350 rub
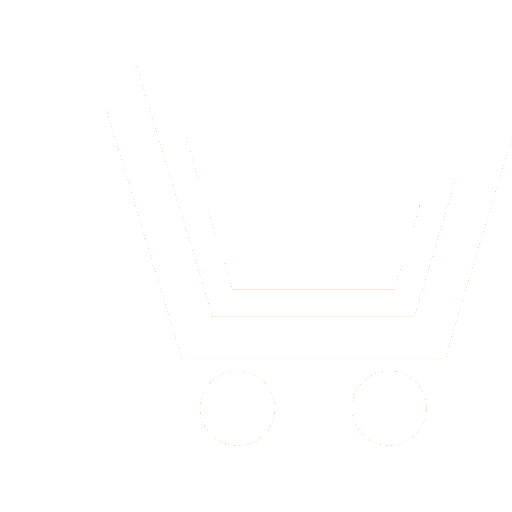
Journal Radioengineering №7 for 2014 г.
Article in number:
On the use of mutual information as objective function for image parameters - estimation
Keywords:
digital image
objective function
mutual information
image entropy
cross-validation
optimization
stochastic gradient
Authors:
S.V. Voronov - Post-graduate Student of Ulyanovsk State Technical University. E-mail: valmedia@yandex.ru
Abstract:
Recently, when specifying the objective function for digital image parameters - estimation information-theoretic approach is becoming common. When using stochastic gradient estimation procedure the most interesting information-theoretic objective function is mutual information (MI). A key step in evaluating the MI gradient estimation is to estimate the probability density function (PDF) using image sample. Based on the approach to solve this problem the MI gradient estimation approaches can be di-vided into two groups. The first group called histogram-based methods. Here entropy and the joint entropy of the images are estimated using the histograms of image samples. In the second group methods are based on the PDF reconstruction using image samples. Methods of the second group, in particular Parzen window density estimation, provide better PDF estimation in comparison with the histogram-based methods. However, their disadvantages are low accuracy of MI gradient estimation and requirement of big image sample. The last one is undesirable in real-time image processing. A method of estimating the entropy of images based on the cross-validation procedure and aimed at reducing the computational cost is proposed. Studies have shown that the application of the proposed method al-lows to reduce the sample size twice in comparison with the state-of-the-art algorithm EMMA. In this case the computational cost of image entropy estimation reduces by 10-12 % with a slight increase in the error variance (a few percent). When using the Parzen window method the pixel intensity gradients with respect to the spatial coordinates are estimated via the finite differences. Due to image correlation the quality of the estimates and the MI gradient estimates depends on the changes in parameters used for finite difference estimation. The possibilities for error minimization finding image intensities PDF by optimizing the values of these increments are analyzed. It is shown that the optimal values deter-mined by the form of the image correlation function and signal-to-noise ratio. Results of statistical modeling have shown adequacy of the analytical expressions. Using the optimization provides almost double the gain in performance of the stochastic gradient image inter-frame geometric deformation estimation.
Pages: 88-94
References
- Goshtasby A.A. Image registration. Principles, tools and methods // Advances in Computer Vision and Pattern Recognition. Springer. 2012. P. 441.
- D'Agostino E, Maes F, Vandermeulen D, Suetens P. An information theoretic approach for non-rigid image registration using voxel class probabilities // Med Image Anal. 2006. V 6(3). P. 413-431.
- Tashlinskij A.G., Voronov S.V. Analiz czelevy'x funkczij pri rekurrentnom oczenivanii mezhkadrovy'x geometricheskix deformaczij izobrazhenij // Naukoemkie texnologii. 2013. T. 14. № 5. S. 16-21.
- Tashlinskij A.G. Psevdogradientnoe oczenivanie prostranstvenny'x deformaczij posledovatel'nosti izobrazhenij // Naukoemkie texnologii. 2002. T. 3. S. 32-43.
- Cifrovaya obrabotka izobrazhenij v informaczionny'x sistemax: Uchebnik NGTU: uchebnoe posobie / Gruzman I.S., Kirichuk V.S., Kosy'x V.P., Peretyagin G.I., Spektor A.A. Novosibirsk: Izd-vo NGTU. 2002. 352 c.
- Pluim J., Maintz A., Viergever M. Interpolation Artefacts in Mutual Information-Based Image Registration // Computer Vision and Image Understanding. 2000. № 77. P. 211-232.
- Collignon A. Multi-modality medical image registration by maximization of mutual information. PhD thesis. Catholic University of Leuven. Leuven. Belgium. 1998.
- Spall J. Introduction to Stochastic Search and Optimization: Estimation, Simulation, and Control. Wiley, Hoboken. NJ. 2003. P. 618.
- Spall J. Implementation of the simultaneous perturbation method for stochastic optimization // IEEE Transactions on Aerospace and Electronic Systems. 1998. № 34. P. 817-823.
- Klein S., Staring M., Pluim P. Evaluation of Optimization Methods for Nonrigid Medical Image Registration Using Mutual Information and B-Splines // IEEE Transactions on Image Processing. 2007. V. 16. № 12. P. 2879-2890.
- Klein S., Staring M., Pluim P. Comparison of gradient approximation techniques for optimization of mutual information in nonrigid registration // Proc. SPIE 5747, Medical Imaging: Image Processing. 2005. P. 192-203.
- Tashlinskij A.G., Voronov S.V., Voronov I.V. Analiz czelevy'x funkczij v zadache oczenivaniya vzaimny'x geometricheskix deformaczij izobrazhenij // Avtomatizacziya proczessov upravleniya. 2013. № 4(34). S. 26-29.
- Parzen E. On Estimation of a Probability Density Function and Mode // Annals of Math. Statistics. 1962. V. 33. P. 1065-1076.
- Viola P., Wells III W.M. Alignment by maximization of mutual in-formation // International Journal of Computer Vision. 1997. V. 24. P. 137-154.
- Voronczov K.V. Kombinatorny'j podxod k oczenke kachestva obuchaemy'x algoritmov // Matematicheskie voprosy' kibernetiki. M.: Fizmatlit. 2004. T. 13. S. 5-36.
- Tashlinskij A.G., Xoreva A.M., Smirnov P.V. Vy'bor konechny'x raznostej pri naxozhdenii psevdogradienta czelevoj funkczii v proczedurax oczenivaniya mezhkadrovy'x deformaczij izobrazhenij // Radiotexnika. 2012. № 9. S. 56-60.
- Tashlinskii A.G., Dikarina G.V., Minkina G.L., Repin A.N. Pseudogradient optimization in the estimation of geometric interframe image deformations // Pattern Recognition and Image Analysis, 2008. V. 18. № 4. P. 706-711.
- Tashlinskii A.G., Safina G.L., Voronov S.V. Pseudogradient optimization of objective function in estimation of geometric interframe image deformations // Pattern recognition and image analysis. 2012. V. 22. №. 2. P. 386-392.