350 rub
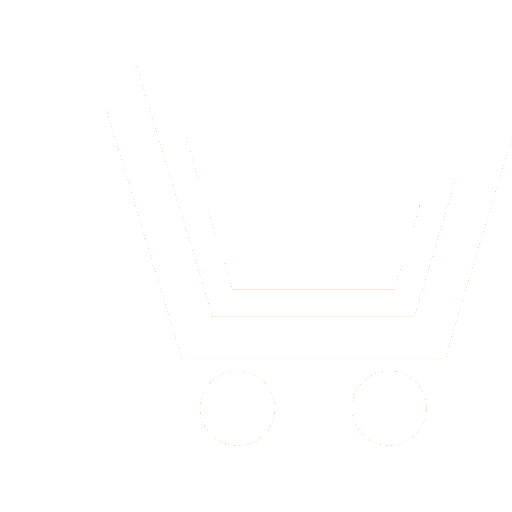
Journal Radioengineering №6 for 2015 г.
Article in number:
Time series forecasting collectives of methods
Authors:
M.V. Artyukhov - General Director of LLC «Everest Research». E-mail: martukhov@krips-consulting.ru
A.A. Romanov - Ph. D. (Eng.), Department «Information Systems», Ulyanovsk State Technical University. E-mail: romanov73@gmail.com
G.Y. Guskov - Post-graduate Student, Department «Information Systems», Ulyanovsk State Technical University. E-mail: guskovgleb@gmail.com
I.A. Timina - Assistant Department «Information Systems», Ulyanovsk State Technical University. E-mail: i.timina@ulstu.ru
Abstract:
In this paper we develop the collective of several methods of time series modeling - exponential smoothing models. We make an as-sumption that the creation of complicated methods modeling and forecasting of time series is optimal if we know all features of subject area and we able to include them in model. To improve the quality of forecasting unknown kind time series, we propose to use the aggregate results of several models. Using a fuzzy approach allows to create models with more options.
Pages: 48-54
References
- Song Q., Chissom B. Forecasting enrollments with fuzzy time series // Fuzzy Sets and Systems. Part I. 1993. P. 1−9.
- Peng G., Wang J., Ren P., Gao H., Luo Y. A new improved forecasting method integrated fuzzy time series with exponential smoothing method // Int. J. Environment and Pollution. 2013. V. 51. № ¾. P. 206−221.
- Elliott G., Granger C.W.J., Timmermann A. Forecast combinations // Handbook of economic forecasting. ElsevierPub. 2006. P. 135−196.
- Vovk V.G. Universal Forecasting Algorithms // Journal of Information and Computation. 1992. V. 96. P. 245−277.
- Huarng K. Effective lengths of intervals to improve forecasting in fuzzy time series // Fuzzy Sets and Systems. 2001. № 123. P. 387−394.
- Viertl R. Statistical Methods for Fuzzy Data // John Wiley & Sons Ltd. 2011. 270 p.
- Perfilieva I., Yarushkina N., Afanasieva T., Romanov A. Time series analysis using soft computing methods// International Journal of General Systems. 08/2013. V. 42. № 6.
- JArushkina N.G., Afanaseva T.V. Nechetkie vremennye rjady kak instrument dlja ocenki i izmerenija dinamiki processov // Datchiki i sistemy. 2007. T. 12. S. 46−50.
- JArushkina N.G., Perfileva I.G., Afanaseva T.V. Integralnyjj metod nechetkogo modelirovanija i analiza nechetkikh tendencijj // Avtomatizacija processov upravlenija. 2010. № 2(20). S. 59−63.
- Perfilieva I., Novák V., Romanov A., Yarushkina N. Time series grouping and trend forecast using f1-transform and fuzzy natural logic // XI International FLINS Conference (FLINS-2014). DOI: 10.1142/9789814619998_0026.
- Stock J., Watson M. Combination forecasts of output growth in a seven-country data set // Journal of Forecasting. 2004. V.23. P. 405−430.
- [EHlektronnyjj resurs] http://irafm.osu.cz/cif/cif-dataset.txt.
- [EHlektronnyjj resurs] http://www.neural-forecasting-competition.com/NN3/.
- Perfileva I.G., JArushkina N.G., Romanov A.A. Modelirovanie nestacionarnykh vremennykh rjadov // IIMezhdunar. Pospelovskijj simpozium «Gibridnye i sinergeticheskie intellektualnye sistemy». GISIS-2014. S. 281−289.
- Namestnikov A.M., Yarushkina N.N. Efficiency of Genetic algorithms for automated design problems // IzvestijaRossijjskojjakademiinauk. Teorija i sistemy upravlenija. 2002. № 2. S. 127−133.
- JArushkina N.G., Perfileva I.G., Afanaseva T.V. Integracija nechetkikh modelejj dlja analiza vremennykh rjadov // Izvestija Samarskogo nauchnogo centra Rossijjskojj akademii nauk. 2010. T. 12. № 4−2.S. 506−509.
- Yarushkina N.G. Soft Computing and complex system analysis // International Journal of General Systems. 2001. T. 30. № 1. S. 71−88.
- JArushkina N.G., Afanaseva T.V., Perfileva I.G. Intellektualnyjj analiz vremennykh rjadov. Ucheb. posobie. M.: 2012. 159 s.