350 rub
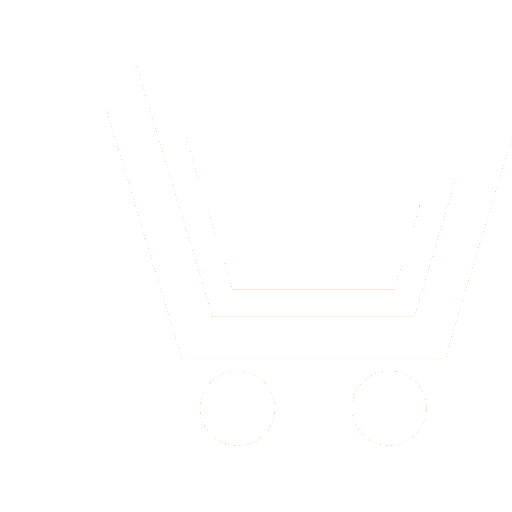
Journal Science Intensive Technologies №4 for 2014 г.
Article in number:
Automatic class recognition of aerial objects in shipborne optical-electronic systems
Keywords:
optical-electronic location systems
Johnson-s model
image preprocessing
pattern recognition
neural networks
multilayer perceptron
adaptive resonance
Authors:
V.V. Smirnov - Ph.D. (Eng.), Leading Programming Engineer, JSC "MSDB "Almaz-Antey?. E-mail: PCMNN@yandex.ru
Abstract:
Pattern recognition as a task consists of relating given object to a corresponding one of predefined patterns. Applied to shipborne data-collecting systems, this task arises in detection, identification and guidance procedures within radio-electronic and optical-electronic location stations (OELS).
Mathematical apparatus of neural networks (NN) is proposed as a basis for automatic recognition. Successful implementation can be estimated on its results correspondence to data, derived from the mathematical model of visual perception of objects by human OELS operator, called Johnson-s model. The basics of Johnson-s model applied to the current research aim are briefly described.
For the neural classifier practical implementation, the "SMART" model, based on the adaptive resonance theory and modified for object recognition in k-valued logics, as well as the classical neural network "multilayer perceptron" are proposed. Due to an important place in classification algorithms of feature set definition to characterize given objects, there is an overview of input data preprocessing methods for NNs, including image reaming and geometrical invariants calculation. Pros and cons of studied methods are reviewed.
And there is an overview of the experiments results over a developed NN-classifier model; they show its adequacy with the model-s results meeting the proposed Johnson-s criteria. Beyond that, the computational complexity of utilized algorithms is analyzed with the display of classification time measurement results for proposed classification system in field conditions.
Pages: 86-91
References
- Rozenblatt F. Principy nejrodinamiki. Perseptrony i teoriya mekhanizmov mozga. M.: Mir. 1965.
- Carpenter G.A., Grossberg S. Adaptive resonance theory. The handbook of brain theory and neural networks. Second edition. Cambridge, Massachusetts: MIT Press. 2002.
- Listratov A.I., Sidorov V.I. Korabel'nye optiko-e'lektronnye lokacionnye sistemy. M.: Izd-vo MIRE'A. 2007.
- Kotsiantis S.B., Kanellopoulos D., Pintelas P.E. Data preprocessing for supervised learning // IJCS. V. 1. № 2. 2006.
- Smirnov V.V. Nejronnaya set' SMART v zadache raspoznavaniya obrazov // Radioe'lektronnye sistemy - Centr «MNIIRE' «Al'tair» OAO GSKB «Almaz-Antej». 2011. № 1 (19). S. 49-56.
- Rajasekaran S., Vijayalakshmi Pai G.A. Image recognition using simplified Fuzzy ARTMAP augmented with a moment based feature extractor // International Journal of Pattern Recognition and Artificial Intelligence. V. 14. № 8 (2000). P. 1081-1095.
- Glumov N.I. Postroenie i primenenie momentnykh invariantov dlya obrabotki izobrazhenij v skol'zyashhem okne // Komp'yuternaya optika. 1995. Vyp. 14-15. Ch. 1. S. 46-54.
- Anisimov B.V., Kurganov V.D., Zlobin V.K. Raspoznavanie i cifrovaya obrabotka izobrazhenij. Uch. posobie dlya studentov vuzov. M.: Vysshaya shkola. 1983.
- Pre'tt U. Cifrovaya obrabotka izobrazhenij. Kn. 2. M.: Mir. 1982.
- Sidorov V.I., Smirnov V.V. O vozmozhnosti ispol'zovaniya nejrosetevykh algoritmov dlya avtomaticheskogo raspoznavaniya vozdushnykh celej v korabel'nykh optiko-e'lektronnykh sistemakh // Radioe'lektronnye sistemy - OAO «MNIIRE' «Al'tair». 2009. № 2 (16). S. 78-88.
- Yoshiko H., Shunji U., Taiko K. Evaluation of artificial neural network classifiers in small sample size situations // Pros. Int. It. Conf. Neural Networks (Nagoja, Oct. 25-29, 1993). P. 1731-1735.
- Smirnov V.V. Rol' vydeleniya priznakov v zadache avtomaticheskogo raspoznavaniya obrazov s ispol'zovaniem nejronnykh setej // Pribory i Sistemy. Upravlenie, Kontrol', Diagnostika. M.: OOO Izd-vo «NAUChTEKhIZDAT». 2010. № 11. S. 22-26.