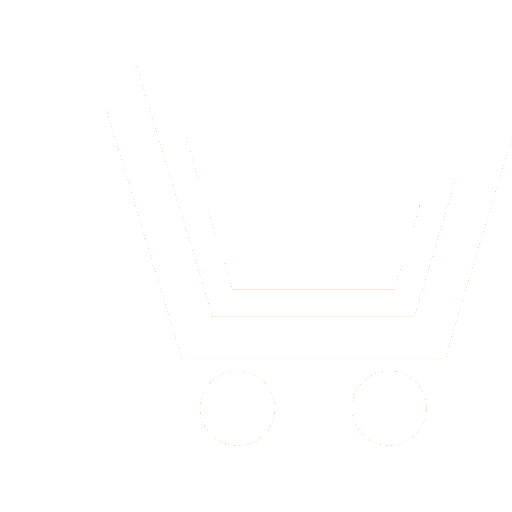
O.A. Saltykova1, V.D. Saushkin2
1,2 RUDN University named after. Patrice Lumumba (Moscow, Russia)
1 olga_a_saltykova@mail.ru; 2 kingrailag@gmail.com
This work examines the possibilities and advantages using neural networks to assess the competitive enterprise’s ability in the digital economy. This issue is relevant, considering the progressing digital economy development and the data accumulation on the enterprise’s activities. Analysis of this data with machine learning methods will make it possible to effectively assess the enterprises competitiveness and make effective management decisions that will bring the company to a high level not only in different regions, but also in the country. To achieve this goal, it is necessary to identify the main factors that have the greatest impact on the business. Such factors include, for example, the introduction electronic document management, business processes robotization using the RPA platform, or their automation using programming languages. The most popular and convenient programming languages for solving similar problems are Python, C#, PowerShell. The latter offers a good approach for communicating with Windows OS and automating system administration tasks.
Among the main factors influencing competitiveness, a total of 5 most significant factors were identified for the task: the financial position of the enterprise, the image of the organization, the quality of services, the personnel qualifications level and organization’s digitalization level. Competitiveness classes are proposed to determine the enterprise competitiveness. Three classes were defined for classification: Below_Norm, Normal, Above_Norm. To solve the problem, a multilayer neural network architecture has been developed, the implementation of which is implemented in Python. The use of such a solution makes it possible to achieve an accuracy of more than 90% when solving the classification task. To confirm the neural network functionality, a method for constructing a confusion matrix has been implemented. This matrix shows the percentage of objects that are accurately classified according to the incorrectly detected. Attached are the results of the neural network when solving the problem of classifying competitiveness. According to the data obtained, it was concluded that the values fed to the input classifier may be classified inaccurately, because they are on the boundaries of the results between the observed classes. The data for training neural networks was generated randomly and had a matrix size of 30x5. Since the purpose of the work is to argue for a machine learning approach to solve determining competitiveness problem, there was no need to use real data. The k-nearest neighbors method was chosen as the classification method, since this method shows high accuracy in solving such problems.
Saltykova O.A., Saushkin V.D. Advantages and machine learning role in assessing the enterprises competitiveness in the digital economy. Nonlinear World. 2024. V. 22. № 1. P. 22-21. DOI: https://doi.org/10.18127/ j20700970-202401-03 (In Russian)
- Ezhova V.A. Konkurentosposobnost' organizacii: Ucheb. posobie. SPb: VShTJe SPbGUPTD. 2022. 38 s. (In Russian).
- Martynova Ju.A. Metody mashinnogo obuchenija pri ocenke konkurentosposobnosti predprijatija. Voprosy innovacionnoj jekonomiki. 2020. T. 10. № 1. S. 549-562. (In Russian).
- Saushkin V.D., Beshmel'nov M.I., Lygarev Ja.S., Shmeleva A.G. Imitacionnoe modelirovanie faktorov konkurentosposobnosti predprijatija v cifrovoj jekonomike. Sovremennoe sostojanie, problemy i perspektivy razvitija otraslevoj nauki. M.: Izd-vo «Pero». 2021. S. 532-535 (In Russian).
- Koh R. Princip 80/20. Kak rabotat' men'she, a zarabatyvat' bol'she: Per. s angl. Lineva I. M.: Bombra. 2021. 432 s. (In Russian).
- Belkin V.N., Belkina N.A., Vladykina L.B. Teoreticheskie osnovy ocenki konkurentosposobnosti predprijatij. Jekonomika regiona. 2015. № 1. S. 145-155 (In Russian).
- Mahmudova G.N., Ashurov Z.A. Faktory konkurentosposobnosti nacional'nyh rynkov v uslovijah cifrovoj transformacii jekonomiki. Cifrovaja transformacija jekonomicheskih sistem i perspektivy. Sb. trudov 6 Vseross. nauch.-praktich. konf. s zarubezhnym uchastiem. SPb: «JeKOPROM». 2022. S. 113-116 (In Russian).
- Shirshikov S.P. Klassifikacija faktorov, vlijajushhih na konkurentosposobnost' malyh i srednih stroitel'nyh organizacij. Sankt-Peterburgskij gos. arhitekturno-stroitel'nyj un-t. Obshhestvoznanie i social'naja psihologija. 2022. S. 189-198 (In Russian).
- Cherkasov D.Ju., Ivanov V.V. Mashinnoe obuchenie. Nauka, tehnika i obrazovanie. 2018. № 5(46). S. 85-87 (In Russian).
- Levchenko K.M., Sych A.A. Nejronnye seti. BGUIR. 2022. S. 90-93 (In Russian).