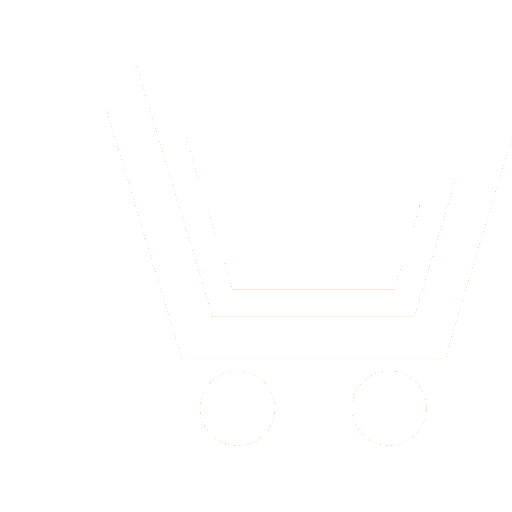
I.V. Sviridova1, M.V. Khoroshailova2, D.V. Lyalin3
1-3 FSBEI of HE “Voronezh State Technical University” (Voronezh, Russia)
1 ri-ss-ka@mail.ru; 2 pmv2205@mail.ru; 3 dmitrylialin95@gmail.com
Problem statement. Deep neural networks are successfully used in channel coding to improve the quality of decoding. However, modern neural channel decoders cannot achieve high decoding performance and low complexity at the same time. To overcome this problem, it is necessary to develop a decoder with a double residual neuron. By integrating residual input and residual learning into the neural channel decoder architecture, it will significantly improve decoding performance while maintaining low complexity.
Goal. Development of a decoder architecture with a dual residual neural network, which provides high decoding performance in channel coding with low model complexity and computational costs.
Results. The simulation results show that the proposed decoder with a double residual neural network provides a significant increase in decoding performance. Compared to a neural decoder based on a trust propagation algorithm, a decoder with a dual residual neural network provides an additional 0.5-1.8 dB encoding gain compared to different channel codes. Compared to the hypergraph neural decoder, which has the best error correction capability among all existing neural channel decoders, the dual residual neural network decoder also provides similar or even better decoding performance for different channel codes.
Practical significance. The results obtained can be used to improve decoding efficiency and improve performance in channel coding.
The work was carried out with the financial support of the Ministry of Science and Higher Education of the Russian Federation within the framework of the state assignment "youth laboratory" No. FZGM-2024-0003.
Sviridova I.V., Khoroshailova M.V., Lyalin D.V. High-performance decoding using deep neural networks. Radiotekhnika. 2024. V. 88. № 7. P. 21−25. DOI: https://doi.org/10.18127/j00338486-202407-04 (In Russian)
- Horoshajlova M.V. Arhitektura kanal'nogo kodirovanija na osnove PLIS dlja 5G besprovodnoj seti s ispol'zovaniem vysokourovnevogo sinteza. Vestnik Voronezhskogo gosudarstvennogo tehnicheskogo universiteta. 2018. T. 14. № 2. S. 99-105 (in Russian).
- Safaldin M., Otair M., Abualigah L. Improved Binary Gray Wolf Optimizer and SVM for Intrusion Detection System in Wireless Sensor Networks. J. Ambient. Intell. Humaniz. Comput. 2021. № 12. Р. 1559–1576.
- Yun-Jiang Wang, Barry C Sanders, Bao-ming Bai, Xin-mei Wang. Enhanced Feedback Iterative Decoding of Sparse Quantum Codes. IEEE Trans. Inf. Theory. 2012. № 58. Р. 1231–1241.
- Horoshajlova M.V. Arhitektura dlja stohasticheskih LDPC-dekoderov c ispol'zovaniem jeffektivnoj ploshhadi kristalla na osnove PLIS. Vestnik Voronezhskogo gos. tehnich. un-ta. 2018. T. 14. № 1. S. 95-100 (in Russian).
- Bashkirov A.V., Horoshajlova M.V. Algoritmy nizkoj slozhnosti dekodirovanija i arhitektura dlja nedvoichnyh nizkoplotnostnyh kodov. Radiotehnika. 2016. № 6. S. 10-14 (in Russian).
- Bashkirov A.V., Muratov A.V., Horoshajlova M.V., Sitnikov A.V., Ermakov S.A. Nizkoplotnostnye kody maloj moshhnosti dekodirovanija. Radiotehnika. 2016. № 5. S. 32-37 (in Russian).
- Thi Bao Nguyen T., Nguyen Tan T., Lee H. Efficient QC-LDPC Encoder for 5G New Radio. Electronics. 2019. № 8. Р. 668.