350 rub
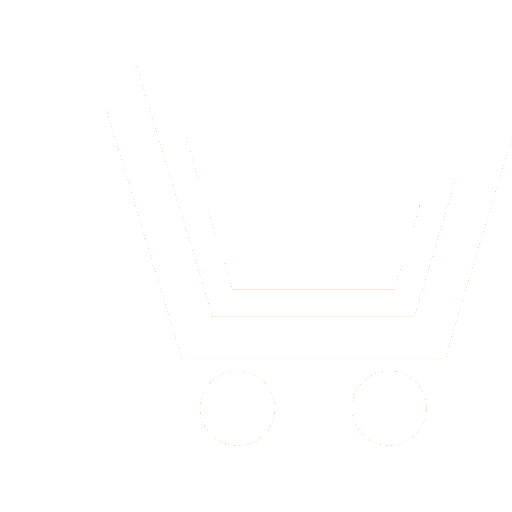
Journal Nonlinear World №8 for 2015 г.
Article in number:
Adaptive estimation of parameters of distribution
of non-Gaussian interferences through the application
of the EM algorithm and its modifications
Authors:
A.F. Nadeev - Dr.Sc. (Phys.-Math.), Professor, Director, Institute of Radioelectronics and Telecommunications, Kazan National Research Technical University named after A.N. Tupolev (KNRTU-KAI). E-mail: afnadeev@kai.ru
I.A. Podkurkov - Post-graduate Student, Kazan National Research Technical University named after A.N. Tupolev (KNRTU-KAI). E-mail: podkiva@mail.ru
Abstract:
One of the defining trends in modern wireless communications is the development and implementation of the concept of Cognitive Radio (CR). An important part of this concept is the ability of a radio system to take into account possible signals and interferences, which can be found in the radio channels, and use it to appropriately adapt procedures of information exchange, such as signal processing.
It is known that the widespread Gaussian models (Additive White Gaussian noise, AWGN) and the corresponding correlation methods do not exhaust the whole variety of signals and interferences, existing in real radio channels. The models in the form of mixtures of Gaussian distributions allow us to adequately represent arbitrary distributions of interference and noise in real channels, thus allowing to synthesize algorithms of reception of radio signals adequate to noise and interference.
To solve the problem of adaptation, i.e. estimation of the parameters of the poliGaussian model of interference, the so-called EM-algorithm was used. The EM-algorithm ("E" means expectation and "M" means maximization) is an iterative procedure of processing the data sample with distribution given by a probabilistic mixture. However, the-EM algorithm has weaknesses, which, if we use it, can significantly affect the construction of adaptive signal receiving schemes. Performance of EM-algorithm strongly depends on the choice of a starting point. Also, another disadvantage of EM procedure for use in adaptive schemes is absence of direct mechanism of estimation of the number of the components in a mixture, which, in general, can be arbitrary.
Iterative methods require a lot of computational resources and it is difficult to use them in real-time applications.
Recursive modifications of the EM algorithm are more appropriate in such circumstances as they allow estimation of the para-meters of the mixture at the same time with the arrival of a new signal sample and classification of that sample. Such methods are based on the simultaneous amendment of existing estimates of the mixture parameters, taking into account newly received signal sample.
This paper describes the formulation of the EM algorithms along with it-s recursive modification; the problem of modeling of recursive EM algorithm is solved; the results of the comparative analysis of iterative and recursive EM algorithms are provided. As a criteria for evaluation of the performance of the algorithms the distance metrics between the probability density functions are used: the root mean square (RMS) distance and the Kullback-Leibler distance (or Kullback-Leibler divergence).
The algorithm was modeled in a visual programming environment LabView. The simulation results indicate that the recursive EM-algorithm can be used in adaptive signal receiving procedures with non-Gaussian noise. Due to the relatively low amount of required computational resources, this algorithm allows to estimate the parameters of the distributions of interference and noise at the same time with the arrival of new signal realization. The accuracy of estimates achieved with recursive modification may be equivalent to 1015 iterations of unmodified (i.e. iterative) EM-algorithm.
Pages: 64-72
References
- CHabdarov SH.M., Trofimov A.T. Poligaussovy predstavlenija proizvolnykh pomekh i priem diskretnykh signalov // Radiotekhnika i ehlektronika. 1975. T. 20. № 4. S. 734-735.
- CHabdarov SH.M. Smesi sluchajjnykh javlenijj v statisticheskojj radiotekhnike // Vestnik Kazanskogo gosudarstvennogo tekhnicheskogo universiteta im. A.N. Tupoleva. 2008. № 4. S. 26-30.
- CHabdarov SH.M., Nadeev A.F., Fajjzullin R.R., Efimov E.N., Adnan A. Polikorreljacionnaja raspredelennaja adaptivnaja obrabotka signalov na fone negaussovskikh pomekh // Nelinejjnyjj mir. 2009. № 5. T. 7. S. 355-359.
- Dempster A.P., Laird N.M., Rubin D.B. Maximum likelihood from incomplete data via the EM algorithm // J. Roy. Stat. Soc. (Series B). Jan. 1977. V. 39. № 1. R. 1-38.
- Ajjvazjan S.A., Bukhshtaber V.M., Enjukov I.S., Meshalkin L.D. Prikladnaja statistika: Klassifikacija i snizhenie razmernosti: sprav. izd. / Pod red. S.A. Ajjvazjana. M.: Finansy i statistika. 1989. 607 s.
- McLachlan G.J., Krishnan T. The EM Algorithm and Extensions. Wiley-Interscience. 2008.
- McLachlan G., Peel D. Finite Mixture Models. John Wiley and Sons. 2000.
- Titterington D.M. Recursive Parameter Estimation Using Incomplete Data // J. Royal Statistical Soc. Series B (Methodological). 1984. V. 2. № 46. R. 257-267.
- Zivkovic Z., van der Heijden F. Recursive Unsupervised Learning of Finite Mixture Models // IEEE Trans. on PAMI. 2004. V. 26. № 5.
- Chavali V.G., da Silva C.R.C.M. Maximum-likelihood classification of digital amplitude phase modulated signals in flat fading non-Gaussian channels // IEEE Trans. Commun. 2011. V. 59. № 8. R. 2051-2056.