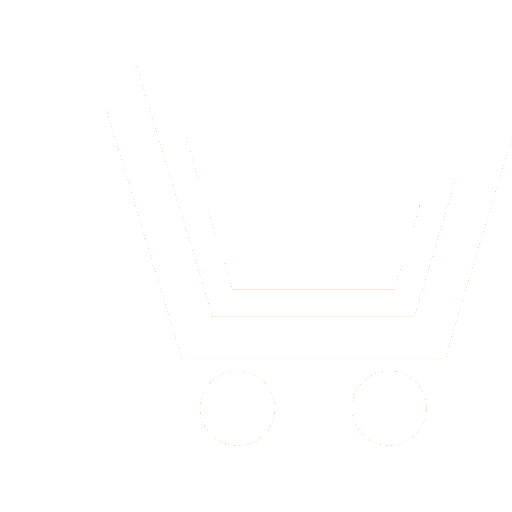
A.A. Sychugov – Ph.D.(Eng.), Associate Professor,
Head of Department «Information Security», Tula State University
E-mail: xru2003@list.ru
A.P. Anchishkin – Student,
Department «Information Security», Tula State University
E-mail: alexan999@yandex.ru
D.V. Chernov – Assistant,
Department «Information Security», Tula State University; Head of the Information Security Sector, JSC ADC (Tula) E-mail: cherncib@gmail.com
The purpose of the work is a formalized description of the methodology for determining the list of current threats to information security, the implementation of which can lead to a violation of the normal operation of multi-level distributed automated process control systems, as well as a set of information security measures required to minimize the risks of the implementation of current threats. This necessitates the development of new effective solutions. The article describes the method of analysis of the state of technological processes as part of the monitoring system, based on the method of single-class classification OneClass SVM. The existing methods of analysis of the state of technological processes are considered. The most effective were the methods of machine learning, which showed a high percentage of accuracy. On the basis of known models of technological processes, a new model of technological process, not previously found in open sources, is proposed as an infinite data flow with changing properties with a large number of parameters. An experiment is described in which the effectiveness of the proposed method is studied and its results are presented. Its solvency is shown, it is noted that it can be used in modern systems of monitoring the state of technological processes to identify malfunctions and threats to information security.
- Maidanovich O.V. Intellektualnye tekhnologii avtomatizirovannogo monitoringa slozhnykh tekhnicheskikh obieektov. Trudy SPIIRAN. 2013. № 6. S. 201−216. (In Russian).
- Plotnikova A.G. Analiz parametrov tekhnologicheskogo protsessa s primeneniem kontrolnykh kart. Sb. nauchnykh trudov po materialam VIII Mezhdunar. nauchno-praktich. konf. «Sovremennye innovatsii: fundamentalnye i prikladnye issledovaniya». Problemy nauki. 2018. S. 16−18. (In Russian). 26. KHeifets M.L., Vasilev A.S., Klimenko S.A., Lyubodrag Tanochiv Tekhnologicheskoe nasledovanie parametrov kachestva materiala i poverkhnosti detali. Materialy VII Mezhdunar. nauchno-praktich. konf. «Innovatsii v mashinostroenii». Kemerovo. 2015. S. 307−312. (In Russian).
- Malkov M.V., Oleinik A.G., Fedorov A.M. Modelirovanie tekhnologicheskikh protsessov: Metody i opyt. Trudy Kolskogo nauchnogo tsentra RAN (Apatity). 2010. № 3. S. 93−101. (In Russian).
- Datev I.O., Putilov V.A., Fedorov A.M. Metod i tekhnologiya sistemno-dinamicheskogo modelirovaniya nagruzki na regionalnye informatsionno-kommunikatsionnye seti. Trudy Instituta sistemnogo analiza RAN. Pod red. Yu.S. Popkova, V.A. Putilova. M.: Knizhnyi dom «LIBROKOM». 2008. T. 39. S. 220−231. (In Russian).
