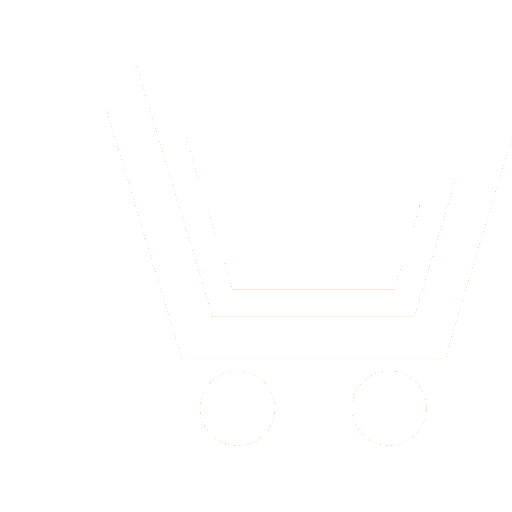
V.N. Klyachkin – Dr.Sc.(Eng.), Professor, Department «Applied Mathematics and Informatics», Ulyanovsk State Technical University
E-mail: v_kl@mail.ru
Yu.E. Kuvayskova – Ph.D.(Eng.), Associate Professor, Department «Applied Mathematics and Informatics», Ulyanovsk State Technical University
E-mail: u.kuvaiskova@mail.ru
D.A. Zhukov – Post-graduate Student, Department «Applied Mathematics and Informatics», Ulyanovsk State Technical University
E-mail: zh.dimka17@mail.ru
To recognize the health state of the technical facility can be used methods of machine learning, using a binary classification: objects are subdivided into serviceable and faulty. Classification quality depends on several factors: quantity and quality of the original sample, method of machine learning, source separation method of sampling for training and reference parts. To improve the quality of its detection equipment are encouraged to use the aggregate methods that combine multiple base classifiers in different ways-on average, median or by means of a vote. The effectiveness of this approach has been confirmed by numerical tests of real objects.
- Klyachkin V.N., Kuvajskova Yu.E., Buby’r’ D.S. Prognozirovanie sostoyaniya ob’‘ekta s ispol’zovaniem sistem vremenny’x ryadov // Radiotexnika. 2015. № 6. S. 45−47.
- Montgomery D.C. Introduction to Statistical Quality Control. New York: John Wiley and Sons. 2009. 754 r.
- Ryan T.P. Statistical Methods for Quality Improvement. New York: John Wiley and Sons. 2011. 687 r.
- Klyachkin V.N. Statisticheskie metody’ v upravlenii kachestvom: komp’yuterny’e texnologii. M.: Finansy’ i statistika, INFRA-M. 2009. 304 s.
- Witten I.H., Frank E. Data Mining: Practical Machine Learning Tools and Techniques. San Francisco: Morgan Kaufmann Publishers. 2005. 525 r.
- Sokolov E.A. Mashinnoe obuchenie. URL = http://wiki.cs.hse.ru/ Mashinnoe _obuchenie. _1/2016_ 2017.
- Zhukov D.A., Klyachkin V.N. Diagnostika ispravnosti texnicheskogo ob’‘ekta s ispol’zovaniem paketa Matlab // Trudy’ Mezhdunar. nauchnotexnich. konf. «Perspektivny’e informaczionny’e texnologii». Samara: Izd-vo Samarskogo nauchnogo czentra RAN. 2018. S. 55−57.
- Klyachkin V.N., Kuvajskova Yu.E., Alekseeva V.A. Statisticheskie metody’ analiza danny’x. M.: Finansy’ i statistika. 2016. 240 s.
- Klyachkin V.N., Shunina Yu.S. Sistema oczenki kreditosposobnosti zaemshhika i prognozirovaniya vozvrata kreditov // Vestnik komp’yuterny’x i informaczionny’x texnologij. 2015. № 11 (137). S. 45−51.
- Klyachkin V.N., Kuvayskova Yu.E., Zhukov D.A. The Use of Aggregate Classifiers in Technical Diagnostics, Based on Machine Learning // CEUR Workshop Proceedings. V. 1903. Data Science. Information Technology and Nanotechnology. 2017. P. 32−35.
- Kuvayskova Y.E. The Prediction Algorithm of the Technical State of an Object by Means of Fuzzy Logic Inference Models // Procedia Engineering «3rdInternational Conference «Information Technology and Nanotechnology (ITNT 2017)». 2017. S. 767−772.