350 rub
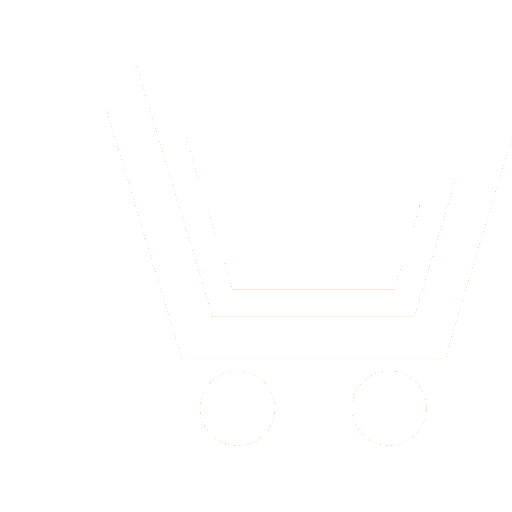
Journal Radioengineering №6 for 2015 г.
Article in number:
Algorithm based on clustering applications for searching and removing time series anomalies
Authors:
T.V. Afanasieva - Dr. Sc. (Eng.), Professor, Department «Information Systems», Ulyanovsk State Technical University. E-mail: tv.afanasjeva@gmail.com
D.V. Zavarzin - Post-graduate Student, Department «Information Systems», Ulyanovsk State Technical University. E-mail: ivan.sibirev@yandex.ru
I.V. Sibirev - Post-graduate Student, Department «Information Systems», Ulyanovsk State Technical University. E-mail: dzavarzin91@gmail.com
Abstract:
Under the anomalies we understand atypical patterns in the behavior of the studding process.
At the heart of anomaly detection among the TS may lie compare the normal behavior (model) and the observed process, the allocation of noise anomalies from the normal process data. Currently, to search for anomalies widely used methods of cluster analysis, sliding window, statistical methods (eg, correlation analysis, testing hypotheses about the similarity of samples, hidden Markov models, and others).
In this paper we consider the new solution of the problem of detection and removal of anomalies in TS. An algorithm of search and detect anomalies using centroid clustering method, as well as the removal of anomalies found. The practical application of this algorithm - correction values of TS, in order to improve the accuracy of its forecast.
Pages: 59-62
References
- Deepthi Cheboli. Anomaly Detection of Time Series // Facility Of The Graduate School Of The University Of Minnesota. EHlektronnyjj resurs. 2010. 75 c. URL: http://conservancy.umn.edu/bitstream/11299/92985/1/Cheboli_Deepthi_May2010.pdf (data obrashhenija: 24.02.2015).
- JArushkina N.G., Afanaseva T.V., Perfileva I.G. Intellektualnyjj analiz vremennykh rjadov. Ucheb. posobie. M. 2012. Ser. Vysshee obrazovanie.
- Makkafri D. Obnaruzhenie anomalnykh dannykh metodom k‑srednikh // ZHurnal MSDNMagazine. 2013. № 2. URL: http://msdn.microsoft.com/ru-ru/magazine/jj891054.aspx (data obrashhenija: 18.02.2015).
- Munz G., Traffic Anomaly Detection Using K‑Means Clustering / Computer Networks and Internet. GI/ITG-Workshop MMBnet. 2007. URL: http://www.net.in.tum.de/fileadmin/TUM/members/muenz/documents/muenz07k-means.pdf (dataobrashhenija: 11.03.2015).
- Barsegjan A.A. Metody i modeli analiza dannykh: OLAP i DataMining. SPb.:BKHV-Peterburg. 2004. S. 158−163.
- Afanaseva T.V., Zavarzin D.V. Algoritm poiska anomalijj v processakh na osnove nechetkikh tendencijj vremennykh rjadov // Trudy XIV nacionalnojj konf. po iskusstvennomu intellektu s mezhdunar. uchastiem KII-2014. 24−27 sentjabrja 2014 g. g. Kazan. Rossija. Kazan: Izd‑vo RIC «SHkola». 2014. T. 3. S. 5−12.
- Begum N., Keogh E. Rare Pattern Discovery from Time Series // Int\'l Conference on Very Large Databases (VLDB). 2015. URL: http://www.vldb.org/pvldb/vol8/p149-begum.pdf (data obrashhenija: 16.03.2015).