350 rub
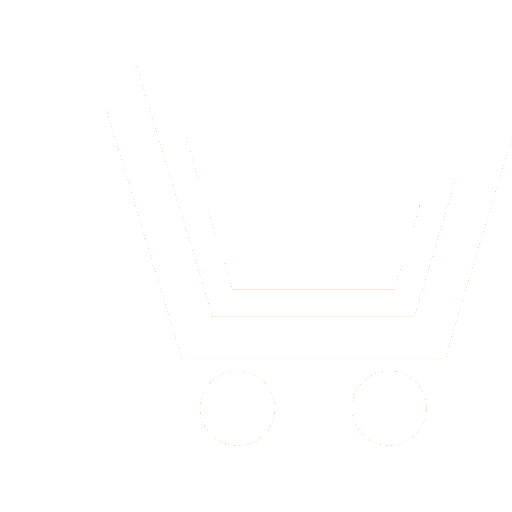
Journal Nonlinear World №3 for 2015 г.
Article in number:
Analysis of the forecasting models of corporate network server processes
Authors:
M.A. Basarab - Dr.Sc. (Phys.-Math.), Professor, Department of «Theoretical Informatics and Computer Technologies», Bauman Moscow State Technical University
I.P. Ivanov - Dr.Sc.(Eng.), Head of Department of «Theoretical Informatics and Computer Technologies», Bauman Moscow State Technical University
A.V. Kolesnikov - Post-graduate student, Department of «Theoretical Informatics and Computer Technologies», Bauman Moscow State Technical University
Abstract:
Most modern algorithms for congestion management in computer networks, such as overload alarm, back pressure, the deterrent package, are based on the statistics properties of the traffic [1]. Development and testing of these algorithms were based on the Poisson model and other recovery processes [2]. In such models one does not take into account the fact that the traffic of modern enterprise networks has periods of strong bursts of activity, or the so-called \"explosive\" character. Autocorrelation functions of such processes do not vanish at large time lags. In modern works it is increasingly indicated that heavy-traffic enterprise networks have self-similar behavior characterized with a distribution with heavy tail. A set of data streams that exhibit the properties of infinite variance leads to self-similarity. This process of data transfer differs from the Poisson process; short periods of increased activity could lead to a buffer overflow even at low average load in the network. A number of modern studies of traffic is devoted to the study of self-similar [3], fractal and chaotic properties of data flow in modern computer networks [4]. Such properties of the traffic were not taken into account in the development of algorithms to ensure QoS and congestion management. Thus, it becomes urgent to develop methods of traffic control, based on its properties and the methods of nonlinear dynamics and chaos theory. In particular, it is necessary to solve the problem of controlling the transmission channel and traffic at the router level, using short-term forecasting of the traffic load in a channel [5]. Such a technique is acceptable to use in conjunction with traffic shaping algorithms, such as the algorithm of markers basket. In this article, the analysis of the dynamic characteristics of incoming and outgoing network traffic server of the corporate network was performed. The calculation of correlation entropy as a measure of randomness of the process is considered. The correlation dimension of the data to estimate the allowable projection horizon is evaluated. Results of the analysis of the autocorrelation function and density distribution indicate the presence of a distribution with heavy tail. The numerical values of the Hurst exponent, as a quantitative measure of self-similarity of the time series are presented. On the basis of known data models and the forecast evaluated, the prediction accuracy of data is estimated. It is found that the fractal model of integrated autoregressive - moving average describes the behavior of self-similar processes and is suitable for time-series forecasting applications.
Pages: 18-31
References
- Stollings V.Sovremennye kompjuternye seti. SPb.: Piter. 2003.
- Tanenbaum EH.Kompjuternye seti. SPb.: Piter. 2003.
- Basarab M.A., Ivanov I.P., Kolesnikov A.V. Analiz setevogo trafika korporativnojj seti universiteta metodami nelinejjnojj dinamiki // Nauka i obrazovanie. EHlektronnoe nauchno-tekhnicheskoe izdanie. 2013. № 08.DOI: 10.7463/0813.0587054.
