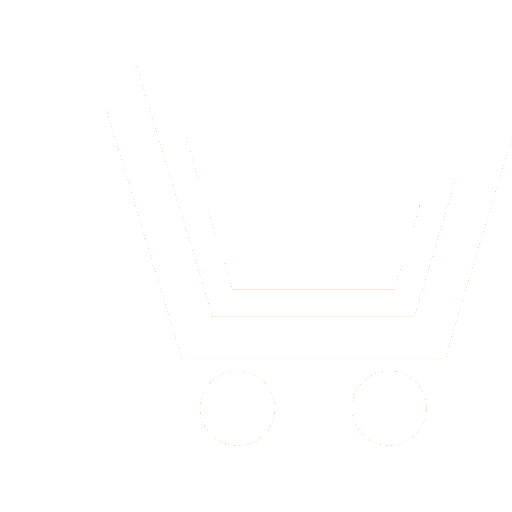
V.Ya. Prorok – Dr.Sc.(Eng.), Professor,
Mozhaysky Military Space Academy (Saint Petersburg)
E-mail: val_prorok@mail.ru
A.V. Timofeev – Post-graduate Student,
Mozhaysky Military Space Academy (Saint Petersburg)
E-mail: alexonwork@gmail.com
S.G. Vorona – Ph.D.(Eng.), Associate Professor, Lecturer,
Department of Missle Attack Warning Means, Mozhaysky Military Space Academy (Saint Petersburg)
E-mail: vorona_1953@mail.ru
On the basis of a mathematical model, the article proposes to trace the process of becoming a specialist using educational tools. The purpose of the article is to analyze the time and cost costs of forming a highly professional specialist depending on the level of development of his abilities.
The investigated mathematical model allows you to track dynamic changes in the process of training. Obtaining quantitative estimates that meet the requirements of reliability and reliability at the first stages of the analysis is impossible, however, as a result of this analysis, cause-effect relationships and mechanisms of phenomena to be observed are manifested. In this case, special attention is paid to one of the most important tasks in modeling the process of becoming a specialist in determining the values of the coefficient of learning speed. In most cases, specialist training is carried out according to a previously developed program, which implies a strict time limit for training and is a constant value. Therefore, we can say that the achievement of the required level of training becomes possible only with a high learning rate. The problem of assessing the level of knowledge that a student has mastered in a given period of time, and as a consequence, the subsequent determination of the coefficient of learning speed, can be classified as poorly formalized.
The presence of uncertainty implies the advisability of using fuzzy inference. Initially, the data that is input to the system is determined. They will be measured variables that are elements of the learning process. Such a value, in particular, can be the time of training. Next, data is generated at the system output. These values correspond to output variables, such is an indicator of learning speed.
The algorithm for calculating the learning speed will include the following steps: creating a rule base for the formation of a fuzzy inference system; fuzzification of source data; logical conclusion; composition and defuzzification.
The results of the study will consist in building a learning model using educational tools, taking into account such features as the ability of the knowledge received by students to self-organize and create new knowledge. This approach makes it possible to predict the process of formation of knowledge and skills of a student in a professional field of activity. A characteristic feature of the proposed model is the use of the transformational theory of learning and the calculation of the coefficient of learning speed using fuzzy inference.
The proposed model of the learning process will allow an analysis of the time and cost of forming a highly professional specialist, depending on the level of development of his abilities, the features used in training educational tools and training strategies of a specific specialist, based on an analysis of the initially existing professional-important qualities and competencies. This, in turn, will make it possible to correct existing training programs, optimize knowledge, highlight logical information blocks that are to be studied, accelerate the educational process without reducing quality indicators, reducing labor costs and eliminating the «human factor» in the assessment procedure.
- Paul D. Thorn, Gerhard Schurz. Meta-inductive prediction based on Attractivity Weighting: Mathematical and empirical performance evaluation. Journal of Mathematical Psychology. 2018. DOI: https://doi.org/10.1016/j.jmp.2018.12.006.
- Atkinson R., Bauer G., Kroters Z. Vvedenie v matematicheskuyu teoriyu obucheniya: per. s angl. M.: Mir. 1969. (in Russian)
- Siddhatra Thyagarajan, Thangasamy Nambirajan, Ganeshkumar Chandirasekaran. An Empirical Analysis of Training Facilities in Micro-SmallMedium Enterprises (MSME) for Self-Help Groups (SHG). Ushus-Journal of Business Management, 2019, DOI: https://doi.org/10.12725/ujbm.47.2.
- Zade L.A. Razmytye mnozhestva i ikh primenenie v raspoznavanii obrazov i klaster-analize: Klassifikatsiya i klaster. M.: Mir. 1986. (in Russian)
- Prorok V.Ya. Postroenie sistem soprovozhdeniya protsessov professionalnogo stanovleniya i deyatelnosti voennykh spetsialistov. SPb.: VKA (filial). 2004. 154 s. (in Russian)
- Xi Zhang, Yuan Zhang, Yongqiang Sun, Miltiadis Lytras, Patricia Ordonez de Pablos. Exploring the effect of transformational leadership on individual creativity in e-learning: a perspective of social exchange theory. Studies in Higher Educatin. 2017. DOI: https://dx.doi.org/10.1080/03075079.2017.1296824.
- William F. Crittenden, Isabella K. Biel, William A. Lovely. Embracing Digitalization: Student Learning and New Technologies. Journal of Marketing Education. 2018. DOI: https://doi.org/10.1177/0273475318820895.
- Venda V.F. Sistemy gibridnogo intellekta: Evolyutsiya, psikhologiya, informatika. M.: Mashinostroenie. 1990. (in Russian)
- Prorok V.Ya. Osnovy postroeniya i organizatsii adaptivnykh sistem professionalnogo otbora. SPb.: Lan. 2003. 330 s. (in Russian)
- Bagretsov S.A. Primenenie metodov mnogomernoi razmytoi klassifikatsii v diagnostike slozhnykh sistem. Priborostroenie. 1995. № 9, 10. S. 74−76. (in Russian)
- Dervis Karaboga, Ebubekir Kaya. Adaptive network based fuzzy inference system (ANFIS) training approaches: a comprehensive survey. Artificial Intelligence Rewiew. 2018. DOI: https://doi.org/10.1007/s10462-017-9610-2.
- Abraham Varghese, Jincy S. George. A Continious Assessment Strategy Using Fuzzy Logic. Learning Strategies and Constructionism in Modern Education Settings. 2018. DOI: https://doi.org/10.4018/978-1-5225-5430-1.ch005.
- Mehyuddin K M Nasution, Indra Aulia, Marischa Elveny. Data.. Journal of Physics: The 3rd International Conference on Computing and Applied Informatics. 2018. DOI: https://doi.org/10.1088/1742-6596-1235/1/012110.
- Shaily Thaker, Viral Nagori. Analysis of Fuzzification Process in Fuzzy Expert System. Procedia Computer Science. 2018. DOI: https://doi.org/10.1016/j/procs.2018.05.047.
- O. Yazdanbaksh, S. Dick. A systematic review of complex fuzzy set and logic. Fuzzy sets and systems. 2017. DOI: https://doi.org/10.1006/j/fss.2017.01.010.
- Samuel Lopez, Antonio A. Marquez, Francisco A. Marquez, Antonio Peregrin. Evolutionary Design of Linguistic Fuzzy Regression Systems with Adaptive Defuzzification in Big Dqata Environmets. Cognitive Computation. 2018. DOI: https://doi.org/10.1007/s12559-019-09632-4.