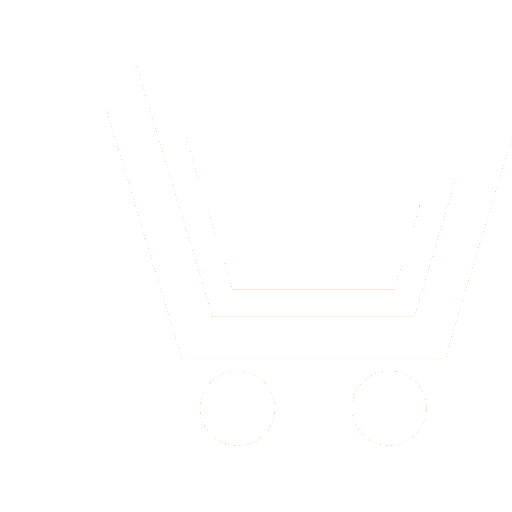
A.A. Potapov1
1 Lomonosov Moscow State University (Moscow, Russia)
The article presents practical implementation of energy detection method based upon non-parametric statistics computed using periodic spectrum samples provided by measuring equipment. The method enables efficient detection and monitoring for signals with low or negative signal-to-noise ratio.
The method's sensitivity is limited by measuring equipment inherent noise fluctuations and can be a priory experimentally established for certain experimental hardware settings and desirable spectrum samples lengths. Sensitivity thresholds (in terms of signal-to-noise ratio) for reliable (with probability > 0,98) signal detection for a typical spectrum analyzer used in experiment varied from –11 dB to + 0,6 dB for spectrum samples lengths ranged between 30 000 and 470 spectrums respectively. The suggested energy detection method can be used for unstable and intermittent signals detection, which are active (or above sensitivity threshold) only for a fraction of spectrum sample recording time. The method is independent of signal's modulation (if any is used), amplitude variability profile and signal's probability distribution features.
Experimentally determined sensitivity threshold levels for real radio frequency signals coincided within 1,9 dB tolerances with corresponding levels estimated from spectrum analyzer inherent noise fluctuations for all implemented spectrum samples lengths. The data recording time for abovementioned spectrum samples lengths ranged between 207 and 3,2 seconds respectively and was entirely hardware-dependent parameter.
Experiment proved equal efficiency and reliability of the suggested method for reliable detection for both white noise signal (generated by analog generator) and broadcasted LTE signal (generated by cellular base stations), which were affected by multi-path propagation effects and average signal level instability due to subscribers time-varying activity.
The experiment showed the proposed energy detection method besides detection of low-level radio frequency signals (down to –11 dB SNR) provides highly reliable assessment of the detected signal's signal-to-noise ratio with 0,6 dB tolerance and 0,95 probability.
The energy detection method demonstrated zero level of false detections when there was no signal at the spectrum analyzer input (the input port of the instrument was terminated by a matched load), which is essential for method applicability in tasks of highly reliable detection of low-level signals from various types of sources.
Taking into account specifications of available hardware, required sensitivity level and limits for data recording time it is possible to choose optimal length of spectrum sample for the energy detection method, which would be the most reasonable for any task in question.
The energy detection method based upon non-parametric statistics computed using periodic spectrum samples can be effectively used in detection and radiomonitoring of low-level signals, in radio frequency electromagnetic compatibility research tasks and radio propagation path properties analysis in high loss environment.
Potapov A.A. Low-level signals radiomonitoring using periodic spectrum samples analysis. Nonlinear World. 2021. V. 19. № 3. 2021. P. 5−17. DOI: https://doi.org/10.18127/j20700970-202103-01 (In Russian)
- Koncepcija razvitija sistemy kontrolja za izluchenijami radiojelektronnyh sredstv i (ili) vysokochastotnyh ustrojstv grazhdanskogo naznachenija v Rossijskoj Federacii na period do 2025 goda: utv. resheniem GKRCh ot 4 ijulja 2017 g. № 1742-06. Veb-sajt Ministerstva cifrovogo razvitija, svjazi i massovyh kommunikacij Rossijskoj Federacii. M., 2018. URL: https://digital.gov.ru/ru/documents/5659/#tdownloadblock (data obrashhenija: 15.04.2021) (In Russian).
