350 rub
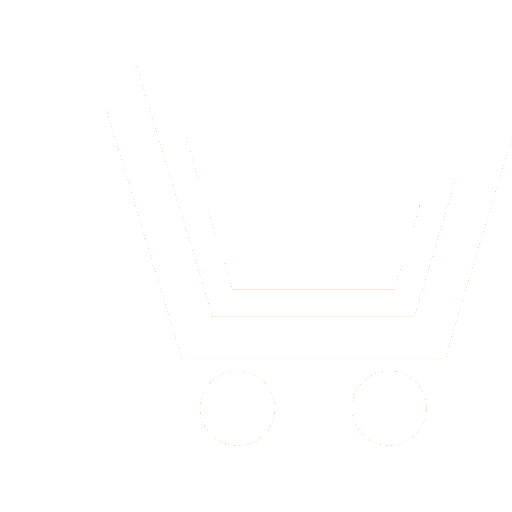
Journal Science Intensive Technologies №3 for 2012 г.
Article in number:
Modification of stochastic parameters models evaluation method of multidimensional hydrometeorological rows in their correlative and limited data conditions
Keywords:
multidimensional time sets
meteorological parameters
space and time correlation
regression equations
Authors:
M.G. Matveev, V.V. Michailov, M.E. Semenov, M.O. Gedzenko
Abstract:
At forecasting of the change meteorological values by methods of the applied statistics raw data in row of the events comfortable to present in the manner of tensor, which component correspond to space-temporary distribution corresponding to values. There is all basis to suppose that behavior of the temporary rows of importances in each node of the three-dimensional spatial net turns out to be interdependent, to the extent that that changes to behavior of one row will influence on behavior of the nearby rows. Such rows pertain to so named multivariate rows, at analysis which can be used vector stochastic models, for instance, vector ARSS(p, q)-model or her(its) simplified variants - a vector ARE(r) or boil(p, d)-models, where d - dimensionality of the multivariate row, p, q - an order to auto regressions and slithering average accordingly. However problem to identifications of these models in condition of the small quantity of the raw datas (the small samples) greatly complicates the analysis of the multivariate rows that requires searching for new approach to modeling of the process of the change the under investigation features.
The method of increasing happens to increase of adequacy of the stochastic models multivariate meteorological rows in condition their intercoupling and small archive samples by modification of the method of the estimation parameter these models, providing increase to stability of the procedure their parametric identification
Pages: 8-14
References
- Кендалл М., Стьюарт А. Многомерный статистический анализ и временные ряды. М.: Наука. 1976.
- Айвазян С.А., Мхитарян В.С. Прикладная статистика и основы эконометрики. М.: ЮНИТИ. 1998.
- Матвеев М.Г., Свиридов А.С., Алейникова Н.А. Модели и методы искусственного интеллекта: применение в экономике. М.: Финансы и статистика. 2008.
- Чураков Е.П. Прогнозирование экономических временных рядов. М.: Финансы и статистика. 2008.