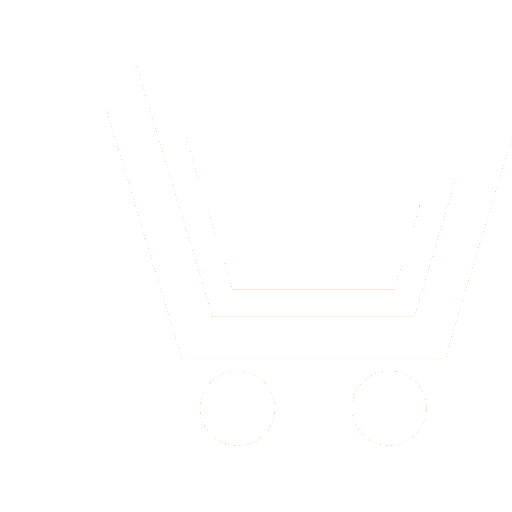
A.A. Zhurina1, S.V. Prokopchina2, P.E. Ryabov3, E.Yu. Shchetinin4
1-4 Financial University under the Government of the Russian Federation (Moscow, Russia)
1 alena21081998@yandex.ru; 2 svprokopchina@fa.ru; 3 peryabov@fa.ru; 4 eyshchetinin@fa.ru
Sentiment analysis of text in documents and social networks provides the opportunity for a prompt and adequate response, including for marketing purposes.
Explore methods for analyzing the sentiment of texts, identify strengths and weaknesses.
Well-known methods for analyzing the sentiment of texts are considered. Testing was carried out on data downloaded from a social network. Based on a detailed description of the algorithms' capabilities, it is possible to develop hybrid models or ensembles to improve forecast accuracy.
Zhurina A.A., Prokopchina S.V., Ryabov P.E., Shchetinin E.Yu. Sentiment analysis of text on social networks. Nonlinear World. 2023. V. 21. № 4. P. 55-68. DOI: https://doi.org/10.18127/j20700970-202304-07 (In Russian)
- Pavlov A.S., Dobrov B.V. Metod obnaruzhenija massovo porozhdennyh neestestvennyh tekstov na osnove analiza tematicheskoj struktury. Vychislitel'nye metody i programmirovanie: novye vychislitel'nye tehnologii. 2011. T. 12. S. 58–72 (in Russian).
- Janina A.O., Voroncov K.V. Mul'timodal'nye tematicheskie modeli dlja razvedochnogo poiska v kollektivnom bloge. Mashinnoe obuchenie i analiz dannyh. 2016. T. 2. № 2. S. 173–186 (in Russian).
- Adaskina Ju.V., Panicheva P.V., Popov A.M. Sentimentnyj analiz tvitov na osnove sintaksicheskih svjazej. Komp'juternaja lingvistika i intellektual'nye tehnologii: materialy ezhegodnoj Mezhdunar. konf. «Dialog». M.: Izd-vo RGGU. 2015. S. 25–35 (in Russian).
- Ajsina R.M. Obzor sredstv vizualizacii tematicheskih modelej kollekcij tekstovyh dokumentov. Mashinnoe obuchenie i analiz dannyh (http://jmlda.org). 2015. T. 1. № 11. S. 1584–1618 (in Russian).
- Kotel'nikov E.V., Klekovkina M.V. Avtomaticheskij analiz tonal'nosti tekstov na osnove metodov mashinnogo obuchenija. Komp'juternaja lingvistika i intellektual'nye tehnologii: trudy XVIII Mezhdunar. konf. «Dialog 2012». V 2-h tomah (Bekasovo, 30 maja – 3 2012 g.). M.: Izd-vo RGGU. 2012. Vyp. 11 (18). T. 2. S. 27–36 (in Russian).
- Tang X., Yang C., Wong Y., Wei C. Understanding Online Consumer Review Opinions with Sentiment Analysis using Machine Learning. Pacific Asia Journal of the Association for Information Systems. 2010. № 3(2). P. 73–89.
- Feng Y., Lapata M. Topic models for image annotation and text illustration. Human Language Technologies: The 2010 Annual Conference of the North American Chapter of the Association for Computational Linguistics. Association for Computational Linguistics, 2010. P. 831–839.
- Chuang J., Gupta S., Manning C., Heer J. Topic model diagnostics: Assessing domain relevance via topical alignment. Proceedings of the 30th International Conference on Machine Learning (ICML-13). Ed. by S. Dasgupta, D. Mcallester. JMLR Workshop and Conference Proceedings. 2013. V. 28. P. 612–620.
- Batura T.V. Metody avtomaticheskoj klassifikacii tekstov. Programmnye produkty i sistemy. 2017. T. 30. № 1. S. 85–99 (in Russian).
- Garshina V.V., Kalabuhov K.S., Stepancov V.A., Smotrov S.V. Razrabotka sistemy analiza tonal'nosti tekstovoj informacii. Vestnik VGU. Ser. Sistemnyj analiz i informacionnye tehnologii. 2017. № 3. S. 185-194. [Jelektronnyj resurs] URL: http://www.vestnik.vsu.ru/pdf/analiz/2017/03/2017-03-21.pdf (data obrashhenija: 19.12.2023) (in Russian).
