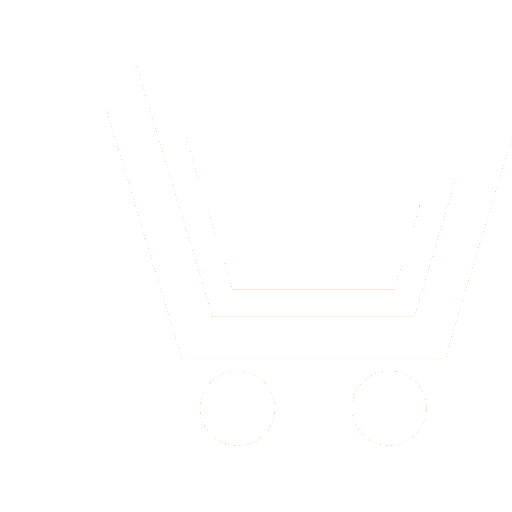
S.A. Korchagin1
1 Financial University under the Government of the Russian Federation (Moscow, Russia)
1 sakorchagin@fa.ru
Formulation of the problem. When training intelligent nanocomposite microscopy analysis systems, it is necessary to efficiently process a large amount of diverse data from various sources and provide reliable results. It is necessary to solve the problem of security and compliance with privacy policies when working with such data.
Target. To develop an intelligent system for analyzing nanocomposites, allowing not only to classify microscopy images with high accuracy, but also to ensure the confidentiality of research data.
Results. An intelligent system for analyzing microscopy of nanocomposites has been developed, based on federated machine learning and allowing the system to be trained locally, taking into account data confidentiality criteria. The intelligent system is based on a modified convolutional neural network VGG16. A horizontal federated machine learning architecture was used to ensure data confidentiality. A comparison of this system with classical machine learning methods was carried out. The accuracy of the model was 94.7%.
Practical significance. The development of an intelligent system for analyzing microscopy of nanocomposites using federated machine learning methods will significantly increase the efficiency of the analysis process. Automation and integration of various data sources will reduce the time spent on data processing and analysis, as well as improve the accuracy of the results obtained. Considering that data on the properties of nanocomposites can be confidential, the development of a system that takes into account security and confidentiality criteria is of great practical importance.
Korchagin S.A. Development of an intelligent system for analyzing microscopy of nanocomposites using federated machine learning methods. Nonlinear World. 2024. V. 22. № 4. P. 86–93. DOI: https://doi.org/10.18127/ j20700970-202404-11 (In Russian)
- Morgan D., Jacobs R. Opportunities and challenges for machine learning in materials science. Annual Review of Materials Research. 2020. V. 50. P. 71–103.
- Korchagin S., Osipov A., Pleshakova E. et al. Intelligent System for Express Analysis of Electrophysical Characteristics of Nanocomposite Media Studies in Computational Intelligence. 2022. V. 1032. SCI. P. 223–230. DOI 10.1007/978-3-030-96993-6_22.
- Deringer V.L., Caro M.A., Csanyi G. Machine learning interatomic potentials as emerging tools for materials science. Advanced Materials. 2019. V. 31. № 46. P. 1902765.
- Chen G. et al. Machine-learning-assisted de novo design of organic molecules and polymers: Opportunities and challenges Polymers. 2020. V. 12. № 1. P. 163.
- Deng X. et al. Large-scale screening and machine learning to predict the computation-ready, experimental metal-organic frameworks for CO2 capture from air. Applied Sciences. 2020. V. 10. № 2. P. 569.
- Alkhatib R. et al. A Brief Review of Machine Learning Algorithms in Forest Fires Science. Applied Sciences. 2023. V. 13. № 14. P. 8275.
- Korchagin S.A., Terin D.V., Klinaev Y.V., Romanchuk S.P. Simulation of Current-Voltage Characteristics of Conglom-erate of Nonlinear Semiconductor Nanocomposites. International Conference on Actual Problems of Electron Devices En-gineering (APEDE). Saratov. Russia. 2018. P. 397–399. DOI: 10.1109/APEDE.2018.8542433.
- Qiu Y., Myers D. R., Lam W.A. The biophysics and mechanics of blood from a materials perspective. Nature Reviews Materi-als. 2019. V. 4. № 5. P. 294–311.
- Ali S. et al. Computer vision based deep learning approach for the detection and classification of algae species using micro-scopic images. Water. 2022. V. 14. № 14. P. 2219.
- Bychkov A.G., Kiseleva T.V., Maslova E.V. Ispol'zovanie svertochnyh nejrosetej dlya klassifikacii izobrazhenij. Vestnik Sibirskogo gosudarstvennogo industrial'nogo universiteta. 2023. № 1 (43). S. 39–49 (In Russian).
- Korchagin S. et al. The use of machine learning methods in the diagnosis of diseases of crops. E3S Web of Conferences. EDP Sciences. 2020. V. 176.
- Okunev A.G. et al. Nanoparticle recognition on scanning probe microscopy images using computer vision and deep learning. Nanomaterials. 2020. V. 10. № 7. P. 1285.
- Luo C.Y. et al. A Computer Vision-Based Approach for Tick Identification Using Deep Learning Models. Insects. 2022. V. 13. № 2. P. 116.
- Saba T. Computer vision for microscopic skin cancer diagnosis using handcrafted and non‐handcrafted features. Microscopy Research and Technique. 2021. V. 84. № 6. P. 1272–1283.
- Holm E.A. et al. Overview: Computer vision and machine learning for microstructural characterization and analysis. Metallurgical and Materials Transactions A. 2020. V. 51. P. 5985–5999.
- Korchagin S. et al. Mathematical modeling of the electrophysical properties of a layered nanocomposite based on silicon with an ordered structure. Mathematics. 2021. V. 9. № 24. P. 3167.
- Osipov A., Pleshakova E., Gataullin S., Korchagin S., Ivanov M., Finogeev A., Yadav V. Deep learning method for recognition and classification of images from video recorders in difficult weather conditions. Sustainability. 2022. Т. 14. № 4.
- Strokova A.V. Provedenie eksperimentov s ryadom svertochnyh nejronnyh setej po ocenke tekhnik Super-Resolution. Grafikon-konferencii po komp'yuternoj grafike i zreniyu. 2023. T. 33. S. 88–96 (In Russian).
- Aparnev A.N., Barten'ev O.V. Analiz funkcij poter' pri obuchenii svertochnyh nejronnyh setej s optimizatorom Adam dlya klassifikacii izobrazhenij. Vestnik Moskovskogo energeticheskogo instituta (Vestnik MEI). 2020. № 2. S. 90–105 (In Russian).