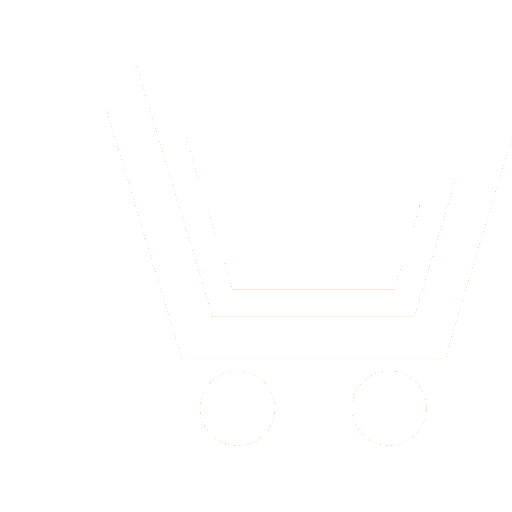
M.E. Gurbatov1, V.Ya. Litnovsky2
1-2 PJSC Radiofizika (Moscow, Russia)
1 Moscow Institute of Physics and Technology (National Research University) (Dolgoprudny, Russia)
The article is devoted to the study of an urgent problem related to the analysis of the effectiveness of neural network algorithms for trajectory processing of radar information. The purpose of the article is to compare the effectiveness of using neural network algorithms for analyzing radar information with algorithms based on the Kalman filter. With the help of modeling, dependencies were obtained that demonstrate the quality of the work of the algorithms under consideration for processing radar information. A comparative analysis of the algorithms was made in terms of the accuracy of estimating the parameters of the target movement both in areas without changing the type of movement, and in areas with a change in the type of movement. The practical significance of the results obtained lies in the fact that they can be used to optimize systems for processing radar monitoring information based on neural network modeling.
Gurbatov M.E., Litnovsky V.Ya. Analysis of the effectiveness of neural network algorithms for trajectory processing of radar information. Radiotekhnika. 2023. V. 87. № 3. P. 31−39. DOI: https://doi.org/10.18127/j00338486-202303-03 (In Russian)
- Li X.R. A Survey of Maneuvering Target Tracking. Part V: Multiple-Model Methods. IEEE Trans on aerospace and electric systems. 2005. V. 41. № 4. P. 1255–1321.
- Bar-Shalom Y. Estimation with Applications to Tracking and Navigation: Theory, Algorithms, and Software. New York: John Wiley & Sons. 2001. P. 558
- Sychev M.I. Traektornaja obrabotka radiolokacionnoj informacii na osnove uproshhennyh mnogomodel'nyh fil'trov. Jelektrosvjaz'. 2016. № 10. S. 32-37 (In Russian).
- Kuz'min S.Z. Cifrovaja radiolokacija. Vvedenie v teoriju. Kiev: Izd-vo «KVShh». 2000. 428 s. (In Russian).
- Blackman S.S. Multiple-target tracking with radar applications. Artech House. 1986. 449 р.
- Konovalov A.A. Osnovy traektornoj obrabotki radiolokacionnoj informacii. Ch. 2. SPB: LJeTI. 2014. 180 s. (In Russian).
- Farber V.E. Osnovy traektornoj obrabotki radiolokacionnoj informacii v mnogokanal'nyh RLS: Ucheb. posobie. M.: MFTI. 2005. 160 s. (In Russian).
- Li X.R., Jilkov V.P. A Survey of Maneuvering Target Tracking: Part I. Dynamic Models. Proceedings of SPIE. 2000. P. 24.
- Farina A. Cifrovaja obrabotka radiolokacionnoj informacii. Soprovozhdenie celej. M.: Radio i svjaz'. 1993. 320 s. (In Russian).
- Kalman R.E. A new approach to linear filtering and prediction problems. Transactions of the ASME. Journal of Basic Engineering (Series D). 1960. P. 35-45.
- Kalman R.E. New Results in Linear Filtering and Prediction Theory. Transactions of the ASME. Journal of Basic Engineering (Series D). 1961. P. 95–108.
- Балакришнан А. В. Теория фильтрации Калмана. М.: Мир. 1988. 168 с.
- Julier S.J. A New Extension of the Kalman Filter to Nonlinear Systems. Proc. of SPIE. July 1997. P. 12.
- Julier S.J. A New Method for the Nonlinear Transformation of Means and Covariances in Nonlinear Filters. IEEE Transactions on Automatic Control. 2000. V. 45. P. 477–482.
- Kazarinov Ju.M. Radiotehnicheskie sistemy. M.: Izdatel'skij centr «Akademija». 2008. 592 s. (In Russian).
- Yuan X. Models and Algorithms for Tracking Target with Coordinated Turn Motion. Hindawi Publishing Corporation Mathematical Problems in Engineering. V. 2014. P. 10.
- Blom H.A.P. The Interacting Multiple Model algorithm for systems with Markovian switching coefficients. IEEE Tr. on Automatic Control. 1988. V. 33. P. 780-783.
- Besada J.A. Design of IMM filter for radar tracking using evolution strategies. IEEE Transactions on Aerospace and Electronic Systems. 2005. V. 41. Is. 3. P. 1109-1122.
- Danilov S.N. Algoritm prognoza koordinat vozdushnyh ob#ektov dlja obespechenija funkcionirovanija sistemy napravlennoj svjazi na osnove sistem so sluchajnym izmeneniem struktury. Vestnik TGTU. 2009. T. 15. № 3. S. 530-539 (In Russian).
- Hajkin S. Preimushhestva i ogranichenija obuchenija metodom obratnogo rasprostranenija. Nejronnye seti. 2006. S. 304–314 (In Russian).
- Parhomenko S.S. O sokrashhenii vremeni obrabotki bol'shogo kolichestva dannyh nejronnymi setjami metodom Levenberga - Markvardta. Impeks. 2014. S. 80–83 (In Russian).
- Sousa C. Neural network learning by the levenberg-marquardt algorithm with Bayesian regularization (part 1). 2009. URL: http://crsouza.blogspot.com/2009/11/neural-networklearning-by-levenberg_18.html.
- Transtrum M.K., Sethna J.P. Improvements to the levenberg-marquardt algorithm for nonlinear least-squares minimization. Journal of Computational Physics. 2012
- Verem'ev V.I., Kovalev D.A. Modelirovanie algoritmov traektornogo soprovozhdenija radiolokacionnoj celi na osnove apparata nejronnyh setej. Izvestija vuzov Rossii. Ser. Radiojelektronika. 2010. 74 s. (In Russian).
- Kiselev V.Ju. Ocenka kachestva traektornoj obrabotki v radiolokacionnyh sistemah upravlenija vozdushnym dvizheniem: avtoref. diss. … kand. tehn. nauk: 05.12.14. SPb: SPGUAP. 2017. 200 s. (In Russian).
- Farber V.E. Analiz harakteristik algoritmov ocenki jeffektivnosti ajerodinamicheskogo tormozhenija vhodjashhih v at-mosferu kosmicheskih ob’ektov. Radiotehnika. 2007. № 10. S. 81−87 (In Russian).