350 rub
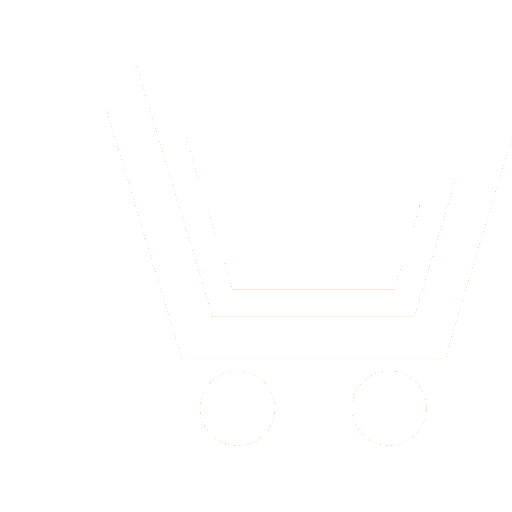
Journal Science Intensive Technologies №5 for 2013 г.
Article in number:
Stop criteria based on parameter convergence for stochastic gradient image registration
Keywords:
image registration
stochastic gradient estimation
Euclidian distance
interframe geometrical distortions
convergence
stabilization
stochastic gradient
Authors:
A.G. Tashlinskii, L.Sh. Biktimirov, P.V.Smirnov
Abstract:
Stochastic gradient procedures are good for registration of large images without a prior information because they provide high accuracy with high performance. Moreover, stochastic gradient procedures do not need prior parameter estimations of images to be registered and they can be used with images, which have smoothly fluctuated dissimilarities. However, optimal values are priory unknown and parameter converges are stochastic. This leads to need of choice of iteration number based on requirement accuracy for the worst case, but for better conditions this number is superfluous. Therefore, it is better to develop stop criteria for estimation process when parameters are close to optimal values (process stabilization). Optimal values themselves are unknown, but we can determine surroundings of optimal values by analyzing parameter convergences. Euclidian mismatch distance is proposed as integral characteristic instead of using parameters themselves. It is suggested to check parameter converge while procedure is evaluating. Different characteristics of Euclidian mismatch distance which can be calculated using sliding window are considered as stop criteria: the sum of absolute difference between the parameter and the expectation, variance, relative magnitude, the trend of changes. The hypothesis is accepted if the calculated value is less than the critical value in a specified number of iterations. Experimental studies have shown that the use of the criteria considered by stabilizing the parameter estimates for stop of stochastic gradient procedures of image registration parameter estimations allows to reduce the average time estimation several times while maintaining accuracy. It is shown that the considered criteria can also be used to refine the estimated parameters and for rejection breakdowns of stochastic gradient procedures (escape of estimation error beyond a given confidence interval).
Pages: 22-25
References
- Tashlinskijj A.G. Ocenivanie parametrov prostranstvennykh deformacijj posledovatelnostejj. Uljanovsk: Izd-vo UlGTU. 2000.
- Tashlinskijj A.G., Kaveev I.N., Voronov S.V. Metodika privjazki izobrazhenijj v uslovijakh intensivnykh pomekh // Radiotekhnika. 2012. № 9. S. 45-49.
- Cypkin JA.Z. Informacionnaja teorija identifikacii. M.: Nauka. Fizmatlit. 1995.
- Tashlinskii A.G. Pseudogradient Estimation of Digital Images Interframe Geometrical Deformations / Vision Systems: Segmentation & Pattern Recognition. Vienna, Austria : I‑Tech Education and Publishing. 2007. P. 465-494.
- Tashlinskii A.G., Safina G.L., Voronov S.V. Optimization of mismatch euclidean distance in evaluating interframe deformations of geometrical images // Pattern recognition and image analysis. 2011. V. 21. № 2. P. 335-338
- Welford B.P. Note on a Method for Calculating Corrected Sums of Squares and Products // Technometrics. 1962. V. 4. № 3. P. 419-420.