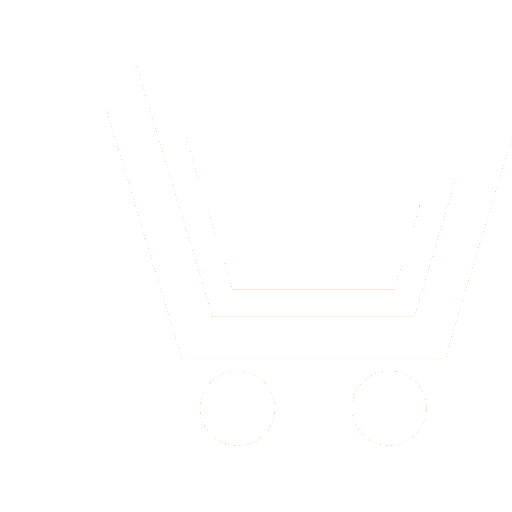
V.А. Ivanov, V.V. Maksimov, K.D. Galev
Statement of the problem. To reduce the cost of accumulating a training sample, it is advisable to use a signal synthesis model with properties similar to the general population, but at the same time differing in numerical indicators of features in the time and frequency domain. The use of neural network models of signal synthesis will ensure the representativeness of the new general population and provide conditions for improving the quality of functioning of the technical means when environmental conditions change. Goal. Creating prerequisites for improving the accuracy of the obtained estimates of property parameters from signal sources of various physical principles of operation (electrodynamics, magnetometric and fiber-optic sensing elements for interferometers and reflectometers).
Results. The solution of the problem of signal synthesis (modeling) is obtained, which allows for an in-depth study of the latent noninterpreted feature space formed by the neural network of the "auto-encoder" type based on the Kullback-Leibler divergence. The research will make it possible to create promising mobile and stationary technical means used in the protection of state and other objects of any form of ownership.
Practical significance. The resulting mathematical model can be used at the design stage of advanced security equipment.
Ivanov V.А., Maksimov V.V., Galev K.D. Graphics Accelerators as a training tool for neural networks signal synthesis of various physical principles of action. Radiotekhnika. 2021. V. 85. № 2. P. 27−32. DOI: 10.18127/j00338486202102-04 (In Russian).
- Barskij A.B. Nejronnye seti: raspoznavanie, upravlenie, prinjatie reshenij. M.: Finansy i statistika. 2004. 176 s. (In Russian).
- Galev K.D., Dvojris L.I. Algoritm adaptivnoj nastrojki poroga obnaruzhenija. Radiotehnika. 2018. № 2. S. 10-12 (In Russian).
- Galev K.D., Dvojris L.I., Kobzar' M.V. Jeffektivnyj metod otbora informativnyh priznakov v zadachah raspoznavanija. Radiotehnika. 2017. № 1. S. 53-55 (In Russian).
- Dvojris L.I., Galev K.D. Vybor optimal'nogo sochetanija vychislitel'nogo jadra i kompleksa algoritmov pri razrabotke sistem obnaruzhenija i raspoznavanija dvizhushhihsja ob’ektov po sejsmicheskim signalam. Radiotehnika. 2019. № 2. S. 44-51. DOI: 10.18127/j00338486-201902-09 (In Russian).
- Dvojris L.I., Ivanov V.A., Galev K.D. Kriterij jenergeticheskogo obnaruzhenija ob’ektov na osnove analiza parametrov zakona raspredelenija centrirovannyh i normirovannyh fonovyh signalov. Radiotehnika. 2019. № 2. S. 34-39. DOI: 10.18127/j00338486201902-07 (In Russian).
- Abdul Dakkak, Tom Wickham-Jones, and Wen-mei Hwu. The design and implementation of the Wolfram Language compiler. In Proceeding of the 18th ACM/IEEE International Symposium of Code Generation and Optimization (CGO 2020). Association for Computing Machinery. New York, NY, USA. 2020. P. 212-228. DOI: https://doi.org/10.1145/33688263377913
- Bishop C. Pattern Recognition and Machine Learning. 2006. P. 55.
- Chun-Yu Shei, Pushkar Ratnalikar, and Aruh Chauhan. Automating GPU computing in MatLab. In Proceeding of the international conference on Supercomputing (ICS ‘11). Association for Computing Machinery. New York, NY, USA. 2011. P. 245-254. DOI: https://doi.org/10.1145/1995896.1995936
- Kulback S., Leibler R.A. On information and sufficiency. The Annals of Mathematical Statistics. 1951. V. 22. № 1. P. 79-86.
- Furber Steve. ARM System-on-chip Architecture (2nd. ed.). Addison-Wesley Longman Publishing Co., Inc. USA. 2000.