- Oleinik A.G., Shalatonova A.A. Agregirovannaya matematicheskaya model protsessov razdeleniya mineralnykh komponentov. Informatsionnye tekhnologii v regionalnom razvitii: kontseptualnye aspekty i modeli. Apatity: Izd-vo KNTs RAN. 2002. S. 71−74. (In Russian).
- Rudnev D.O., Sychugov A.A. Metod analiza sostoyaniya avtomatizirovannykh sistem upravleniya tekhnologicheskimi protsessami. Izvestiya TulGU. Tekhnicheskie nauki. 2017. № 10. S. 165−173. (In Russian).
- Bifet A., Kirkby R. Data stream mining. A practical approach. Waikato: The University of Waikato. 2017. № 1.
- Rajeev T., Santosh K. A Quick Review of Data Stream Mining Algorithms. Imperial Journal of Interdisciplinary Research. 2016. V. 2. № 7. P. 870−873.
- Mining data streams. Mining of Massive Datasets. Ed. by J. Leskivec, A. Ullman, D. Jeffrey. Cambridge: Cambridge University Press. 2017. № 2. P. 131−162.
- Rohit B., Agarwal S. Stream Data Mining:Platforms, Algrithms, Performance Evaluators and Research Trends. International Journal of Database Theory and Application. 2016. V. 9. № 9. P. 201−218.
- Fong S., Wong R., Vasilakos A. Accelerated PSO Swarm Search Feature Selection for Data Stream Mining Big Data. IEEE Transactions on Services Computing. 2015. P. 1−1.
- Yusuf B., Reddy P. Mining Data Streams using Option Trees. International Journal of Computer Network and Information Security. 2012. V. 4. № 8. P. 49−54.
- Moya M., Koch M. and Hostetler L. One-class classifier networks for target recognition applications. Proc. of World Congress on Neural Networks. Portland, OR: International Neural Network Society. INNS. 1993. P. 797−801.
- Ritter G. and Gallegos M. Outliers in statistical pattern recognition and an application to automatic chromosome classification. Pattern Recognition Letters. 1997. № 18. P. 525−539.
- Roberts S., Tarassenko L., Pardey J. and Siegwart D. A validation index for artificial neural networks. Proc. of Int. Conference on Neural Networks and Expert Systems in Medicine and Healthcare. 1994. P. 23−30.
- Koch M., Moya M., Hostetler L. and Fogler R. Cueing, feature discovery and one-class learning for synthetic aperture radar automatic target recognition. Neural Networks. 1995. № 8(7/8). P. 1081−1102.
- Scholkopf B., Platt J.C., Shawe-Taylor J., Smola A.J., Williamson R.C. Estimating the support of a high-dimensional distribution. Neural Computation. 2001. № 13. P. 1443−1471.
- Pavel A. Turkov, Olga Krasotkina, Vadim Mottl, Sychugov A.A. Feature Selection for Handling Concept Drift in the Data Stream Classification. Proc. of 12th International Conference «Machine Learning and Data Mining in Pattern Recognition». MLDM 2016. New York, NY, USA. July 16−21, 2016. Lecture Notes in Computer Science 9729. Springer 2016. P. 614−629.
- Albert D. Shieh, David F. Kamm Ensembles of One Class Support Vector Machines. MCS 2009. LNCS 5519. Springer-Verlag. Berlin. Heidelberg. 2009. P. 181−190.
- Tax D.M.J., Duin R.P.W. Support vector domain description. Pattern Recognition Letters 1999. № 20. P. 1191−1999.
- Tax D.M.J. One-class classification. Ph.D thesis. Delft University of Technology. 2001.
- Tax D.M.J., Duin R.P.W. Combining one-class classifiers. Ed. By J. Kittler, F. Roli. MCS 2001. LNCS. Springer. Heidelberg. 2001. V. 2096. P. 299−308.
- Tax D.M.J., Juszczak P. Kernel whitening for one-class classification. Ed. by S.-W. Lee, A. Verri. SVM 2002. LNCS. Springer. Heidelberg. 2002. V. 2388. P. 40−52.
- https://sites.google.com/a/uah.edu/tommy-morris-uah/ics-data-sets.