350 rub
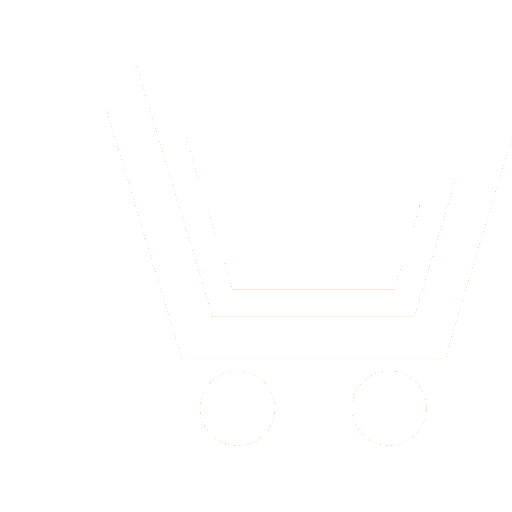
Journal Science Intensive Technologies №11 for 2015 г.
Article in number:
Pretreatment of noisy speech signals using binary masks in the problem of speaker identification
Authors:
G.S. Tupitsin - Post-graduate Student, Department of Electronic Systems dynamics, Yaroslavl State University. E-mail: genichyar@genichyar.com
A.I. Topnikov - Ph. D. (Eng.), Department of Electronic Systems dynamics, Yaroslavl State University. E-mail: topartgroup@gmail.com
A.L. Priorov - Dr. Sc. (Eng.), Associate Professor, Department of Electronic Systems dynamics, Yaroslavl State University. E-mail: andcat@yandex.ru
Abstract:
One of the most effective ways of increasing robustness to noise of the speaker identification systems is using of noise reduction algorithms.
A noise reduction algorithm using the binary mask with a threshold decision rule based on signal / noise ratio (SNR) estimating by the two-step algorithm (Two Step Noise Reduction - TSNR) was proposed in this paper.
The proposed algorithm was tested and compared with the existing noise reduction algorithms in the problem of speaker identification. Testing was carried out using noise samples from the NOISEX 92 library. The advantage of the new noise reduction algorithm for some noise samples and SNRs was shown.
Pages: 56-61
References
- Sorokin V.N., Vjugin V.V., Tananykin A.A. Raspoznavanie lichnosti po golosu: analiticheskijj obzor // Informacionnye processy. 2012. T. 12. № 1. S. 1−30.
- Furui S. An overview of speaker recognition technology // ESCA Workshop on Automatic Speaker Recognition, Identification and Verification. 1994. P. 1−9.
- Bansal S., Hooda A., Anima Speaker identification system using close set // International journal of research in Engineering and Technology. 2012. V. 1. № 3. P. 411−414.
- May T., van de Par S., Kohlrausch A. Noise-robust speaker recognition combining missing data techniques and universal background modeling // Audio, Speech, and Language Processing, IEEE Transactions on. 2012. V. 20. № 1. P. 108−121.
- Ortega-Garcia J., Gonzalez-Rodriguez J. Overview of speech enhancement techniques for automatic speaker recognition // Spoken Language. 1996. ICSLP-96. Proceedings. FourthInternationalConferenceon. 1996. V. 2. P. 929−932.
- Boll S. Suppression of acoustic noise in speech using spectral subtraction // Acoustics, Speech and Signal Processing, IEEE Transactions on. 1979. V. 27. № 2. P. 113−120.
- Nefedov V.I., Egorova E.V., Pugachev O.I., Gerasimov A.B. Primenenie cifrovojj obrabotki dlja filtracii shuma v zvukovykh signalakh // Nelinejjnyjj mir. 2009. T. 7. № 11. S. 869−871.
- Lim J.S., Oppenheim A.V. Enhancement and bandwidth compression of noisy speech // Proceedings of the IEEE. 1979. V. 67. P. 1586−1604.
- McAulay R.J., Malpass M.L. Speech enhancement using a soft-decision noise suppression filter // Acoustics, Speech and Signal Processing, IEEE Transactions on. 1980. V. 28. № 2. P. 137−145.
- Ephraim Y., Malah D. Speech enhancement using a minimum mean-square error short-time spectral amplitude estimator // Acoustics, Speech and Signal Processing, IEEE Transactions on. 1984. V. 32. № 6. P. 1109−1121.
- Scalart P., Filho J.V. Speech enhancement based on a priori signal to noise estimation // Acoustics, Speech and Signal Processing, IEEE Transactions on. 1996. V. 2. P. 629−632.
- Plapous C., Marro C., Scalart P., Mauuary L. A Two-Step Noise Reduction Technique // Acoustics, Speech, and Signal Processing. 2004. Proceedings. (ICASSP \'04). IEEEInternationalConferenceon. 2004. V. 1. P. 289−292.
- Novoselov S.A., Topnikov A.I., Savvatin A.I., Priorov A.L. Podavlenie shuma v rechevykh signalakh na osnove metoda nelokalnogo usrednenija // Cifrovaja obrabotka signalov. 2011. № 4. S. 23−28.
- Mysore G.J., Smaragdis P. A non-negative approach to semi-supervised separation of speech from noise with the use of temporal dynamics // Acoustics, Speech and Signal Processing (ICASSP). 2011 IEEEInternationalConferenceon. 2011. P. 17−20.
- Sprechmann P., Bronstein A., Bronstein M., Sapiro G. Learnable low rank sparse models for speech denoising // Acoustics, Speech and Signal Processing (ICASSP-2013). IEEEInternationalConferenceon. 2013. P. 136−140.
- Seltzer M., Raj B., Stern R. A Bayesian classifier for spectrographic mask estimation for missing feature speech recognition // Speech Communication. 2004. V. 43. P. 379−393.
- Roman N., Wang D. Pitch-based monaural segregation of reverberant speech // The Journal of the Acoustical Society of America. 2006. V. 120. P. 458−469.
- Roman N., Wang D., Brown G. Speech segregation based on sound localization // The Journal of the Acoustical Society of America. 2003. V. 114. P. 2236−2252.
- Renevey P., Drygajlo A. Detection of reliable features for speech recognition in noisy conditions using a statistical criterion // Proceedings of the Consistent and Reliable Acoustic Cues for Sound Analysis Workshop. 2001. P. 71−74.
- Hu Y., Loizou P. Techniques for estimating the ideal binary mask // Proceedings 11th International Workshop on Acoustic and Echo Noise Control. 2008.
- Varga A., Steeneken H.J.M. Assessment for automatic speech recognition: II. NOISEX‑92: A database and an experiment to study the effect of additive noise on speech recognition systems // Speech Communication. 1993. № 3. P. 247−251.