350 rub
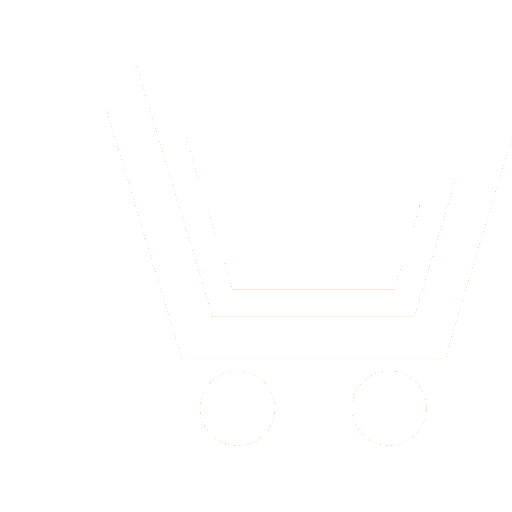
Journal Radioengineering №5 for 2013 г.
Article in number:
Color image quality evaluation for impulse noise suppressing
Authors:
A.L. Priorov, V.V. Khryashchev, E.A. Pavlov, N.B. Gerasimov, A.M. Shemyakov
Abstract:
In many practical applications images are corrupted by noise caused either by faulty image sensors or due to transmissions corruption resulting from artificial or natural phenomena. Transmission noise, also known as salt-and-pepper noise in grey-scale imaging, is modeled by an impulsive distribution. However, a problem in the study of the effect of the noise in the image processing community is the lack of commonly accepted multivariate impulse noise model. A number of simplified models have been introduced recently, to assist the performance evaluation of the different color image filters.
Based on trichromatic color theory, color pixels are encoded as three scalar values, namely, red, green and blue (RGB color space). Since each individual channel of a color image can be considered as a monochrome image, traditional nonlinear image filtering techniques have involved the application of scalar filters on each channel separately. However, this disrupts the correlation that exists between the color components of natural images. As such the color noise model should be considered as a 3-channel perturbation vector in color space.
The main approach for impulse noise removing is to use median-based filters. However, these nonlinear filters also tend to modify pixels that are not affected by the noise. In addition, when impulse noise probability is high, they are prone to edge jitter, so that details and edges of the original image are usually blurred by the filter.
In order to improve performance of median-based filter approach, various decision-based filters have been proposed, where possible impulse noise pixels are first identified and then replaced by using median filter. The examples of decision-based filters for random-valued impulse noise removal from grayscale images are: adaptive center-weighted median filter and directional weighted median filter. These filters are good in locating the noise even in the case of high noise probability.
In this paper authors introduce a novel filter for the purpose of random-valued impulse noise removal from RGB-color images which utilizes the advantages of both weighted vector median and decision-making filtration schemes.
Pages: 41-49
References
- Pratt W. Digital Image Processing // Third Edition. Wiley. 2001.
- Jahne B. Practical Handbook on Image Processing for Scientific and Technical Applications // Second edition. CRC. 2004.
- Lukac R. Computational Photography: Methods and Applications. CRC Press / Taylor & Francis. 2010.
- Gonsales R., Vuds R. Cifrovaja obrabotka izobrazhenijj. M.: Tekhnosfera. 2005.
- Plataniotis K.N., Androutsos D., Venetsanopoulos A.N. Adaptive Fuzzy Systems for Multichannel Signal Processing // Proceedings of the IEEE. 1999. V. 87. №9. P. 1601-1622.
- Plataniotis K.N., Androutsos D., Venetsanopoulos A.N. Colour Image Filter: The Vector Directional Approach // Optical Engineering Journal. 1997. V. 36(9). P. 2375‑2383.
- Smolka B. Peer Group Filter for Impulsive Noise Removal in Color Images // Lecture Notes in Computer Science. Springer Berlin. 2008. P. 699-707.
- Russ J. The image processing handbook // CRC. 1995.
- Abreu E., Lightstone M., Mitra S., Arakawa K. A New Efficient Approach for the Removal of Impulse Noise from Highly Corrupted Images // IEEE Trans. on Image Processing. 1996. V. 5. №6. P. 1012-1025.
- Wang Z., Zhang D. Progressive switching median filter for the removal of impulse noise from highly corrupted images // IEEE Trans. Circuits Systems ‑ II. 1999. V. 46. №1. P. 78-80.
- Mitra S., Sicuranza G. Nonlinear Image Processing. Academic Press. 2000.
- Plataniotis K.N., Androutsos D., Venetsanopoulos A.N. Multichannel filters for image processing // Signal Processing: Image Communications. 1997. V. 9. №2. P. 143-158.
- Plataniotis K.N., Venetsanopoulos A.N. Color Image Processing and Applications. Springer, Berlin. 2000.
- Lukac R., Smolka B., Martin K., Plataniotis K.N., Venetsanopoulos A.N. Vector Filtering for Color Imaging // IEEE Signal Processing Magazine. 2005. V. 22(1). P. 74‑86.
- Barni M., Cappellini V., Mecocci A. Fast Vector Median Filter Based on Euclidean Norm Approximation // IEEE Signal Processing Letters. 1994. V. 1(6). P. 92-94.
- Bukhtojarov S.S., Priorov A.L., Apalkov I.V., KHrjashhev V.V. Perekljuchajushhijjsja mediannyjj filtr s blokom predvaritelnogo detektirovanija // Cifrovaja obrabotka signalov. 2006. №4. S. 2-8.
- Signal and Image Processing Image Database (http://sipi.usc.edu/database).
- Priorov A.L., KHrjashhev V.V., Kujjkin D.K. Udalenie impulsnogo shuma iz izobrazhenijj na osnove ranzhirujushhikh filtrov // EHlektrosvjaz. 2010. №3. S. 31-34.
- Priorov A.L., KHrjashhev V.V., Golubev M.N. Udalenie impulsnogo shuma so sluchajjnymi znachenijami impulsov iz izobrazhenijj // Radiotekhnika. 2010. №5. S. 72-79.
- Khryashchev V.V., Kuykin D.K., Studenova A.A. Vector median filter with directional detector for color image denoising // Proc. World Congress on Engineering 2011. London. 2011. V. 2. P. 1699-1704.