350 rub
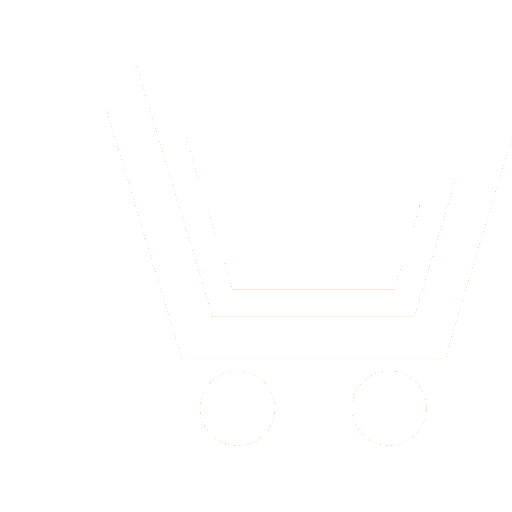
Journal Radioengineering №5 for 2016 г.
Article in number:
The use of compressive sampling in subsurface holographic radiolocation
Keywords:
subsurface radiolocation
holographic radar
automatic data acquisition
sparse sensing
compressive sampling
Authors:
M.A. Chizh - Post-graduate Student, Research Assistant, Bauman Moscow State Technical University. E-mail: mchizh@rslab.ru
Abstract:
In the last decade, the sparse or compressed sensing theory has been developed which allows reconstructing images of objects from a reduced number of measurements in comparison with traditional methods. The main objective of compressive sensing is to reconstruct a signal on the base of a grid with spatial or time sampling greater than required by the Nyquist theorem.
The experimental setup developed in the Remote sensing laboratory at BMSTU was used for experimental studies of the sampling in-fluence on the resolution of the test object reconstructed image. This setup consists of a vector network analyzer, an antenna, a tripod, flexible feeders to connect the antenna, and a mechanical two-coordinate scanner and allows specifying the spacing between samples, testing different scanning parameters with the help of the PC-side software. Data acquisition is performed automatically by moving objects near the antenna line by line.
A complex data processing algorithm was developed for improving the resolution and background-object contrast enhancement. To evaluate the effectiveness of the developed processing algorithm, two procedures of obtaining radar images were considered: one in-cluding the processing stages and another without them. For imaging in both the procedures the conventional back-propagation method for radar images processing was applied.
For the three specified values of sampling interval, at the frequency of 22.5 GHz complex holograms were obtained by scanning the sample with the mentioned experimental setup, then the processing of the signal frequency spectrum was performed. The obtained results show that the above processing procedure significantly improves image quality, increases the contrast of the object against the background, effectively suppresses reconstruction algorithm artifacts. It should also be noted that despite the considerable un-dersampling the form of the object can be clearly distinguished.
A further step to adaptiveness and flexibility in the development of the proposed sparse sensing technique will be the rejection of rigid geometry scanning when data acquisition is performed on an equidistant grid. Further implementation of the compressive sensing concept will enable an adaptive data acquisition with more image detail in the areas of interest and minimum amount of noninformative data.
Pages: 134-141
References
- Sergey I. Ivashov, Vladimir V. Razevig, Timothy D. Bechtel, Igor A. Vasiliev, Lorenzo Capineri, Andrey V. Zhuravlev. Microwave Holography for NDT of Dielectric Structures // Proceedings of the IEEE International Conference on Microwaves, Communications, Antennas and Electronic Systems (COMCAS 2015). Tel-Aviv, Israel, 2−4 November 2015. IEEE. doi: 10.1109/COMCAS.2015.7360372.
- Chan W.L., Moravec M., Baraniuk R., Mittleman D. Terahertz imaging with compressed sensing and phase retrieval // Optics Letters. 2008. 33. P. 974−976.
- Emmanuel Candès, Terence Tao. Decoding by linear programming // IEEE Trans. on Information Theory. 2005. V. 51. № 12. P. 4203−4215.
- Xiaolin Liu, Chong Luo, Feng Wu. Formulating binary compressive sensing decoding with asymetrical property // Data Compression Conference. 2011. P. 213−222.
- Zoltan Szabo, Barnabas Poczos, Andras Lorincz. Collaborative Filtering via Group-Structured Dictionary Learning // Latent Variable Analysis and Signal Separation (LVA/ICA). Tel-Aviv. Israel. 12−15 March 2012. V. 7191 of LNCS. P. 247−254.
