350 rub
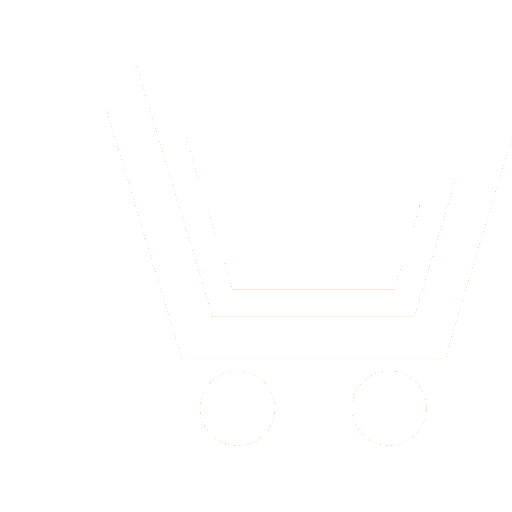
Journal Radioengineering №10 for 2015 г.
Article in number:
Method of determining 3D permittivity profile of a sample using artificial neural network mathematical model
Keywords:
artificial neural network
FDTD method
training algorithm for artificial neural network
nondestructive evaluation and testing
Authors:
A.V. Brovko - Ph. D. (Phys.-Math.), Associate Professor, Department «Applied Information Technologies», Yuri Gagarin State Technical University of Saratov. E-mail: brovkoav@gmail.com
Abstract:
The problem of determining the spatial distribution (profile) of the complex permittivity of the dielectric material within the sample is considered in the paper. This problem is relevant in many practical applications. Particular interest in the technology arises from the considerable principal obstacle in the development of controllable microwave sintering of particulate and powder materials.
To solve this problem, the paper suggests a method based on the use of artificial neural network as a numerical inverter, electromagnetic measurements multiport waveguide system (turnstile connection waveguides), and three-dimensional approximation of the profile of the dielectric constant with the quadratic polynomial function.
The initial data for determining the profile of the dielectric constant are the elements of the scattering matrix of turnstile waveguide junction within which the sample is placed. Since the measuring system provides six ports (including two orthogonally oriented modes in a circular waveguide), the total scattering matrix contains 36 complex coefficients. These coefficients are the input data for the artificial neural network which is used as an inverter for the numerical solution of the inverse problem.
An arbitrary three-dimensional profile of the dielectric constant can be approximated with quadratic polynomial function of the three spatial coordinates. To construct such a function, optimal experimental design theory is used (we apply central composite orthogonal design). This function can be completely defined by specifying its values in 15 base points. These values in the base points are the output of the artificial neural network.
Neural network training is performed using the results of direct numerical simulation of the measuring system with the test sample using the FDTD method. Training sets (pairs of input and corresponding output data sets) are generated as follows. The distribution of dielectric constant using a random set of values in 15 base points is defined in the sample. Next, the simulation of the measuring system with the distribution of the dielectric constant inside the sample is performed, and the scattering matrix of turnstile junction is calculated. Thus it turns out a single training set. For the next training set the following random distribution of the dielectric constant is generated, and the scattering matrix is calculated, etc.
The quality of training of the neural network is estimated using the absolute and relative error of profiles reconstruction for profiles which did not participate in the training of the network. After the neural network is sufficiently trained, it can be used to determine the profiles of the dielectric constant of samples from measurements of the coefficients of the scattering matrix of the measuring system.
To illustrate the performance of the proposed method, the paper presents numerical results of the reconstruction of three test profiles defined with nonpolynomial functions. For all three profiles the relative error in determining the profile of the dielectric constant does not exceed 10%.
Pages: 115-120
References
- Li X., Davis S.K., Hagness S.C., Van DerWeide D.W., VanVeen B.D. Microwave imaging via space‑time beamforming: experimental investigation of tumor detection in multilayer breast phantoms // IEEE Transactions on Microwave Theory and Techniques. 2004. V. 52. № 8. P. 1856−1865.
- Belkebir K., Kleinman R.E.,Pichot C. Microwave imaging: Location and shape reconstruction from multifrequency scattering data // IEEE Transactions on Microwave Theory and Techniques. 1997. V. 45. № 4. P. 469−476.
- Qaddoumi N., Carriveau G., Ganchev S.,Zoughi R. Microwave imaging of thick composite panels with defects // Materials Evaluation. 1995. V. 53. № 8. P. 926−929.
- Bykov Y.V., Rybakov K.I., Semenov V.E. High‑temperature microwave processing of materials // Journal of Physics D: Applied Physics. 2001. V. 34. № 13. P. R55−R75.
- Brovko A.V., Murphy E.K., Yakovlev V.V. Waveguide microwave imaging: neural network reconstruction of functional 2D permittivity profiles // IEEE Transactions on Microwave Theory and Techniques. 2009. V. 57. № 2. P. 406−414.
- Pleskunin V.I., Voronina E.D. Teoreticheskie osnovy organizacii i analiza vyborochnykh dannykh v ehksperimente. L.: Leningradskijj un-t. 1979.
- QuickWave‑3 DTM, QWED Sp. z o.o., ul. Nowowiejska 28, lok. 32, 02‑010 Warsaw, Poland. http://www.qwed.com.pl/.
- Dolinina O.N., Ermakov A.V. Metod povyshenija ehffektivnosti obrabotki videoinformacii s ispolzovaniem GRID‑vychislenijj // Vestnik SGTU. 2010. № 4(50). Vyp. 2. S. 131−133.
- Kleev A.I., Manenkov A.B., Rozhnev A.G. CHislennye metody rascheta diehlektricheskikh volnovodov (volokonnykh svetovodov). Universalnye metodiki // Radiotekhnika i ehlektronika. 1993. T. 38. № 11. S. 1938.
- Kolomejjcev V.A., Komarov V.V., KHomjakov S.V. Modelirovanie nereguljarnykh volnovedushhikh struktur slozhnojj konfiguracii s neodnorodnym pogloshhajushhim zapolneniem // Radiotekhnika i ehlektronika. 2000. T. 45. № 12. S. 1420.
- Petrov E.Yu., Kudrin A.V. Exact axisymmetric solutions of the maxwell equations in a nonlinear nondispersive medium // Physical Review Letters. 2010. T. 104. № 19. S. 190404.