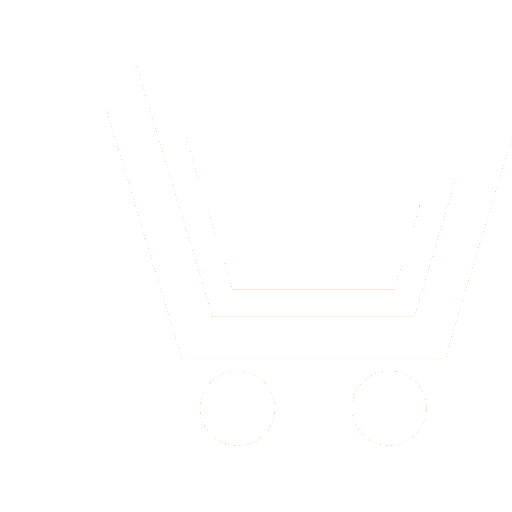
R.S. Ekhlakov1, V.A. Sudakov2
1 Financial University under the Government of the Russian Federation (Moscow, Russia)
2 Institute of Applied Mathematics n. a. M.V. Keldysh RAS (Moscow, Russia)
1 rsekhlakov@fa.ru, 2 vasudakov@fa.ru
The accuracy of traffic flow forecasting is influenced by many factors, such as road accidents and adverse weather conditions. The influence of weather conditions on such characteristics of traffic flow as the average speed, volume and density of cars on the road has been almost not studied. Unfavorable weather conditions, including snowfall, rain, fog, etc., have a negative impact on driver behavior. Thus, when forecasting traffic flows, adverse weather conditions should be taken into account as accurately as possible.
Target – analysis of the impact of adverse weather conditions on the average speed and congestion in the transport network. Improving the efficiency of load forecasting taking into account the influence of weather conditions.
Graphs of the relationships between average speed, density and number of cars on the route are presented. A deep learning model with an internal attention mechanism has been developed to increase performance when working with large data sets.
Research can serve as a starting point for further study of the problem with the goal of achieving high accuracy in forecasting congestion taking into account negative weather conditions.
Ekhlakov R.S., Sudakov V.A. Model of the influence of adverse weather conditions on the congestion of the transport network. Nonlinear World. 2024. V. 22. № 4. P. 122–128. DOI: https://doi.org/10.18127/ j20700970-202404-16 (In Russian)
- Pan Y.Y., Zhu Z.Y., Shen P.Y., Gu T.T., Zhang W.W. Highway traffic accident features and analysis of effect and adverse weather conditions in Zhejiang province. Highway. 2013. V. 58. № 12. P. 157–160.
- Liu Q.C., Liu T., Cai Y.F., Xiong X.X., Jiang H.B., Wang H., Hu Z.N. Explanatory prediction of traffic congestion propagation mode: A self-attention based approach. Physica A 573. 2021. DOI: http://dx.doi.org/10.1016/j.physa.2021.125940.
- Zhang W.B., Feng Y.Y., Lu K., Song Y.H., Wang Y.H. Speed prediction based on a traffic factor state network model. IEEE Trans. Intell. Transp. Syst. 2021. V. 22 (5). P. 3112-3122. DOI: http://dx.doi.org/10.1109/TITS.2020.2979924.
- Ekhlakov R., Andriyanov N. Multicriteria Assessment Method for Network Structure Congestion Based on Traffic Data Using Advanced Computer Vision. Mathematics. 2024. V. 12. Is. 555. ISSN 2227-7390. DOI: https://doi.org/10.3390/math12040555.
- Meng M., Toan T.D., Wong Y.D., Lam S.H. Short-term travel-time prediction using support vector machine and nearest neighbor method. Transp. Res. Rec. 2022. V. 2676 (6). P. 353–365. DOI: http://dx.doi.org/10.1177/03611981221074371.
- Zhang W.S., Yao R.H. Traffic flow parameters estimation based on spatio-temporal characteristics and hybrid deep learning. J. Transporat. Syst. Eng. Inform. Technol. 2021. V. 21 (1). P. 82–89. DOI: http://dx.doi.org/10.16097/j.cnki.1009-6744.2021.01.013.