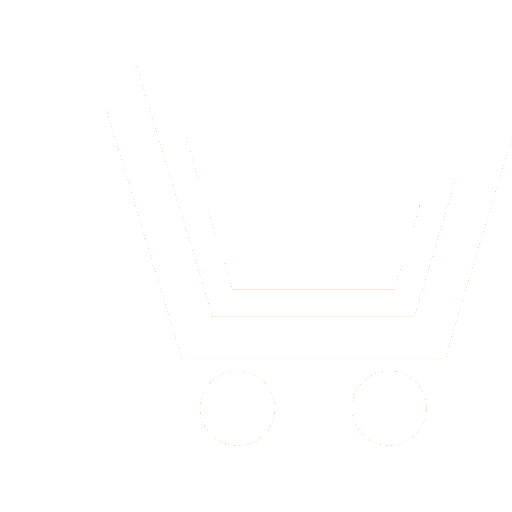
A.A. Korobkov1, I.A. Safiullin2, I.P. Ashaev3, A.K. Gaysin4, A.F. Nadeev5
1-5 Kazan National Research Technical University n.a. A.N. Tupolev – KAI (Kazan, Russia)
1 aakorobkov@kai.ru; 2 iasafiullin@kai.ru; 3 ipashaev@kai.ru; 4 akgaysin@kai.ru; 5 afnadeev@kai.ru
The performance of modern heterogeneous Radio Access Networks (RANs) can be improved by introducing new methods and algorithms for network resource management. In particular, user mobility information can be used to control RAN resources. To determine user mobility, information about the variation of the Signal to Interference + Noise Ratio (SINR) between base stations and users can be collected and analyzed. These data are multi-linear and can be represented as tensors to extract information from them. However, for the tensors decomposition, it is necessary to know the order of the multi-linear model or the rank of the tensors.
Inspired by the LaRGE (LineAr Regression of Global Eigenvalues) method, we propose a novel method for the model order estimation of the multi-linear data based on the analysis of the Complimented Global Eigenvalues (CGE) profile. We have denoted our method as Enhanced LaRGE (EnLaRGE). We have compared the EnLaRGE method with the classical methods AIC, MDL and their multi-linear versions, as well as with the M-EFT and N-D EFT methods. EnLaRGE shows good performance for the tensors with small one of the tensors dimensions.
EnLaRGE method is implemented for the automatic model order estimation of multi-linear data received from the Network Simulator 3 with RAN Intelligent Controller (RIC). The results show the high precision of the multi-linear model order estimation in comparison with state-of-the-art methods. The estimated model order can be used for user mobility classification and improving heterogeneous RANs performance.
This research was funded by Russian Science Foundation, grant number 23-69-10084, 318, https://rscf.ru/project/23-69-10084/
Korobkov A.A., Safiullin I.A., Ashaev I.P., Gaysin A.K., Nadeev A.F. Model order estimation method for user mobility classification
in heterogeneous RAN systems. Radiotekhnika. 2024. V. 88. № 1. P. 44−58. DOI: https://doi.org/10.18127/j00338486-202401-05 (In Russian)
- Mezhuev A.M., Korennoj A.V., Sturov D.L., Rodionov D.V. Ocenka jeffektivnosti informacionnogo obmena v cifrovyh setjah svjazi nazemno-vozdushnogo bazirovanija: algoritmicheskoe i programmnoe obespechenie. Radiotehnika. 2023. T. 87. № 9. S. 138-148. DOI: https://doi.org/10.18127/j00338486-202309-12 (in Russian).
- Sviridova I.V. Horoshajlov R.N., Sviridova I.V., Ljalin D.V. Analiz metodov MIMO dlja 5G i posledujushhih tehnologij: preimushhestva i nedostatki. Radiotehnika. 2023. T. 87. № 8. S. 100-104. DOI: 10.18127/j00338486-202308-16.
- Lerner I.M., Hajrullin A.N. Teorija razreshajushhego vremeni v oblasti sistem shirokopolosnogo dostupa. Algoritm ocenki dzhittera, obuslovlennogo peredachej dannyh, i propusknoj sposobnosti s polinomial'nym vremenem ispolnenija. T-Comm: Telekommunikacii i transport. 2023. T. 17. № 5. S. 48-57 (in Russian).
