridge University Press. NewYorkGraves. 2003.P. 411.
- Gonsales R.S.,Vud R.EH. Cifrovaja obrabotka izobrazhenijj. M.: Tekhnosfera. 2005. 1072 s.
- Semenov S.I. Informacionno-diagnosticheskaja sistema dlja onkologii. Vladimir: Rost. 2002.208 c.
- Pavithra R., Ramya R., Alaiyarasi G. Wavelet Based Non Local Means Algorithm for Efficient Denoising of MRI Images // International Journal of Advanced Research in Computer and Communication Engineering. V. 4. № 2. 2015. P. 388−392.
- Marcos Martin-Fernandez, Sergio Villullas. The EM Method in a Probabilistic Wavelet-Based MRI Denoising // Computational and Mathematical Methods in Medicine. V. 2015. ArticleID 182659. 21 p.
- Siti Noraini Sulaiman, Siti Mastura Che Ishak, Iza Sazanita Isa, Norhazimi Hamzah. Denoising of Noisy MRI Brain Imageby Using Switching-based Clustering Algorithm // Energy, Environment, Biology and Biomedicine. 2014. P. 33−39.
- Strugajjlo V.V. Obzor metodov filtracii i segmentacii cifrovykh izobrazhenijj // Nauka i obrazovanie (ehlektronnyjj nauchno-tekhnich. zhurnal). MGTU. 2012. 270−281 s.
- Gruzman I.S., Kirichuk V.S., Kosykh V.P., Peretjagin G.I., Spektor A.A. Cifrovaja obrabotka izobrazhenijj v informacionnykh sistemakh: Ucheb. posobie. Novosibirsk: Izd-vo NGTU. 2002. 352 c.
- Aggelos K. Katsaggelos. Osnovy obrabotki cifrovykh video i izobrazhenijj. Northwestern university / https://www.coursera.org/ course/digital (dataobrashenija 05.08.2015).
- Anisha S.R., Venugopala Krishnan J.Comparison of various filters for noise removal in MRI brain image // International Conference on Futuristic Trends in Computing and Communication (ICFTCC-2015). P. 68−73.
- H. Oulhaj, A. Amine, M. Aziza, D. Aboutajdine. Noise reduction in medical imaging - comparison of noise removal algorithms //International Conference on Mathematics and Computer Science (ICMCS). 2012.6320218.
- Bondina N.N., Kalmychkov A.S., Krivencov V.EH. Sravnitelnyjj analiz algoritmov filtracii medicinskikh izobrazhenijj //Vestnik NTU «KHPI». 2012. № 38.S. 14−25.