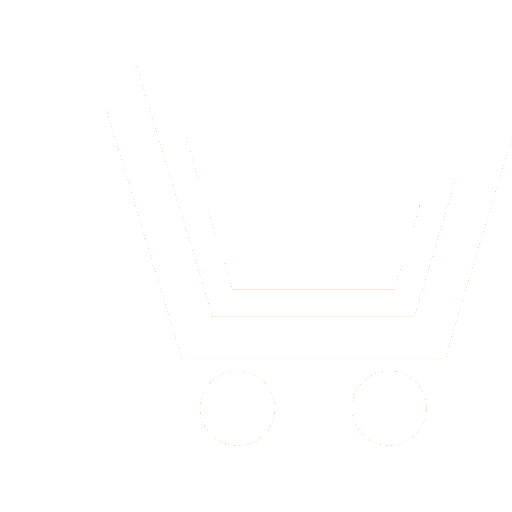
V.P. Fedosov1, R.R. Ibadov2, S.R. Ibadov3
1-3 Institute of Radio Engineering Systems and Control, Southern Federal University (Taganrog, Russia)
Formulation of the problem. Currently, unmanned aerial vehicles (UAVs) are actively used to solve cross-industry tasks, such as photo and video filming of the surrounding space, remote analysis of surrounding objects, cargo delivery, monitoring the state of transport routes and mining complexes. UAVs are also used in emergency areas where the presence of people is associated with a threat to life. Since good orientation in space is necessary in all of these areas, UAV orientation in urban infrastructure is of great practical importance. For example, for accident-free driving of UAVs, determining the distance to obstacles (image depth maps) and constructing the trajectory of their flight is of great importance, which is relevant for precise UAV orientation in urban infrastructure.
Target. Propose a modified method for post-processing the image depth map for the problems of accident-free driving of UAVs in urban infrastructure.
Results. Existing methods and algorithms for converting from 2D signals to 3D signals in image analysis and display systems are considered. A study of the method of post-processing of the image depth map was carried out and its modification was proposed. To evaluate the effectiveness of the developed post-processing method, a comparative analysis of depth maps for various test images obtained by the original method and its modification was performed. Root-mean-square errors are obtained for the proposed and original post-processing methods for the considered test images.
Practical significance. The presented modified image depth map post-processing method makes it possible to build sharper image depth maps, which provides more accurate UAV orientation in urban infrastructure.
Fedosov V.P., Ibadov R.R., Ibadov S.R. Modified depth map post-processing method for problems of accident-free UAV driving in urban infrastructure. Radiotekhnika. 2023. V. 87. № 9. P. 113−123. DOI: https://doi.org/10.18127/j00338486-202309-10 (In Russian)
- Wang X., Pan Y., Lun D.P.K. Stereoscopic image reflection removal based on Wasserstein Generative Adversarial Network. IEEE International Conference on Visual Communications and Image Processing (VCIP). IEEE. 2020. P. 148-151.
- Lu S.P., You J., Ceulemans B., Wang M., Munteanu A. Synthesis of Shaking Video Using Motion Capture Data and Dynamic 3D Scene Modeling. 25th IEEE International Conference on Image Processing (ICIP). IEEE. 2018. P. 1438-1442.
- Yu Z., Mizuno K., Asada A., Tabeta S., Fujimoto Y., Shimada T. Research on Fish Classification by Using High-Resolution Acoustic Video Camera-ARIS and 3-D Fish Model Simulator. OCEANS-MTS/IEEE Kobe Techno-Oceans (OTO). IEEE. 2018. P. 1-4.
- Liu Z., Li X., Luo P., Loy C.C., Tang X. Deep learning markov random field for semantic segmentation. IEEE transactions on pattern analysis and machine intelligence. 2017. V. 40. № 8. P. 1814-1828.
- Yan S., Wu C., Wang L., Xu F., An L., Guo K., Liu Y. Ddrnet: Depth map denoising and refinement for consumer depth cameras using cascaded cnns. Proceedings of the European conference on computer vision (ECCV). 2018. P. 151-167.
- Godard C., Mac Aodha O., Brostow G.J. Unsupervised monocular depth estimation with left-right consistency. Proceedings of the IEEE conference on computer vision and pattern recognition. 2017. P. 270-279.
- Fu H., Gong M., Wang C., Batmanghelich K., Tao D. Deep ordinal regression network for monocular depth estimation. Proceedings of the IEEE conference on computer vision and pattern recognition. 2018. P. 2002-2011.
- Fan Z., Ohashi T., Hayashi T. Make 3D Cable Model from Single RGB Image. 10th International Congress on Advanced Applied Informatics (IIAI-AAI). IEEE. 2021. P. 482-485.
- Zhao H., Shi J., Qi X., Wang X., Jia J. Pyramid scene parsing network. Proceedings of the IEEE conference on computer vision and pattern recognition. 2017. P. 2881-2890.
- Karsch K., Liu C., Kang S.B. Depth transfer: Depth extraction from videos using nonparametric sampling. Dense Image Correspondences for Computer Vision. Springer, Cham. 2016. P. 173-205.
- Xia K., Yin H., Zhang Y. Deep semantic segmentation of kidney and space-occupying lesion area based on SCNN and ResNet models combined with SIFT-flow algorithm. Journal of medical systems. 2019. V. 43. № 1. P. 1-12.
- Ibadov R.R., Fedosov V.P., Ibadov S.R. Vosstanovlenie defektov i slepoj zony na izobrazhenijah podstilajushhej poverhnosti dlja bortovyh radiolokacionnyh sistem kartografirovanija na osnove doplerovskogo obuzhenija lucha. Izvestija Juzhnogo federal'nogo universiteta. Tehnicheskie nauki. 2020. № 6(216). S. 4-52 (In Russian).
- Ibadov R.R., Fedosov V.P., Ibadov S.R. The method of spatial-temporal reconstruction of dynamic images based on a geometric model with contour and texture analysis. IOP Conference Series: Materials Science and Engineering. IOP Publishing. 2021. V. 1029. № 1. P. 012093.
- Fedosov V.P., Ibadov R.R., Ibadov S.R. Restoration of the Lost Area of the Underlying Surface Image Using the Saliency Map. 2021 Radiation and Scattering of Electromagnetic Waves (RSEMW). IEEE. 2021. P. 447-450.
- Didyuk A. Ya., Ivankin E. F., Ponkin V. A. Estimation of the accuracy of measuring object parameters from an image. Radiotekhnika. 2010. Т. 74. № 8. S. 90-95 (In Russian).