- Lerner I.M., Fajzullin R.R., Hajrullin A.N., Shushpanov D.V., Il'in V.I., Rjabov I.V. Povyshenie udel'noj propusknoj sposobnosti kak fundamental'naja problema teorii svjazi. Strategija razvitija v postshennonovskuju jepohu. Ch. 1. Retrospektivnyj obzor metodov priema i obrabotki signalov v chastotno-selektivnyh kanalah svjazi pri skorostjah peredachi informacii vyshe skorosti Najkvista. Uspehi sovremennoj radiojelektroniki. 2023. T. 77. № 1. S. 37-50. DOI: https://doi.org/10.18127/j20700784-202301-02 (in Russian).
- Lerner I.M., Fajzullin R.R., Hajrullin A.N., Shushpanov D.V., Il'in V.I., Rjabov I.V. Povyshenie udel'noj propusknoj sposobnosti kak fundamental'naja problema teorii svjazi. Strategija razvitija v postshennonovskuju jepohu. Ch. 2. Retrospektivnyj obzor metodov priema i obrabotki signalov v chastotno-selektivnyh kanalah svjazi pri nalichii mezhsimvol'nyh iskazhenij. Uspehi sovremennoj radiojelektroniki. 2023. T. 77. № 2. S. 16–33. DOI: https://doi.org/10.18127/j20700784-202302-02 (in Russian).
- Lerner I.M., Fajzullin R.R., Shushpanov D.V., Il'in V.I., Rjabov I.V., Hajrullin A.N. Povyshenie udel'noj propusknoj sposobnosti kak fundamental'naja problema teorii svjazi. Strategija razvitija v postshennonovskuju jepohu. Ch. 3. Retrospektivnyj obzor metodov ocenki propusknoj sposobnosti chastotno-selektivnyh kanalov svjazi pri nalichii pri nalichii mezhsimvol'nyh iskazhenij i ispol'zovanii FMn-n i AFMn-N-signala. Uspehi sovremennoj radiojelektroniki. 2023. T. 77. № 3. S. 24–33. DOI: https://doi.org/10.18127/j20700784-202303-02 (in Russian).
- Lerner I.M. Capacity estimation method with linear computational complexity of narrow-band ionospheric decameter channels with PSK-N-signals. T-Comm. 2023. V. 17. № 9. P. 55-66. DOI: 10.36724/2072-8735-2023-17-9-55-66.
- Lerner I.M. Metod ocenki propusknoj sposobnosti uzkopolosnyh ionosfernyh dekametrovyh kanalov svjazi s linejnoj vychislitel'noj slozhnost'ju pri ispol'zovanii mnogopozicionnyh fazomanipulirovannyh signalov. T-Comm: Telekommunikacii i transport. 2023. T. 17.
№ 9. S. 55-66 (in Russian). - Lerner I.M. Sposob povyshenija propusknoj sposobnosti radiotehnicheskoj sistemy s posledovatel'noj peredachej informacii v uzkopolosnyh KV-kanalah svjazi na baze teorii razreshajushhego vremeni. Vestnik Povolzhskogo gosudarstvennogo tehnologicheskogo universiteta. Ser. Radiotehnicheskie i infokommunikacionnye sistemy. 2023. № 1(57). S. 6-23. DOI: 10.25686/2306-2819.2023.1.6.
- Lerner I.M., Fajzulin R.R. Rjabov I.V. Optimizirovannyj algoritm ocenki propusknoj sposobnosti kanalov svjazi, funkcionirujushhih na baze teorii razreshajushhego vremeni. Radiotehnika. 2022. T. 86. № 4. S. 91-109. DOI: https://doi.org/10.18127/j00338486-202204-13 (in Russian).
- Rjabov I.V., Tokar' M.S. Slepaja kadrovaja sinhronizacija v sisteme peredachi s differencial'nym prostranstvenno-vremennym blokovym kodirovaniem. Radiotehnika. 2021. T. 85. № 4. S. 99-107. DOI: 10.18127/j00338486-202104-11 (in Russian).
- Tokar' M.S. Algoritm dekodirovanija nizkoj slozhnosti dlja sistem peredachi differencial'nogo prostranstvenno-vremennogo blokovogo kodirovanija. Radiotehnika. 2021. T. 85. № 4. S. 89-98. DOI: 10.18127/j00338486-202104-10 (in Russian).
