350 rub
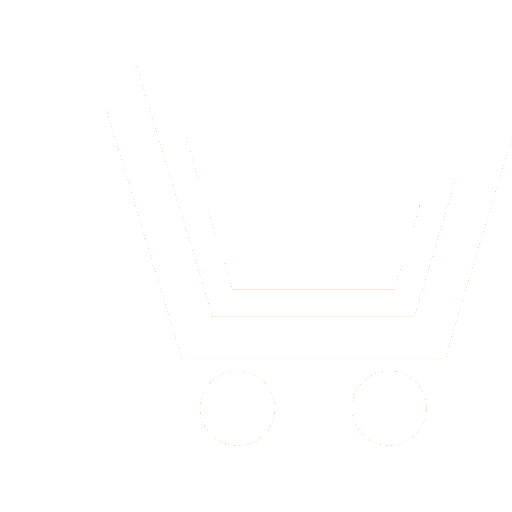
Journal Radioengineering №12 for 2013 г.
Article in number:
Mobile network formalized representation with dynamic subscriber`s clustering under virus influence
Keywords:
mobile communication
virus type information influence
dynamic clustering
epidemiological model
Authors:
А.G. Alferov - Ph.D. (Eng.), JSC - Concern "Sozvezdie"
I.O. Tolstykh - Ph.D. (Eng.), JSC - Concern "Sozvezdie"
I.O. Tolstykh - Ph.D. (Eng.), JSC - Concern "Sozvezdie"
Abstract:
The expression for probability density definition of the arbitrary subscriber`s calls in a clustered network taking into account virus influence and network element interaction dynamics are proposed based on four standard statistical models consideration of the problem-oriented networks. Formalized representations are selected as a standard model network representations on the basis of: weak relationships, Small world, the network growth and agent-oriented structure.
Information impact on mobile network elements known to the present can be classified into: program intentionally installed in the equipment or generated during the process of exploitation by brining program codes into processing operating area from other subscribers. Information impact, mobile phone viruses, in particular, taking into account impact effect type can be divided into
- Passive, that have a low reproduction rate and do not affect considerably on data exchange key features;
- Active, with high reproduction rate, other subscribers unassisted calls and intense realization of objective functions;
- Destructive, primary function of which is the telephone operational environment destruction or (system) control area (after the given number of reproductions).
The analysis based on the proposed formalization method and clustered network operation model representation in information conflict mode showed that the probability density distribution of subscriber`s calls is weakly varied under information impacts with the passive nature. However, under virus active behavior this distribution can be changed considerably with mobile phone destructive virus behavior. In general, it could degenerate and is independent of the network model type and operating equipment.
Pages: 4-9
References
- Castells M. Materials for an exploratory theory of network society // Brit. J. Soc. 2000. № 51. P. 5-24.
- Granovetter M., Swedberg R. (Eds.) The Sociological Approaches to Labor Market Analysis: A Social Structural View / The Sociology of Economic Life. Boulder: Westview Press. 1992. P. 244-245.
- Bartolom'yu D. Stoxasticheskie modeli soczial'ny'x proczessov. M.: FiS. 1985. 295 s.
- Albert R., Jeong H., Barabasi A. Attack and error tolerance of complex networks // Nature. 2008. V. 506. P. 378-382.
- Ebel H., Mielsch L., Bornholdt S. Scale-free topology of e-mail networks // Phys. Rev. E. 2002. V. 66.
- Jain A.K., Murty M.N., Flynn P.J. Data Clustering: A Review (http://www.csee.umbc.edu/Nicholasclustering,p264-jain.pdf). 2006.
- Davy'denko V.A., Romashkina G.F., Chukanov S.N. Modelirovanie soczial'ny'x setej // Vestnik Tyumenskogo gosudarstvennogo universiteta. 2005. № 1. S. 68-79.
- Watts D.J., Strogatz S.H. Collective dynamics of -small-world - networks // Nature. 1998. V. 393. P. 440-442.
- Polansky L., Rockmore D., Johnson K., Repetto D. A mathematical model for optimal tuning systems. Mathematical monthly // Nature. 2007. V. 24. P. 528-535.
- Newman M.E.J., Moore C., Watts D.J. Mean-field solution of the small-world network model. Report 1399. Santa Fe Institute. Santa Fe, NM 87501.
- Aiello W., Chung F., Lu L. Random evolution of massive graphs // Handbook of Massive Data Sets. Eds. by J. Abello, P.M. Pardalos, M.G.C. Resende. Dordrecht: Kluwer. 2002. P. 97-122.
- Andersson H., Epidemic models and social networks // Math. Scientist. 1999. V. 24. P. 128-147.
- Hethcote H.W., The mathematics of infectious diseases // SIAM Review. 2000. V. 42. P. 599-653.