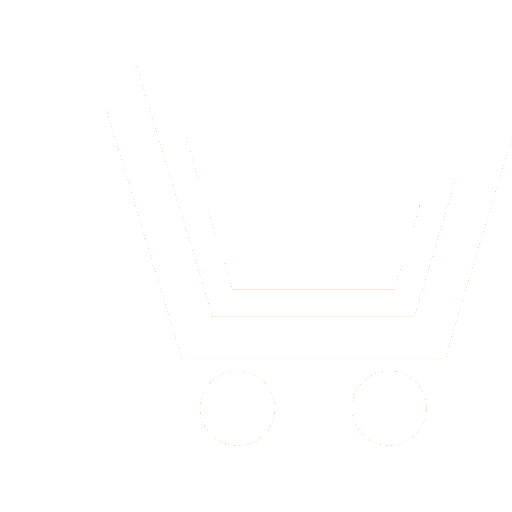
E.A. Kirsanov¹, V.A. Tatarintsev²
1-2 MESC AF «N.E. Zhukovsky and Y.A. Gagarin Air Force Academy»
Traditional algorithms of the cluster analysis are inapplicable for the solution of a problem of automatic classification of a set of estimates of coordinates of sources of a radio emission on not set number of classes for the purpose of identification of groups of territorially combined radio emission sources because of the heterogeneity of space of signs caused by difference of kovariatsionny matrixes of errors of calculation of coordinates of sources of a radio emission in various points of the plane. Therefore their modification is required.
Purpose is carrying out the comparative analysis of efficiency of the modified clustering algorithms on the basis of the self-organized neural network and a method of «average shift» taking into account heterogeneity of space of signs when processing a set of estimates of coordinates of sources of a radio emission for goniometric multiposition radio system.
The modified algorithms of processing of a set of the estimates of coordinates of sources of a radio emission received in multiposition radio systems on the basis of automatic classification of objects on not set number of classes the self-organized neural network and by method of «average shift» considering the heterogeneity of space of signs caused by difference of kovariatsionny matrixes of errors of calculation of coordinates in various points of the plane are considered. Results of the comparative analysis of efficiency of algorithms of processing of a set of estimates of coordinates of sources of a radio emission for goniometric multiposition radio system are given. Use of the modified method of «average shift» will provide increase in probability of the correct definition of number of classes more than for 30% in comparison with a traditional method of «average shift» and more than for 60% in comparison with the selforganized neural network of competitive training.
Kirsanov E.A., Tatarintsev V.A. Comparative analysis of algorithms of automatic classification of coordinate information in multiposition radio systems on the basis of the self-organized neural network and method of average shift. Radioengineering. 2021. V. 85. № 1. P. 92−68. DOI: 10.18127/j00338486-202101-08. (
- Afanasev V.I., Kirsanov E.A., Sirota A.A. Sravnitelnyi analiz vozmozhnostei cheloveka-operatora i avtomaticheskikh algoritmov klassifikatsii koordinatnoi informatsii v rezhime samoobucheniya. Radiotekhnika. 2000. № 10. S. 79−85. (in Russian)
- Radzievskii V.G., Sirota A.A. Teoreticheskie osnovy radioelektronnoi razvedki. M.: Radiotekhnika. 2004. 352 s. (in Russian)
- Kirsanov E.A., Sirota A.A. Obrabotka informatsii v prostranstvenno-raspredelennykh sistemakh radiomonitoringa: statisticheskii i neirosetevoi podkhody. M.: FIZMATLIT. 2012. 344 s. (in Russian)
- Kirsanov E.A. Vyyavlenie grupp territorialno-sovmeshchennykh istochnikov radioizlucheniya v mnogopozitsionnykh radiosistemakh na osnove samoorganizuyushcheisya neironnoi seti. Informatsionno-izmeritelnye i upravlyayushchie sistemy. 2007. № 10. S. 70−73. (in Russian)
- Kirsanov E.A., Tatarintsev V.A. Klasterizatsiya mnozhestva otsenok koordinat istochnikov radioizlucheniya v mnogopozitsionnykh radiosistemakh metodom srednego sdviga. Sb. materialov XX Mezhdunar. nauchno-metodicheskoi konf. «Informatika: problemy, metody, tekhnologii» (pod red. A.A. Zatsarinnogo, D.N. Borisova) Voronezhskii gosudarstvennyi universitet 13−14 fevralya 2020 g. Voronezh: Izd-vo «Nauchno-issledovatelskie publikatsii» (OOO «Velborn»). 2020. S. 1040−1046. (in Russian)
- Kirsanov E.A., Tatarintsev V.A. Algoritmy vyyavleniya grupp territorialno sovmeshchennykh istochnikov radioizlucheniya modifitsirovannym metodom srednego sdviga. Sb. trudov XXVI Mezhdunar. nauchno-tekhnich. konf. «Radiolokatsiya, navigatsiya, svyaz». 29 sentyabrya – 1 oktyabrya 2020 g. Voronezhskii gosudarstvennyi universitet (AO «Kontsern «Sozvezdie»). Voronezh: Izdatelskii dom VGU. 2020. V 6 tomakh. T. 5. S. 282−290. (in Russian)
- Xu L., Krzyzak A., Oja E. Rival penalized competitive learning for clustering analysis, RBF net and curve detection. IEEE Trans. Neural Networks. 1993. № 4. P. 636−649.
- Fukunaga K., Hostetler L. The estimation of the gradient of a density function, with applications in pattern recognition. IEEE Transactions on information theory. 1975. № 1. P. 32−40.
- Comaniciu D., Meer P. Mean shift: A robust approach toward feature space analysis. IEEE Transactions on Pattern Analysis & Machine Intelligence. 2002. № 5. P. 603−619.