- O-RAN Alliance, O-RAN use cases and deployment scenarios. Technical Report. February 2020. [Online].
- Ullah R., Marwat S. N. K., Ahmad A. M., Ahmed S., Hafeez A., Kamal T., Tufail M. A maching learning approach for 5G SINR prediction. Electronics. 2020. V. 9. № 10. Р. 1660.
- Bennis M., Perlaza S. M. Decentralized cross-tier interference mitigation in cognitive femtocell networks. Proc. 2011 IEEE international conference on communications (ICC). 2011. Р. 1–5.
- Gannapathy V. R., Nordin R., Abu-Samah A., Abdullah N. F., Ismail M. An Adaptive TTT Handover (ATH) Mechanism for Dual Connectivity (5G mmWave-LTE Advanced) during Unpredictable Wireless Channel Behavior. Sensors. 2023. V. 23. № 9. Р. 4357.
- Chirkov O.N., Romashhenko M.A., Bobylkin I.S. Jeffektivnyj metod modelirovanija sistem besprovodnoj svjazi. Radiotehnika. 2023.
T. 87. № 8. S. 110-115. DOI: 10.18127/j00338486-202308-18 (in Russian). - Nechaev Ju.B., Maljutin A.A. Matrichnye razlozhenija dlja formirovanija i obrabotki signalov v MIMO sistemah svjazi v kanalah s MSI. Radiotehnika. 2012. № 8. S. 40-45 (in Russian).
- Sidiropoulos N., Giannakis G., Bro R. Blind PARAFAC receivers for DS-CDMA systems. Transactions on Signal Processing. 2000. V. 48. № 3. Р. 810–823.
- De Lathauwer L., Castaing J. Tensor-based techniques for the blind separation of DS–CDMA signals. Signal Processing. 2007. V. 87.
№ 2. Р. 322–336. - Nion D., De Lathauwer L. An enhanced line search scheme for complex-valued tensor decompositions. Application in DS-CDMA. Signal Processing. 2008. V. 88. № 3. Р. 749–755.
- Kolda T.G., Bader B.W. Tensor Decompositions and Applications. SIAM Review. 2009. V. 51. № 3. Р. 455–500.
- Akaike H., A new look at the statistical model identification. IEEE Trans. Autom. Control. 1974. V. 19. № 6. Р. 716–723.
- Schwarz G. Estimating the dimension of a model. Ann. Statist. 1978. V. 6. № 2. Р. 461–464.
- L. da Costa J.P.C., Haardt M., Roemer F., Del Galdo G. Enhanced model order estimation using higher-order arrays. Proc. 2007 Conference Record of the Forty-First Asilomar Conference on Signals, Systems and Computers. Nov 2007. Р. 412–416.
- L. da Costa J.P.C., Roemer F., Haardt M., T. de Sousa Jr R. Multidimensional model order selection. EURASIP Journal on Advances in Signal Processing. 2011.
- Quinlan A., Barbot J.-P., Larzabal P., Haardt M. Model order selection for short data: An exponential fitting test (EFT). EURASIP Journal on Advances in Signal Processing. 2006. V. 2007. Р. 1–11.
- Korobkov A.A. и др. Multi-dimensional model order estimation using LineAr Regression of Global Eigenvalues (LaRGE) with applications to EEG and MEG recordings. 2020 28th European Signal Processing Conference (EUSIPCO). 2021. Р. 1005–1009.
- Korobkov A.A. и др. Robust Multi-Dimensional Model Order Estimation Using LineAr Regression of Global Eigenvalues (LaRGE). IEEE Transactions on Signal Processing. 2022. V. 70. Р. 5751–5764.
- Boas B.V., Zirwas W., Haardt M. Deep-LaRGE: Higher-Order SVD and Deep Learning for Model Order Selection in MIMO OFDM
Systems. 26th International ITG Workshop on Smart Antennas and 13th Conference on Systems, Communications, and Coding. 2023. Р. 1–6. - Badeau R., Boyer R. Multilinear singular value decomposition for structured tensors. SIAM J. Matrix Anal. Appl. 2008. V. 30.
№ 3. Р. 1008–1021.