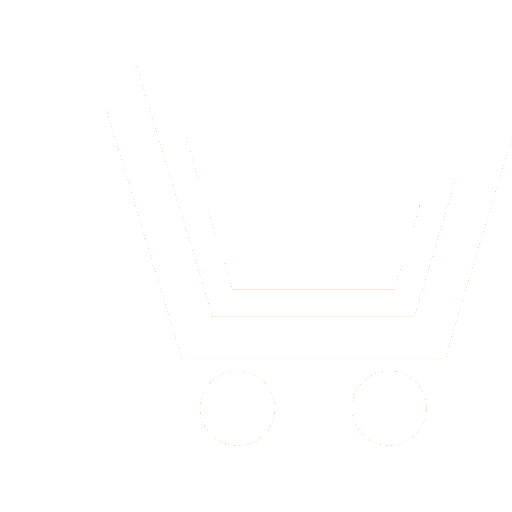
A.V. Kvasnov – Ph.D.(Eng.), Associate Professor,
Peter The Great St. Petersburg Polytechnic University
E-mail: Kvasnov_AV@spbstu.ru
The article discusses the application of the Bayesian programming methodology for recognition and classification of radio emission sources. The mathematical model of the Bayesian program involves assigning a family of probability distributions according to wellknown features contained in the training set. Based on the training sample, correlations between classes and types of objects are estimated and the probabilities of their identification are calculated. The architecture of the Bayesian program assumes the existence of criteria for assessing the representativeness of the sample, the target task of the technique (recognition, classification, etc.).
The research was carried out on based classifier of radar stations, containing 345 objects of 16 types. The following parameters were used as features of the received radio signal: station frequency range, pulse width, pulse repetition period, radar rotation frequency. The following assumptions were made: the signals do not contain intrapulse modulation, the law of feature distribution of signals is uniform or Poisson.
The results of simulation showed that the recognition of an object in automatic mode is possible with PREC > 0.15, and the classification of an object with PCLASS > 0.32. A type I error will be for recognized objects PREC_ER = 0.014…0.307, but for classified PCLASS_ER = 0.021…0.393. Therefore, it can be argued that the recognition of an object allows you to classify it. At the same time, the classification of an object does not mean that it will be recognized.
The results obtained in the article showed the consistency of the Bayesian programming technique under given assumptions. At the same time, a number of problems remain that should be addressed. First, this is the extension of the classifier and the use of a signal model with intrapulse modulation.
- Kouemou G. (ed.) Radar Technology. InTech. 2010. ISBN 978-953-307-029-2.
- Dudczyk J. A method of feature selection in the aspect of specific identification of radar signals. Bulletin of the polish academy of sciences technical sciences. 2017. V. 65. № 1. P. 113.
- Richard G. Wiley. ELINT: The Interception and Analysis of Radar Signals. Artech House. 2006.
- Kawalec A., Owczarek R., Dudczyk J. Data modeling and simulation applied to radar signal recognition. Molecular and Quantum Acoustics. 2005. V. 26. № 7. P. 165.
- Zhutian Yang, Wei Qiu, Hongjian Sun, Arumugam Nallanathan. Robust Radar Emitter Recognition Based on the Three-Dimensional Distribution Feature and Transfer Learning. Sensors. 2016. № 16 (3). P. 289.
- Adzhemov S.S., Tereshonok M.V., Chirov D.S. Raspoznavanie vidov tsifrovoi modulyatsii radiosignalov s ispolzovaniem neironnykh setei. VMU. Fizika. Astronomiya. 2015. № 1. S. 23.
- Dudczyk J. Radar Emission Sources Identification Based on Hierarchical Agglomerative Clustering for Large Data Sets. Journal of Sensors. 2016. V. 2016. Article ID 1879327. 9 p.
- Zhu J., Zhao Y., Tang J., Xu J. Automatic recognition of radar signals based on time-frequency image shape character. Defence Science Journal. 63(3). 308.
- Chirov D.S., Kandaurova E.O. Analiz metodov raspoznavaniya vidov mezhimpulsnoi modulyatsii signalov radiolokatsionnykh sredstv. T-Comm: Telekommunikatsii i transport. 2019. T. 13. № 3. S. 15. (in Russian)
- Jaynes Edwin T. Probability Theory: The Logic of Science. Cambridge University Press. ISBN 0-521-59271-2.
- Robert Christian & Marin Jean-Michel. Bayesian Core: A Practical Approach to Computational Bayesian Statistics. 2007.
- Barber D. Bayesian Reasoning and Machine Learning. Cambridge University Press. 2012. ISBN: 978-0521518147.
- Finn B. Jensen. Bayesian Networks and Springer. 2nd edition. 2007.
- Kvasnov A., Shkodyrev V., Arsenyev D. Method of recognition the radar emitting sources based on the naive Bayesian classifier. WSEAS transactions on systems and control. 2019. V. 14. P. 112.
- Kvasnov A.V., Shkodyrev V.P., Arsenev D.G. Vysokotochnye modeli raspoznavaniya ob'ektov na osnove rekurrentnykh baiesovskikh algoritmov. Materialy konf. «Upravlenie v aerokosmicheskikh sistemakh» (UAS-2018). SPb: AO «Kontsern «TsNII «Elektropribor». 2018. S. 90. (in Russian)
- Database of radars. http://www.radartutorial.eu. Accessed 20 May 2019.
- Svid-vo o registratsii programmy dlya EVM. № 2018618004. Opublikovano 27.08.18. Programma klassifikatsii istochnikov radioizlucheniya po radiotekhnicheskim priznakam. Kvasnov A.V., Shkodyrev V.P. Reestr programm dlya EVM. 1 s. (in Russian)