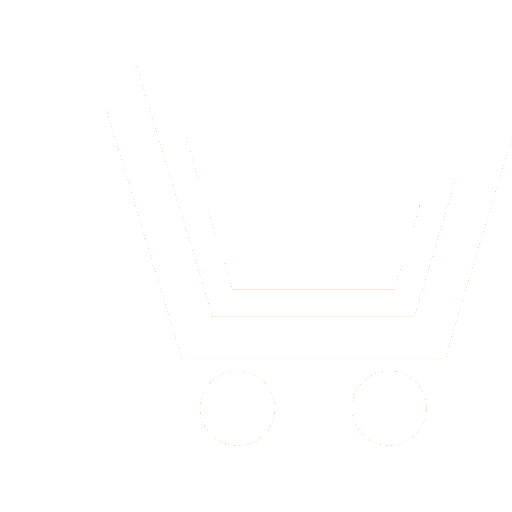
A.P. Ryzhkov1, O.N. Katkov2, N.A. Safronova3
1-3 FSSEIHVT Academy of Federal Agency of Protection of Russia, The employee of Academy (Orel, Russia)
Most of the most common hybrid codecs based on linear prediction use the method of «analysis through synthesis», which provides one or more codebooks to generate the necessary excitation signal. The use of codebooks led to the creation of a class of codecs, collectively called CELP (method of linear prediction of a speech signal with code excitation) and used for low-speed speech transmission. The urgency of improving the procedure for synthesizing code books with vector quantization in speech signal encoding systems at low transmission rates (up to 24 kbit/s) is explained, firstly, by the current demand for such a range of speech encoding, and secondly, by achieving higher quality indicators when solving problems related to quality the synthesized speech signal or the transmission rate.
The hypothesis of a possible improvement in the quality of speech synthesis is associated with the study of the relationships between the vectors of the elements of the decomposition of the speech signal – the excitation signal and the parameters of the transfer function of the vocal tract. The use of the dependencies of the speech signal separation elements in linear prediction makes it possible to improve the coding process, since most of the works in this area of research used independent processing and coding of the spaces of representations of these parameters.
A feature of the system is the application of the procedure for dividing PC segments into a limited number of classes, since the implementation of the hypothesis of the interdependence of the elements of the PC decomposition provides for the use of classified vector quantization systems adequate under the conditions of the proposed optimization. The speech segment classification algorithm, which uses the analysis of statistical and parametric characteristics of the speech signal, consists of three stages. For each type of segment, its own neural networks are used – classifiers of radial basis functions RBF. At the stage of functioning, pre-trained neural networks are used with a finite number of classes of partitions of the parameter spaces of the decomposition of segments. The stage of training neural networks for the coefficients of the synthesis model and excitation signals allows us to determine the interdependence between these decomposition parameters. There is a relationship between the classification space (cells) of the coefficients of the forming model and the vector space of the linear prediction error signal (the excitation signal at reception), which can be used to reduce the transmission rate
Thus, based on the analysis of the properties of the speech signal and the use of neural network technologies, algorithms have been created focused on the transmission rate of 4.8 kbit/s, the functioning of the coding system, which differ from the known solutions in that they implement procedures for restructuring the parameters and structure of the system using procedures for vector quantization of elements of the decomposition of segments of the speech signal based on neural classifier networks.
Testing of the developed algorithms was performed on real PC with a duration of at least 15 minutes for 13 speakers.
Ryzhkov A.P., Katkov O.N., Safronova N.A. Speech signal encoding and decoding algorithms based on interdependent codebooks. Nonlinear World. 2023. V. 21. № 3. P. 5-15. DOI: https://doi.org/10.18127/j20700970-202303-01 (In Russian)
- Markel Dzh.D, Grej A.H. Linejnoe predskazanie rechi. M.: Svjaz'. 1980. 308 s. (In Russian).
- Sheluhin O.I., Luk'jancev N.F. Cifrovaja obrabotka i peredacha rechi. M.: Radio i svjaz'. 2000. 456 s. (In Russian).
- Hajkin S. Nejronnye seti: polnyj kurs. Izd. 2-e. M.: ID «Vil'jams». 2006. 1104 s. (In Russian).
- Bykov S.F., Zhuravlev V.I., Shalimov I.A. Cifrovaja telefonija: Ucheb. posobie dlja vuzov. M.: Radio i svjaz'. 2003. 144 s. (In Russian).
- Afanas'ev A.A., Vlasov R.S. Parametricheskaja identifikacija sintezirujushhej sistemy golosovogo trakta na vydeljaemyh odnorodnyh segmentah analiza rechevogo signala. Informacionnye sistemy i tehnologii. 2020. № 2(118). S. 5–12 (In Russian).
- Afanas'ev A.A. Nepreryvnaja autentifikacija diktora pri vedenii telefonnyh peregovorov po nizkoskorostnym cifrovym kanalam. Voprosy kiberbezopasnosti. 2016. № 3(16). S. 60–67 (In Russian).
- Ryzhkov A.P., Dviljanskij A.A., Safronova N.A. Matematicheskaja model' kodeka dlja sistem s rechevym upravleniem. Promyshlennye ASU i kontrollery. 2021. № 6. S. 17–25 (In Russian).
- Vlasov R.S., Siren'kij E.I., Afanas'ev A.A. Identifikacija rechevyh pauz v uslovijah slozhnoj pomehovoj obstanovki. Sb. nauch. trudov II Mezhdunar. nauch.-praktich. konf. «Infokommunikacionnye tehnologii: aktual'nye voprosy cifrovoj jekonomiki». Ekaterinburg: Ural'skij tehnicheskij institut svjazi i informatiki (filial) FGOBU VPO «Sibirskij gosudarstvennyj universitet telekommunikacij i informatiki». 2022. S. 27–32 (In Russian).
- Ryzhkov A.P., Novikov D.A. Ispol'zovanie nejrosetevyh tehnologij sozdanija kodovyh knig i poiska v nih pri vektornom kvantovanii dannyh v zadachah nizkoskorostnogo rechevogo kodirovanija. Nejrokomp'jutery: razrabotka, primenenie. 2015. № 3. S. 19–28 (In Russian).
- Afanas'ev A.A., Ryzhkov A.P. Metod snizhenija skorosti peredachi v vokoderah s linejnym predskazaniem na osnove primenenija nejronnyh setej pri peremennoj dline segmenta analiza. Informacionnye sistemy i tehnologii. 2012. № 6 (74). S. 20–28 (In Russian).
- Afanas'ev A.A., Vlasov R.S., Lisichkin V.G., Pitolin A.V. Algoritmy obrabotki rechevogo signala pri peremennoj dlitel'nosti segmenta analiza. Vestnik Voronezhskogo gosudarstvennogo tehnicheskogo universiteta. 2019. T. 15, № 4. S. 41–48 (In Russian).