- Ivanov I.P., Basarab M.A., Kolesnikov A.V. Nelinejjno-dinamicheskie modeli setevogo trafika // Nelinejjnyjj mir. 2014. № 4. T.12.
- Kashin M.M. Razrabotka metoda upravlenija peregruzkami v setjakh SIP na osnove prognoza signalnogo trafika: Diss. kand. tekhn. nauk. Samara, 2011.
- Eliseeva I.I.EHkonometrika: uchebnik. M.: Finansy i statistika. 2002.
- Belkov D.V., Edemskaja E.N. Statisticheskijj analiz setevogo trafika. Doneckijjnacionalnyjjtekhnicheskijjuniversitet. 2011.
- Hurst H., Black R. Long-Term Storage: An Experimental Study. London: Constable. 1965.
- Hurst H. E. Long-Term Storage Capacity of Reservoirs // Transactions of the American Society of Civil Engineering. 1951. № 116. P. 770-799.
- Kirillov D.S., Korob O.V., Mitin N.A., Orlov Yu.N., Pleshakov R.V. Distribution of the Hurst Exponent of a Nonstationary Marked Time Series. Keldysh Institute of Applied Mathematics. Preprints. 2013. № 11.
- Teorija teletrafika: Uchebnoe posobie. Uljanovskijj Gosudarstvennyjj Tekhnicheskijj Universitet. 2006.
- Cano J.C., Manzoni P. On the use calculation of the Hurst parameter with MPEG videos data traffic. Valencia. 2000.
- Grassberger P., Procaccia I. Estimation of the Kolmogorov entropy from a chaotic signal. Phys. Rev. A 28. 1983.
- Sychev V.V. Vychislenie stokhasticheskikh kharakteristik fiziologicheskikh dannykh. Pushhino. 1999.
- Boks G., Dzhenkins G. Analiz vremennykh rjadov, prognoz i upravlenie. M.: Mir. 1974.
- Krjukov A.JU., CHernjagin D.V. ARIMA-model prognozirovanija znachenijj trafika // Informacionnye tekhnologii i vychislitelnye sistemy. 2001.
- Shim C., Ryoo I., Lee J., Lee S. Modeling and call admission control algorithm of variable bit rate video in ATM networks // IEEE journal on Selected Areas in Communications. 1993. V.3.P. 408 - 420.
- Cao L.J., Francis E.H. Tay. Support Vector Machine with Adaptive Parameters in Financial Time Series Forecasting. 2003.
- Fadeev I.V., Ivkin N.P. Avtoregressionnye algoritmy prognozirovanija, Mashinnoe obuchenie i analiz dannykh. 2011.
- Elsner J.B., Tsonis A.A. Singular Spectral Analysis. A new tool in the time series analysis. Plenum Press. 1996.
- Golyandina N., Nekrutkin V., Zhigljavsky A. Analysis of time series structure: SSA and related techniques. Chapman & Hall/CRC. 2001.
- Vautard R., Ghil M. Singular spectrum analysis in nonlinear dynamics, with applications to paleoclimatic time series // Physica D. 1989. V. 35. P. 395 - 424.
- Allen M.R., Smith L.A. Monte Carlo SSA: Detecting irregular oscillations in the presence of colored noise // Journal of Climate. 1996. V. 9. P.3373 - 3404.
- SHelukhin O.I.Multifraktaly. Infokommunikacionnye prilozhenija. M.: Gorjachaja Linija-Telekom. 2011.
- SHelukhin O.I., Osin A.V., Smolskijj S.M. Samopodobie i fraktaly. M.: FIZMALIT. 2008.
- Dingding Zhou, Songling Chen, Shi Dong. Network traffic prediction based on ARFIMA model. 2013.
- Grebennikov A. V., Krjukov JU. A. Modelirovanie setevogo trafika i prognozirovanie s pomoshhju modeli ARIMA // Sistemnyjj analiz v nauke i obrazovanii. 2011.