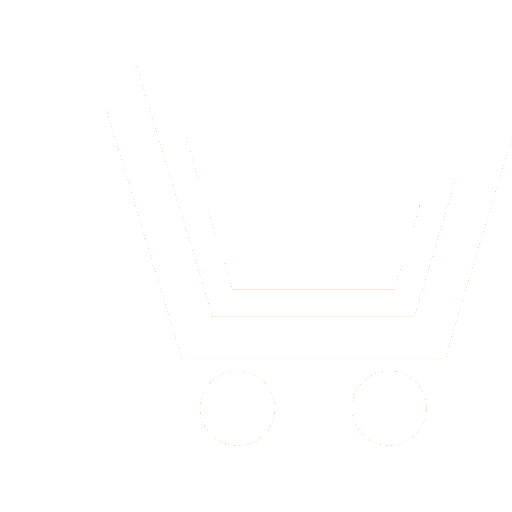
O.V. Osipov 1, A.A. Diyazitdinova 2
1,2 Povolzhskiy State University of Telecommunications and Informatics (Samara, Russia)
1 o.osipov@psuti.ru; 2 miftaxovaaa@mail.ru
The improving quality of the television signals is an important task at video surveillance systems. The quality of television signals is image resolution. This property defines the possibility of discerning two object points in the images. The current methods of interpolation images allow increasing scale image, but not allow increasing the resolution. The radical way of improving the quality of television signals has been provided by the multi-camera system. The specificity of those systems is applying several cameras with a different focus of the lens. Applying those systems allow switching between video data with different zoom when the image is scaled. On the one hand, this way guarantees to save information about all protected area; on the other hand, this way allows exploring some region of interest. The accurate switching between video data of the different cameras is provided by the superposition of images with various zoom. The overview of the method for superposition the images with different scales identifies the perspective way for solving the current task. It is applying two methods, which include the method of feature points and method of affine images superposition. The feature points are local extremum and they have defined offsets between regions of analyzing images. The method of affine images superposition allows estimating the scale and rotates. For this aim, the images are shown from the Cartesian coordinate system to the log-polar system. The result of matching the feature points is defined by the maximum correlation coefficient. After matching the superposition parameters are estimated as homography parameters. The developed method is applied for real television signals of a multi-camera system. The method has shown accurate zoom of images and improving the quality of television signals.
Osipov O.V., Diyazitdinova A.A. Signal superposition for improving quality of television video surveillance system. Radiotekhnika. 2020. V.84. № 12(23). P. 72−. DOI: 10.18127/j00338486-202012(23)-08 (In Russian).
- Fleet David J., Jepson Allan D. Computation of component image velocity from local phase information. International Journal of Computer Vision. 1990. № 1. P. 77–104.
- Hidetoshi M., Atsushi N., Kazutoshi K. Determining image flow from multiple frames based on the continuity equation. Technology Repts Yamaguchi University. 1991. № 5. P. 387–397.
- Mjasnikov E.V. Opredelenieparametrovgeometricheskihtransformacijdljasovmeshhenijaportretnyhizobrazhenij. Komp'juternajaoptika. 2007. T. 31. № 3. S. 77-82(In Russian).
- Wolberg G., Zokai S. Robust image registration using log-polar transform. Processing of IEEE International Conference on Image Processing. 2000. V. 1. P. 493‒496. DOI:10.1109/ICIP.2000.901003.
- Kuglin C.D., Hines D.C. The phase correlation image alignment method. Processing International Conference on Cybernetics and Society. 1975. Р. 163-165.
- Goshin E.V., Kotov A.P., FursovV.A. Dvuhjetapnoeformirovanieprostranstvennogopreobrazovanijadljasovmeshhenijaizobrazhenij. Komp'juternajaoptika. 2014. T. 38. № 4. S. 886-891 (In Russian).
- Novikov A.I., et al. The Contour Analysis and Image Superimposition Problem in Computer Vision Systems. Pattern Recognition and Image Analysis. 2015. V. 25(1). P. 73-80. DOI: 10.1134/S1054661815020194.
- Efimov A.I., Novikov A.I. Algoritmpojetapnogoutochnenijaproektivnogopreobrazovanijadljasovmeshhenijaizobrazhenij. Komp'juternajaoptika. 2016. T. 40. № 2. S. 258-265.DOI: 10.18287/2412-6179-2016-40-2-258-265(In Russian).
- Loeckx D., et al. Nonrigid image registration using free-form deformations with a local rigidity constraint. Medical Image Computing and Computer-Assisted Intervention (MICCAI 2004). Springer Berlin Heidelberg, 2004. P. 639-646.
- Ke Y., Sukthankar R. PCA-SIFT: A more distinctive representation for local image descriptors. Computer Vision and Pattern Recognition. 2004. V. 2. P. 506-513.
- Harris С., Stephens M. A combined corner and edge detector. Proceedings of the 4th Alvey Vision Conference. 1988. P. 147–151.