350 rub
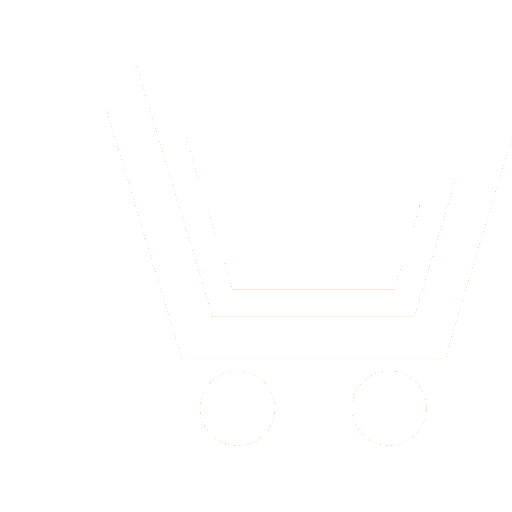
Journal Radioengineering №3 for 2016 г.
Article in number:
Performance analysis of blind source separation algorithms
Authors:
N.E. Miroshnikova - Post-graduate Student, Moscow Technical University of Communication and Informatic. E-mail: drugbamir@mail.ru
Abstract:
The aim of the article is to analyze the performance of blind source separation algorithms, which allow us to select desired signal from signal mixture received by the antenna in the presence of spatial diversity. The first section provides a statement of the blind source separation (BSS) problem. Most of the classical blind separation methods based on a priori information. Under the assumption that signals in a mixture viewed statistically independent, algorithms based on the method of independent component analysis (ICA) were analyzed in the article.
The second section, performance parameter of algorithms is given. Signal / interference ratio (SIR) at the output of the separation unit were used to compare separation efficiency of algorithms.
In the third section, simulation parameters are defined. The number of antennas P was taken as 3. A mixture of two signals with dif-ferent types of linear modulation received by the antenna array, than, the number of signals in the mixture N = 2. Signals with the following types of modulation were used: BPSK, QPSK, 8 PSK, DPSK, QAM 16.When modeling is considered passing the mixture receiving radio path signals. Model of super heterodyne receiver was used, designed in Matlab. The block dividing the digitized signal is fed from the output of the intermediate frequency. The following blind separation algorithms belonging to the group based on the ICA were used: AMUSE (Algorithm for Multiple Unknown Signals Extraction) [1], SOBI (Second Order Blind Identification) [2], JADE (Joint Approximation Diagonalisation of Eigen matrices) [3], EFICA (EfficientFastICA) [4]. Separaion of the signals mixture using the above algorithms and calculation of SIR parameter was made in the MATLAB ICALAB application.
. If you are adding to a mixture of white Gaussian noise SOBI algorithm can be effective, especially at small ratios Eb / No. For example, the separation of a mixture of signals from QPSK and DPSK modulation with respect to Eb / No = 5 dB SIR value using the SOBI algorithm is 1 dB higher than when using the algorithm EFICA. The result of the algorithm is also dependent on the length of the sample. When its value increases SIR at the output of division increases. Also worth noting is that the efficiency of algorithms is not strongly dependent on the type of signal modulation in the mixture.
SIR value calculation results show that the most effective of the selected algorithms are blind separation algorithms SOBI and EFICA, while algorithms based on higher order statistics (JADE) are ineffective. SIR maximum value were obtained using the EFICA algorithm for separating a mixture of signals with QPSK and BPSK modulation. SIR minimum value obtained using the JADE algorithm. Separation performance of the algorithm is also dependent on the sample length. When its value increases, than SIR at the output of separation unit increases too. Also worth noting is that the efficiency of algorithms is not strongly dependent on the modulation type of signals in the mixture.
Pages: 37-42
References
- Miroshnikova N.E. Obzor sistem kognitivnogo radio // T‑comm: telekommunikacii i transport. 2013. № 9. S. 108−112.
- Miroshnikova N.E. Vlijanie oshibok sinkhronizacii na priem cifrovykh signalov // T‑comm: telekommunikacii i transport. 2013. № 9.S. 112−115.
- Priputin V.S. Metod slepogo razdelenija signalov na baze statistik vtorogo porjadka v zadache prostranstvenno-poljarizacionnojj selekcii // T‑comm: telekommunikacii i transport. 2014. № 6. S. 36−39.
- Priputin B.C. Koncepcija primenenija tenzornykh metodov slepogo razdelenija signalov v adaptivnykh antennykh reshetkakh // Materialy Mezhdunar. nauchno-tekhnich. seminara «Sistemy sinkhronizacii, formirovanija i obrabotki signalov v info-kommunikacijakh». JAroslavl. 2013. S. 205−208.
- Comon P., Jutten C. Handbook of Blind Source Separation: Independent Component Analysis and Applications.: ELSEVIER. 2010. 831 p.
- Belouchrani A., Abed-Meraim K., Cardoso J.F.Second-order blind separation of temporally correlated sources // Proc. Int. Conf. onDigitalSig. Proc. 1993. P. 346−351.
- Tong L., Soon V., Huang Y.F., Liu R. Indeterminacy and identifiability of blind identification // IEEE Trans. CAS. 1991. V. 38. P. 499−509.
- Cardoso J.-F., Souloumiac A. Blind beam-forming for non Gaussian signals // IEEE Proceedings. 1993. V. 140. P. 362−370.
- Koldovsky Z.Efficient variant of algorithm FastICA for Independent Component Analysis attaining the Cramer-Rao Lower Bound // IEEE trans. on neural networks. 2006. V. 17. P. 1265−1277.
- Cichocki A, Amari S, Siwek K, Tanaka T, Anh Huy Phan, Zdunek R. ICALAB.MATLAB Toolbox Ver. 3 for signal processing // ICALAB toolboxes. 2003. URL. http://www.bsp.brain.riken.jp/ICALAB/ICALABSignalProc/ (data obrashhenija 17.03.2015).
- Chevalier P., Ferréol A., Albera L. High resolution direction finding from higher order statistics: The 2q-MUSIC algorithm // IEEE Trans. SignalProcess. 2006. V. 54. № 8. P. 2986−2997.