- Kotel'nikov E.V., Klekovkina M.V. Opredelenie vesov ocenochnyh slov na osnove geneticheskogo algoritma v zadache analiza tonal'nosti 28 tekstov. Programmnye produkty i sistemy, 2013. Vyp. 4. S. 296–301 (in Russian).
- Rubcova Ju.V. Postroenie korpusa tekstov dlja nastrojki tonovogo klassifikatora. Programmnye produkty i sistemy. Novosibirsk: Nauchno-issledovatel'skij institut «Centrprogrammsistem». 2015 № 109. S. 72–78 (in Russian).
- Klekovkina M.V., Kotel'nikov E.V. Metod avtomaticheskoj klassifikacii tekstov po tonal'nosti, osnovannyj na slovare jemocional'noj leksiki. Trudy XIV Vseross. nauch. konf. «Jelektronnye biblioteki: perspektivnye metody i tehnologii, jelektronnye kollekcii» (RCDL). Pereslavl'-Zalesskij: Izd-vo «Universitet goroda Pereslavl'». 2012. S. 118–123 (in Russian).
- Frei O., Apishev M. Parallel non-blocking deterministic algorithm for online topic modeling. AIST’2016, Analysis of Images, Social networks and Texts. Springer International Publishing Switzerland, Communications in Computer and Information Science (CCIS). 2016. V. 661. P. 132–144.
- Osnovy Natural Language Processing dlja teksta. [Jelektronnyj resurs] URL: https://habr.com/ru/company/Voxim-plant/blog/446738/ (data obrashhenija: 19.12.2023) (in Russian).
- Obuchaem komp'juter chuvstvam (sentiment analysis po russki) [Jelektronnyj resurs] URL: https://habr.com/ru /post/149605/ (data obrashhenija: 19.12.2023) (in Russian).
- Avtomaticheskoe opredelenie tonal'nosti teksta (Sentiment Analysis). [Jelektronnyj resurs] URL: https://habr.com/ru/post/263171/ (data obrashhenija: 19.12.2023) (in Russian).
- Matematicheskie metody analiza tekstov. Seminar 3. Klassifikacija tekstov. Analiz tonal'nosti. [Jelektronnyj resurs] UR: http://www.machinelearning.ru/wiki/images/6/64/Mel_lain_msu_nlp_sem_3.pdf (data obrashhenija: 19.12.2023) (in Russian).
- Matematicheskie metody analiza tekstov. Vektornye predstavlenija slov [Jelektronnyj resurs] URL: word2vechttp://www.machinelearning.ru/wiki/images/b/b3/Word2Vec.pdf (data obrashhenija: 19.12.2023) (in Russian).
- Voroncov K.V., Potapenko A.A. Modifikacii EM-algoritma dlja verojatnostnogo tematicheskogo modelirovanija. Mashinnoe obuchenie i analiz dannyh. 2013. T. 1. № 6. S. 657–686 (in Russian).
- Voroncov K.V., Potapenko A.A. Reguljarizacija verojatnostnyh tematicheskih modelej dlja povyshenija interpretiruemosti i opredelenija chisla tem. Komp'juternaja lingvistika i intellektual'nye tehnologii: materialy ezhegodnoj Mezhdunar. konf. «Dialog» (Bekasovo, 4–8 ijunja 2014 g.). Vyp. 13 (20). M: Izd-vo RGGU. 2014. S. 676–687 (in Russian).
- SVM. Ob’jasnenie s nulja i realizacija na python. Podrobnyj razbor metoda opornyh vektorov. [Jelektronnyj resurs] URL: https://habr.com/ru/company/ods/blog/484148/ (data obrashhenija: 19.12.2023) (in Russian).
- Kratkij obzor algoritma mashinnogo obuchenija Metod Opornyh Vektorov (SVM). [Jelektronnyj resurs] URL: https://habr.com/ru/post/428503/ (data obrashhenija: 19.12.2023) (in Russian).
- Bodrunova S., Koltsov S., Koltsova O., Nikolenko S. I., Shimorina A. Interval semisupervised LDA: Classifying needles in a haystack. MICAI (1). Ed. by F.C. Espinoza, A.F. Gelbukh, M. Gonzalez-Mendoza. V. 8265 of Lecture Notes in Computer Science. Springer. 2013. P. 265–274.
- Kataria S., Mitra P., Caragea C., Giles C.L. Context sensitive topic models for author influence in document networks. Proceedings of the Twenty-Second international joint conference on Artificial Intelligence. IJCAI’11. AAAI Press, 2011. V. 3. P. 2274–2280.
- Liu J., Shang J., Wang C., Ren X., Han J. Mining quality phrases from massive text corpora. Proceedings of the 2015 ACM SIGMOD International Conference on Management of Data. SIGMOD ’15. New York, NY, USA: ACM. 2015. P. 1729–1744.
- Shang J., Liu J., Jiang M., Ren X., Voss C.R., Han J. Automated phrase mining from massive text corpora. CoRR. 2017. V. abs/1702.04457.
- Fuller C.M., Biros D.P. and Delen D. An investigation of data and text mining methods for real world deception detection. Expert Systems with Applications. 2011. № 38. P. 8392–8398.
- Grant C.E., George C.P., Kanjilal V., Nirkhiwale S., Wilson J.N., Wang D.Z. A topic-based search, visualization, and exploration system. FLAIRS Conference. AAAI Press. 2015. P. 43–48.