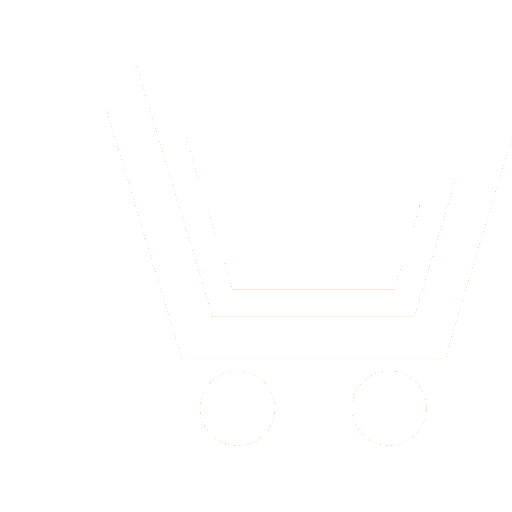
S.N. Cherkashin1, I.A. Petrov2
1,2 Financial University under the Government of the Russian Federation (Moscow, Russia)
Problem Statement. The rapid development of artificial intelligence (AI) technology has opened new possibilities in the field of penetration testing, offering pentesters several tools and opportunities to improve the efficiency and re-efficacy of their work. However, it is important to explore the positive and negative aspects of using AI as a pentester's assistant to understand its true potential and limitations.
Objective. To explore the role of AI in helping pentesters, highlighting benefits such as automatic vulnerability detection, increased efficiency, intelligent exploitation, and decision support. To consider the problems associated with false positives and negative results, limited understanding of context, the use of AI by hackers, and the risk of over-reliance on automation.
Results. Analyzing the positives and negatives, provides insight into the balanced use of AI as a helper for pentesters, considering the changing cybersecurity landscape and emphasizing the importance of human experience and manual testing methods.
Practical Significance. By understanding the capabilities and limitations of AI tools in penetration testing, penetration testing professionals can make informed decisions about incorporating AI into their workflows. In addition, AI developers and researchers can use the work as a benchmark for the development of AI methods, which will ultimately lead to the creation of more efficient and reliable AI penetration testing tools.
Cherkashin S.N., Petrov I.A. The role of AI as a helper for pentesters: study of positive and negative aspects. Nonlinear World. 2023. V. 21. № 3. P. 46-53. DOI: https://doi.org/10.18127/j20700970-202303-05 (In Russian)
- Begaev A. N., Begaev S. N., Fedotov V. A. Testirovanie na proniknovenie. Uchebnoe posobie universiteta ITMO. 2018. S. 45. EDN: OOEGKN (in Russian).
- Myasnikov A.V. Primenenie tekhnologij mashinnogo obucheniya dlya optimizacii processa testirovaniya na proniknove-nie. Problemy informacionnoj bezopasnosti. Komp'yuternye sistemy. 2019. №2. S. 9–15. EDN: EZKGMT (in Russian).
- Poltavtseva M. A., Pechenkin A. I. Intelligent data analysis in decision support systems for penetration tests. Automatic Con-trol and Computer Sciences. 2017. № 51 (8). P. 985-991. EDN: XXLVYD.
- OWASP Benchmark. URL: https://owasp.org/www-project-benchmark/ (data obrashcheniya: 28.05.2023).
- Maksimenkova V. S. Avtomatizaciya testirovaniya na proniknovenie. Studencheskij vestnik. 2022. №42–8(234). S. 33–34. EDN: QHIRVL (in Russian).
- Kutuev T.M. Primenenie iskusstvennogo intellekta pri reshenii zadach informacionnoj bezopasnosti. Informacionnye tekhnologii obespecheniya kompleksnoj bezopasnosti v cifrovom obshchestve. 2021. S. 197–200. EDN: TYUJZC (in Russian).
- Svishchyova I. V., Hlopovskaya A. V. Bezopasnaya razrabotka programmnogo obespecheniya. testirovanie na proniknovenie. Moya professional'naya kar'era. 2022. №36. S. 54–62. EDN: CDANIE (in Russian).
- SHkradyuk A. D. Ocenka bezopasnosti informacionnyh sistem s pomoshch'yu testirovaniya na proniknovenie. Umnaya cifrovaya ekonomika. 2022. №4. S. 18–30. EDN: TSHJTT (in Russian).
- Myasnikov A. V. Primenenie mashinnogo obucheniya s podkrepleniem v zadache testirovaniya na proniknovenie. Sovre-mennaya nauka: aktual'nye problemy teorii i praktiki. seriya: estestvennye i tekhnicheskie nauki. 2020. №11. S. 104–107. EDN: DZRISR (in Russian).
- Belyanova I. A., Pojmanova E. D. Testirovanie na proniknovenie veb-prilozhenij. Informacionnye tekhnologii v obrazovanii. 2021. S. 44–47. EDN: KEVECI (in Russian).