- M.H. Mahoor, M. Zhou, K. Veon, S.M. Mavadati J. Cohn. Facial Action Unit Recognition with Sparse Representation // IEEE International Conference on Automatic Face & Gesture Recognition (FG 2011). March 2011. P. 336−342.
- Martin F. Schiffner, Georg Schmitz. Compensating the combined effects of absorption and dispersion in plane wave pulse-echo ultrasound imaging using sparse recovery // IEEE Int. Ultrasonics Symp. (IUS). July 2013. Prague. P. 573−576.
- Leyuan Fang, Shutao Li,; Ryan P. McNabb, Qing Nie, Anthony N. Kuo, Cynthia A. Toth, Joseph A. Izatt, Sina Farsiu. Fast Acquisition and Reconstruction of Optical Coherence Tomography Images via Sparse Representation // IEEE Trans. on Medical Imaging. 2013. V. 32. № 11. P. 2034−2049.
- Hamed Kajbaf, Joseph T. Case, Zengli Yang, Yahong Rosa Zheng. Compressed Sensing for SAR-Based Wideband Three-Dimensional Microwave Imaging System Using Non-Uniform Fast Fourier Transform // ET Radar, Sonar & Navigation. July 2013. V. 7. № 6. P. 658−670.
- Jungang Yang, John Thompson, Xiaotao Huang, Tian Jin, Zhimin Zhou. Random-Frequency SAR Imaging Based on Compressed Sensing // IEEE Transactions on Geoscience and Remote Sensing. February 2013. V. 51. № 2. P. 983−994.
- Hossam M. Kasem, Maha El-Sabrouty. A Comparative Study of Audio Compression Based on Compressed Sensing and Sparse Fast Fourier Transform (SFFT): Performance and Challenges. [EHlektronnyjj resurs]: URL: http://arxiv.org/abs/1403.3061 (data obrashhenija: 31.03.2016).
- Niranjan M Gowda, Arun Pachai Kannu. Compressive sensing framework for signal processing in heterogeneous cellular networks // IEEE Global Communications Conference. 3−7 Dec. 2012. P. 3610−3615 (doi: 10.1109/GLOCOM.2012.6503676).
- Christy Fernandez Cull, David A. Wikner, Joseph N. Mait, Michael Mattheiss, David J. Brady. Millimeter-wave compressive holography // Applied Optics. July 2010. 49(19): E67−E82.
- Scott A. Wilson Ram M. Narayanan. Compressive wideband microwave radar holography // Proc. SPIE 9077, Radar Sensor Technology XVIII, 907707. May 29 2014. doi:10.1117/12.2050131.
- H.L. Taylor, S.C. Banks, J.F. McCoy. Deconvolution with the l1 norm // Geophysics. 1979.
- S. Levy and P.K. Fullagar. Reconstruction of a sparse spike train from a portion of its spectrum and application to high-resolution deconvolution // Geophysics. 1983. V. 46. P. 1235−1243.
- Emmanuel Candes, Justin Romberg, Terence Tao. Robust uncertainty principles: Exact signal reconstruction from highly incomplete frequency information // IEEE Trans. on Information Theory. 2006. V. 52. № 2. P. 489−509.
- Mackenzie Dana. Compressed sensing makes every pixel count // What\'s Happening in the Math. Sciences. 2009. AMS. 114−127.
- Andrey V. Zhuravlev, Vladimir V. Razevig, Sergey I. Ivashov, Alexander S. Bugaev. Experimental Comparison of Multi-Static and Mono-Static Antenna Arrays for Subsurface Radar Imaging // Proceedings of the IEEE International Conference on Microwaves, Communications, Antennas and Electronic Systems. Tel-Aviv, Israel. 2−4 November 2015. P. 1−4 (doi: 10.1109/COMCAS.2015. 7360380).
- Andrey Zhuravlev, Alexander Bugaev, Sergey Ivashov, Vladimir Razevig, Igor Vasiliev. Microwave holography in detection of hidden objects under the surface and beneath clothes // XXX International Union of Radio Science (URSI) General Assembly. Istanbul. Turkey. August 13−20. 2011.
- Otkrytaja biblioteka SciPy dlja jazyka programmirovanija Python [EHlektronnyjj resurs]: ofic. sajjt. URL: http://www.scipy.org/ (data obrashhenija: 31.03.2016).
- Dianyuan Han. Comparison of Commonly Used Image Interpolation Methods // Proceedings of the 2nd International Conference on Computer Science and Electronics Engineering (ICCSEE 2013). Published by Atlantis Press. Paris. France. P. 1556−1559.