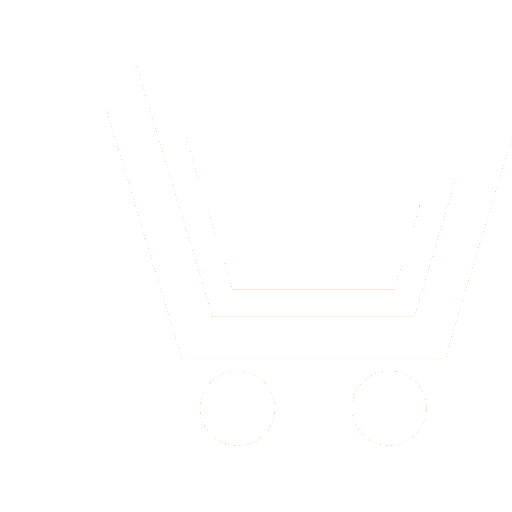
A.M. Poykalaynen1, R.A. Kochkarov2
1,2 Financial University under the Government of the Russian Federation (Moscow, Russia)
1 ampojkalajnen@fa.ru, 2 rkochkarov@fa.ru
Problem Statement. Graph neural networks (GNNs) typically assume homophily, which limits their efficiency under heterophily where the connected nodes are different.
Objective. The aim of this work is to analyze the performance of GNNs under heterophily and homophily using the AMUD-ADPA method on user action data on a massive open online courses (MOOC) platform.
Results. Existing methods for analyzing graphs under homophily and heterophily are investigated. It is determined how and why to use the AMUD-ADPA method to improve GNN performance. The features and differences of the AMUD-ADPA method and its application in analyzing temporal and dynamic networks are studied. The effectiveness of the method in predicting user behavior in online courses is shown.
Practical significance. The results of the study show that the AMUD-ADPA method can significantly improve the performance of GNNs in different topological conditions. This opens up new opportunities for data analysis in areas such as recommendation systems, where traditional methods are often insufficient. The application of the AMUD-ADPA method can lead to more accurate and adaptive models for processing complex graph structures.
Poykalaynen A.M., Kochkarov R.A. Heterophily and homophily in graphs: AMUD-ADPA model analysis on the MOOC dataset. Nonlinear World. 2024. V. 22. № 4. P. 56–62. DOI: https://doi.org/10.18127/ j20700970-202404-07 (In Russian)
- Jiayi Y., Sourav M., Wei Y. Incorporating Heterophily into Graph Neural Networks for Graph Classification. arXiv preprint arXiv:2203.07678. 2024. DOI: https://doi.org/10.48550/arXiv.2203.07678
- Sun H., Li X., Wu Z., Su D., Li R.-H., Wang G. Breaking the Entanglement of Homophily and Heterophily in Semi-supervised Node Classification. arXiv preprint arXiv:2312.04111. 2024. DOI: https://doi.org/10.48550/arXiv.2312.04111
- URL: https://snap.stanford.edu/data/act-mooc.html. (data obrashcheniya: 04.06.2024) (In Russian)
- Habr: Grafy v rekomendatel'nyh sistemah [chast' 1]. 2024. URL: https://habr.com/ru/articles/825896/ (data obrashcheniya: 06.06.2024)
- Habr: Ispol'zovanie metodov analiza grafov dlya poiska anomalij. 2020. URL: https://habr.com/ru/companies/lanit/articles/508176/ (data obrashcheniya: 06.06.2024) (In Russian)
- Prokopenko A.A. Primenenie teorii grafov k analizu social'nyh setej. Pedagogicheskij potencial: Materialy Vserossijskoj s mezhdunarodnym uchastiem nauch.-prakt. konf., Bryansk, 27 noyabrya 2023 goda. Bryansk: Bryanskij gosudarstvennyj inzhenerno-tekhnologicheskij universitet. 2023. S. 237–239 (In Russian).
- Kipf T.N., Welling M. Semi-Supervised Classification with Graph Convolutional Networks. arXiv preprint arXiv:1609.02907. 2017. DOI: https://doi.org/10.48550/arXiv.1609.02907
- Velickovic P., Cucurull G., Casanova A., Romero A., Lio P., Bengio Y. Graph Attention Networks. arXiv preprint arXiv:1710. 10903. 2018. DOI: https://doi.org/10.48550/arXiv.1710.10903
- Hamilton W., Ying R., Leskovec J. Inductive Representation Learning on Large Graphs. Advances in Neural Information Processing Systems. 2017. DOI: https://doi.org/10.48550/arXiv.1706.02216
- Habr: Giperparametricheskij poisk i optimizaciya modelej. 2023. URL: https://habr.com/ru/companies/otus/articles/754402/ (data obrashcheniya: 09.06.2024) (In Russian)