350 rub
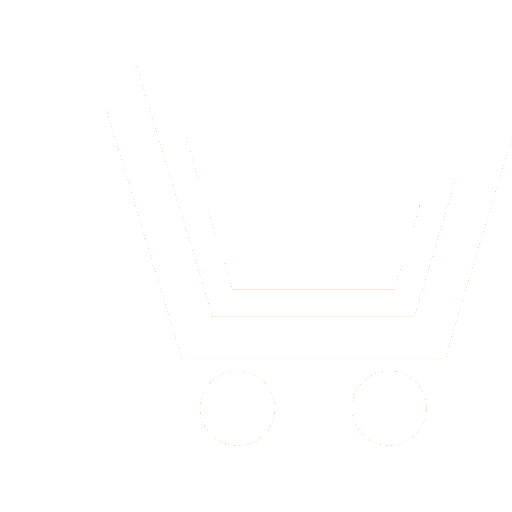
Journal Nonlinear World №6 for 2015 г.
Article in number:
Algorithm of quadrotor vehicle control based on neural network for typical trajectories
Authors:
V.Е. Pavlovsky - Dr.Sc. (Phys.-Math.), Professor, Chief Research Scientist, Keldysh Institute of Applied Mathematics of RAS (Moscow). E-mail: vlpavl@mail.ru
A.V. Savitskiy - Post-graduate Student, Lomonosov Moscow State University. E-mail: as@ttorr.ru
Abstract:
This paper presents a theoretical mechanical model of an unmanned aerial vehicle - quadrotor. There are the description of the lifting power and the moment of resistance of the rotor in the first part of this paper. Using the Lagrange equations it was described the dynamics of quadrotors in general. Such basic trajectories as take-off, landing, hovering, flying in a straight line were studied. A convergent neural network algorithm of control was considered.
A lot of effects and impacts that are difficult to model mathematically appear in-flight of multirotor robot. The neural network controller solves this type of problems. Neural controller consists of three blocks: the sensor unit, the unit of the neural network, and integrating unit. Based on the results of numerical simulation it was built a training set and а neural network. It is shown that the constructed control algorithm satisfactorily controls the rotation angle in the case of basic trajectories. The results of the neural network algorithm for the case of the presence of random noise and errors in the position sensor were also presented. It is shown that the neural controller works satisfactorily for take-off even if the height sensor has error of 5 cm.
Pages: 47-54
References
- Avila Vilchis J.C., Brogliato B., Dzulc A., Lozano R. Nonlinear modelling and control of helicopters // Automatica. 2003. V. 39. P. 1583 - 1596.
- Dzul P.A., Lozano R. Real-time stabilization and tracking of a four-rotor mini rotorcraft // IEEE Transaction on Control System Technology. 2004. V. 12(4). P. 510 - 516.
- Bresciani T. Modelling, Identification and Control of a Quadrotor Helicopter. Department of Automatic Control, Lund University. 2008.
- Fantoni I., Lozano R. Nelinejjnoe upravlenie mekhanicheskimi sistemami s deficitom upravljajushhikh vozdejjstvijj. M.-Izhevsk: OOO «Kompjuternaja dinamika». 2012. 312 s.
- Evgenov A.A. Nejjrosetevojj reguljator sistemy upravlenija kvadrokopterom // Sovremennye problemy nauki i obrazovanija. 2013. №5. 7 s.
- Madani T., Benallegue A. Adaptive Control via Backstepping Technique and Neural Networks of a Quadrotor Helicopter // Proceedings of the 17th World Congress. The International Federation of Automatic Control. Seoul, Korea. July 6-11 2008. P. 6513-6518.
- Hoffmann G.M., Huang H., Waslander S.L., Tomlin C.J. Quadrotor helicopter flight dynamics and control: Theory and experiment, Proceedings of the AIAA Guidance // Navigation and Control Conference and Exhibit. Aug. 2007. 20 p.
- Pounds P., Mahony R., Corke P. Modelling and Control of a Quad-Rotor Robot. Canberra, Australian National University. 2008, 10 p.
- Boudjedir Hana, Yacef Fouad Dual neural network for adaptive sliding mode control of quadrotor helicopter stabilization // International Journal of Information Sciences and Techniques (IJIST). July 2012. V. 2. № 4. 14 p.
- Nicol C., Macnab C.J.B., Ramirez-Serrano A. Robust neural network control of a quadrotor helicopter // Mechatronics. September 2011. V. 21. № 6. P.927-938.
- Dierks T., Jagannathan S. Neural Network Control and Wireless Sensor Network-based Localization of Quadrotor UAV Formations // Aerial Vehicles. 2009. P. 601-620.
- Munoz R.S.M., Rossi C., Cruz A.B. Modelling and Identification of Flight Dynamics in Mini-Helicopters Using Neural Networks // Aerial Vehicles. 2009. P. 287-312.
- Lavi B. An Adaptive Neuro PID for Controlling the Altitude of quadcopter Robot // International Conference on Methods and Models in Automation and Robotics. Poland. 2014. V. 18. P. 662-665.