350 rub
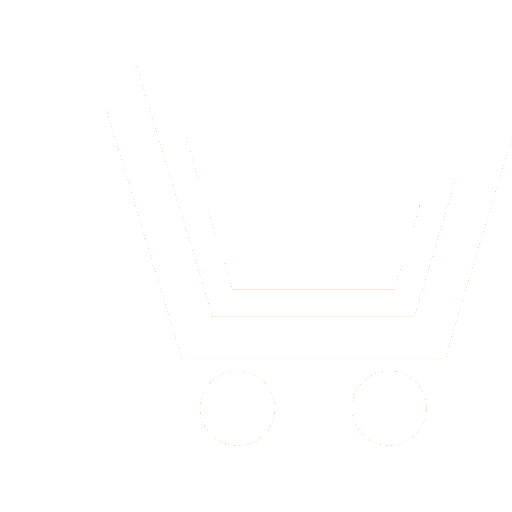
Journal Radioengineering №10 for 2016 г.
Article in number:
Model of nonlinear blurring image
Authors:
I.V. Zhigulina - Ph. D. (Eng.), Associate Professor, Professor, Department of Mathematics, MESC «Zhukovsky-Gagarin Air Force Academy» (Voronezh)
E-mail: ira_zhigulina@mail.ru
Abstract:
This paper considers the model of nonlinear blurring. It is shown that the development of an effective deconvolution method is im-possible without an understanding of the video signal distortion mechanism. The light energy distribution over a discrete spatial coor-dinates at the formation of a video sequence frame is analyzed. The video signal distortion process is described by the discrete-analog representation of luminosity.
The general case of a non-linear movement law is considered. Double integrals of the Heaviside function are used to find deposits of discrete-analog luminosity elements in the formation of distorted samples dynamic object. The system of linear algebraic equations for the undistorted samples of output object image video signal is obtained. The system describes the linear blur in the particular case. The law of the object image movement on the photosensitive surface is in the form of the Maclaurin expansion. Cases of uniform and uniformly accelerated motion are analyzed.
Oscillating blur considered. In the simplest case, it is modeled by two successive linear blurring directed in opposite directions. Systems of linear equations for the undistorted video signal samples of a dynamic object image are obtained. It is shown that the own methods of measurement the video signal samples corresponding to a stationary background for each specific type of oscillatory blurring are re-quired.
Pages: 116-122
References
- Gonsales R., Vuds R. Cifrovaja obrabotka izobrazhenijj. M.: Tekhnosfera. 2006.
- Gruzman I.S., Kirichuk V.S., Kosykh V.P., Peretjagin G.I., Spektor A.A. Cifrovaja obrabotka izobrazhenijj v informacionnykh sistemakh.: Uchebnoe posobie. Novosibirsk: Izd-vo NGTU. 2000.
- Donatelli M., Estatico C., Martinelli A., Serra- Capizzano S. Improved image deblurring with antireflective boundary conditions and reblurring // Inverse problems. 2006. V. 22. P. 2035−2053.
- Holmes T.J. Blind deconvolution: Handbook of biological confocal microscopy. Third edition, edited by J.B. Pawler, Springer Science+Business Media, LLC, New York. 2006.
- Jiann-Ming Wu, Hsiao-Chang Chen, Chun-Chang Wu, Pei-Hsun Hsu. Blind image deconvolution by neural recursive function approximation // World Academy of Science, Engineering and Technology. 2010. V. 4. P. 10−22.
- Sizikov V.S., Belov I.A. Rekonstrukcija smazannykh i defokusirovannykh izobrazhenijj metodom reguljarizacii // Opticheskijj zhurnal. 2000. T. 67. № 3. S. 60−63.
- Machikhin A.S. Avtomaticheskoe vosstanovlenie izobrazhenijj, iskazhennykh prjamolinejjnym ravnomernym smazom // Priborostroenie. 2008. T. 51. № 1. S. 59−64.
- Voskobojjnikov JU.E., Mukhina I.N. Lokalnyjj reguljarizirujushhijj algoritm vosstanovlenija kontrastnykh signalov i izobrazhenijj // Avtometrija.2000. № 3.S. 45−53.
- Bogoslovskijj A.V., Bogoslovskijj E.A., ZHigulina I.V., Vasilev V.V., Ponomarev A.V. Linejjnyjj smaz // Radiotekhnika. 2015. № 12. S. 147−153.
- Bogoslovskijj A.V., Bogoslovskijj E.A., ZHigulina I.V., Vasilev V.V., Ponomarev A.V. Algoritm kompensacii linejjnogo smaza izobrazhenija dvizhushhegosja obekta // Materialy XVIII Mezhdunar. nauch. konf. «Cifrovaja obrabotka signalov i ee primenenie (DSPA-2016)». M.: RNTOREHS im. A.S. Popova. 2016. S. 817−822.