- Wiles E., Negus K. Long-term spectrum monitoring and occupancy from 174 to 1000 MHz in rural western Montana. 12th European Conference on Antennas and Propagation (EuCAP 2018). London: IET. 2018. P. 1–5. DOI: 10.1049/cp.2018.1054.
- Homssi B.A., Al-Hourani A., Evans R. J., Chavez K. G., Kandeepan S., Rowe W., Loney M. Free spectrum for IoT: How much can it take?. 2018 IEEE International Conference on Communications Workshops (ICC Workshops). Kansas City: IEEE. 2018. P. 1–6. DOI: 10.1109/ICCW.2018.8403749.
- Saavedra Melo M.A., Ramirez Arroyave G.A., Araque Quijano J.L. Spectrum occupation assessment in Bogota and opportunities for cognitive radio systems. 2018 IEEE-APS Topical Conference on Antennas and Propagation in Wireless Communications (APWC). Cartagena: IEEE, 2018. P. 932–935. DOI: 10.1109/APWC.2018.8503802.
- Mostafa M.G., Haralambous H. Wideband channel availability statistics over the high frequency spectrum in Cyprus //2018 2nd URSI Atlantic Radio Science Meeting (AT-RASC). Meloneras. IEEE. 2018. P. 1–4. DOI: 10.23919/URSI-AT-RASC.2018.8471564.
- Handbook of cognitive radio /Zhang W. – Ed. Singapore: Springer Nature. 2019. 2048 p.
- Mandloi M., Gurjar D., Pattanayak P., Nguyen H. (Eds.) 5G and beyond wireless systems: PHY layer perspective. Singapore: Springer Nature. 2021. 410 p.
- Kumar A., Thakur P., Pandit S., Singh G. Performance analysis of different threshold selection schemes in energy detection for cognitive radio communication systems. 2017 Fourth International Conference on Image Information Processing (ICIIP). Shimla. IEEE. 2017. P. 1–6. DOI: 10.1109/ICIIP.2017.8313702.
- Kumar A., Thakur P., Pandit S., Singh G. Analysis of optimal threshold selection for spectrum sensing in a cognitive radio network: an energy detection approach. Wireless Networks. 2019. № 25. P. 3917–3931.
- Suneel A.S., Shiyamala S. Peak detection based energy detection of a spectrum under Rayleigh fading noise environment. Journal of Ambient Intelligence and Humanized Computing. 2021. № 12. P. 4237–4245.
- Lorincz J., Ramljak I., Begušić D. A review of the noise uncertainty impact on energy detection with different OFDM system designs. Computer Communications. 2019. № 148. P. 185–207.
- Mahendru G., Shukla A., Banerjee P. A novel mathematical model for energy detection based spectrum sensing in cognitive radio networks. Wireless Personal Communications. 2020. № 110. P. 1237–1249.
- Sarala B., Rukmani Devi S., Sheela J.J. Spectrum energy detection in cognitive radio networks based on a novel adaptive threshold energy detection method. Computer Communications. 2020. № 152. P. 1–7.
- Verma P. Adaptive threshold based energy detection over Rayleigh fading channel. Wireless Personal Communications. 2020. № 113. P. 299–311.
- Meeker W.Q., Hahn G.J., Escobar L.A. Statistical intervals: A guide for practitioners and researchers. 2nd. Ed. Hoboken: John Wiley & Sons, Ltd. 2017. 592 p.
- Zaharov P.N., Korolev A.F., Potapov A.A., Turchaninov A.V. Issledovanie radiosignalov bazovyh stancij sotovoj svjazi s primeneniem giperspektral'nogo radiomonitoringa. Nelinejnyj mir. 2018. T. 16. № 4. S. 29−40 (In Russian).